Jointly Gaussian random variables play an important role in probability theory, due partly to the fact that linear combinations of Gaussians are themselves Gaussian. This allows us to answer complex questions by only calculating means and variances. Here, we will explore an application of this phenomenon to the expected value. Let X₁,..., ✗n be independent Gaussian random variables, with expected values E[X¿] = μ and variances Var[X¿] σ² for i = 1,...,n and let 1 n = Y = Σ X; be their average. Intuitively, the random variable Y should get “closer" to μ as n i=1 the number of samples n increases. Below, we will try to make this intuition precise. 1 X1 (a) Define X = Determine its mean vector E[✗] and covariance matrix Σx. Xn (b) Express Y = AX for some matrix A. (Note that row vectors and column vectors are special cases of matrices.) (c) Determine E[Y]. (d) Determine Var[Y]. (e) Calculate the probability that Y is more than 8 > 0 away from its mean, P[|Y − E[Y]| > 6]. Express your answer in terms of the standard normal complementary CDF Q(z) = 1- Þ(z) or the standard normal. (f) Using the fact that Q(3.29) = 2000, calculate how many samples n are needed, as a function of the variance o² to guarantee that P[|Y - E[Y]| > 10] is 1000 or smaller.
Jointly Gaussian random variables play an important role in probability theory, due partly to the fact that linear combinations of Gaussians are themselves Gaussian. This allows us to answer complex questions by only calculating means and variances. Here, we will explore an application of this phenomenon to the expected value. Let X₁,..., ✗n be independent Gaussian random variables, with expected values E[X¿] = μ and variances Var[X¿] σ² for i = 1,...,n and let 1 n = Y = Σ X; be their average. Intuitively, the random variable Y should get “closer" to μ as n i=1 the number of samples n increases. Below, we will try to make this intuition precise. 1 X1 (a) Define X = Determine its mean vector E[✗] and covariance matrix Σx. Xn (b) Express Y = AX for some matrix A. (Note that row vectors and column vectors are special cases of matrices.) (c) Determine E[Y]. (d) Determine Var[Y]. (e) Calculate the probability that Y is more than 8 > 0 away from its mean, P[|Y − E[Y]| > 6]. Express your answer in terms of the standard normal complementary CDF Q(z) = 1- Þ(z) or the standard normal. (f) Using the fact that Q(3.29) = 2000, calculate how many samples n are needed, as a function of the variance o² to guarantee that P[|Y - E[Y]| > 10] is 1000 or smaller.
A First Course in Probability (10th Edition)
10th Edition
ISBN:9780134753119
Author:Sheldon Ross
Publisher:Sheldon Ross
Chapter1: Combinatorial Analysis
Section: Chapter Questions
Problem 1.1P: a. How many different 7-place license plates are possible if the first 2 places are for letters and...
Related questions
Question
Only c, d, e, f please. Thanks!
![Jointly Gaussian random variables play an important role in probability theory, due partly to
the fact that linear combinations of Gaussians are themselves Gaussian. This allows us to answer
complex questions by only calculating means and variances. Here, we will explore an application
of this phenomenon to the expected value. Let X₁,..., ✗n be independent Gaussian random
variables, with expected values E[X¿] = μ and variances Var[X¿]
σ² for i = 1,...,n and let
1
n
=
Y = Σ X; be their average. Intuitively, the random variable Y should get “closer" to μ as
n
i=1
the number of samples n increases. Below, we will try to make this intuition precise.
1
X1
(a) Define X =
Determine its mean vector E[✗] and covariance matrix Σx.
Xn
(b) Express Y = AX for some matrix A. (Note that row vectors and column vectors are special
cases of matrices.)
(c) Determine E[Y].
(d) Determine Var[Y].
(e) Calculate the probability that Y is more than 8 > 0 away from its mean, P[|Y − E[Y]| > 6].
Express your answer in terms of the standard normal complementary CDF Q(z) = 1- Þ(z)
or the standard normal.
(f) Using the fact that Q(3.29) = 2000, calculate how many samples n are needed, as a function
of the variance o² to guarantee that P[|Y - E[Y]| > 10] is 1000 or smaller.](/v2/_next/image?url=https%3A%2F%2Fcontent.bartleby.com%2Fqna-images%2Fquestion%2F02681530-b6ca-4d72-bfd1-7260256101ab%2F70ba3dcc-ba29-4b15-9189-416ee2bc3b7b%2Fznsakh_processed.png&w=3840&q=75)
Transcribed Image Text:Jointly Gaussian random variables play an important role in probability theory, due partly to
the fact that linear combinations of Gaussians are themselves Gaussian. This allows us to answer
complex questions by only calculating means and variances. Here, we will explore an application
of this phenomenon to the expected value. Let X₁,..., ✗n be independent Gaussian random
variables, with expected values E[X¿] = μ and variances Var[X¿]
σ² for i = 1,...,n and let
1
n
=
Y = Σ X; be their average. Intuitively, the random variable Y should get “closer" to μ as
n
i=1
the number of samples n increases. Below, we will try to make this intuition precise.
1
X1
(a) Define X =
Determine its mean vector E[✗] and covariance matrix Σx.
Xn
(b) Express Y = AX for some matrix A. (Note that row vectors and column vectors are special
cases of matrices.)
(c) Determine E[Y].
(d) Determine Var[Y].
(e) Calculate the probability that Y is more than 8 > 0 away from its mean, P[|Y − E[Y]| > 6].
Express your answer in terms of the standard normal complementary CDF Q(z) = 1- Þ(z)
or the standard normal.
(f) Using the fact that Q(3.29) = 2000, calculate how many samples n are needed, as a function
of the variance o² to guarantee that P[|Y - E[Y]| > 10] is 1000 or smaller.
Expert Solution

This question has been solved!
Explore an expertly crafted, step-by-step solution for a thorough understanding of key concepts.
This is a popular solution!
Trending now
This is a popular solution!
Step by step
Solved in 1 steps

Recommended textbooks for you
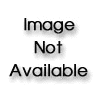
A First Course in Probability (10th Edition)
Probability
ISBN:
9780134753119
Author:
Sheldon Ross
Publisher:
PEARSON
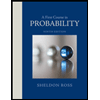
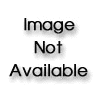
A First Course in Probability (10th Edition)
Probability
ISBN:
9780134753119
Author:
Sheldon Ross
Publisher:
PEARSON
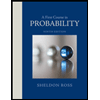