I've wrote a script to find the top three features for a random forest, but it is not work. please assist to fix the below code. #Import scikit-learn dataset library from sklearn import datasets #Load dataset iris = datasets.load_iris() # Creating a DataFrame of given iris dataset. import pandas as pd import numpy as np data=pd.DataFrame({ 'sepal length':iris.data[:,0], 'sepal width':iris.data[:,1], 'petal length':iris.data[:,2], 'petal width':iris.data[:,3], 'species':iris.target }) iris['target_names'] print(data.head()) # Import train_test_split function from sklearn.model_selection import train_test_split X=data[['sepal length', 'sepal width', 'petal length', 'petal width']] # Features y=data['species'] # Labels # Split dataset into training set and test set X_train, X_test, y_train, y_test = train_test_split(X, y, test_size=0.3) #Import Random Forest Model from sklearn.ensemble import RandomForestClassifier #Create a Gaussian Classifier rf=RandomForestClassifier(n_estimators=100) #Train the model using the training sets y_pred=rf.predict(X_test) rf.fit(X_train,y_train) y_pred=rf.predict(X_test) #Import scikit-learn metrics module for accuracy calculation from sklearn import metrics # Model Accuracy, how often is the classifier correct? print("Accuracy:", metrics.accuracy_score(y_test, y_pred)) # Predict species for a single flower. # sepal length = 3, sepal width = 5 # petal length = 4, petal width = 2 prediction = rf.predict([[3, 5, 4, 2]]) # 'setosa', 'versicolor', 'virginica' print(prediction) feature_list = ['sepal length', 'sepal width', 'petal length', 'petal width'] features = np.array(feature_list) # Get numerical feature importances importances = list(rf.feature_importances_) # List of tuples with variable and importance feature_importances = [(feature, round(importance, 2)) for feature, importance in zip(feature_list, importances)] # Sort the feature importances by most important first feature_importances = sorted(feature_importances, key = lambda x: x[1], reverse = True) # Print out the feature and importances [print('Variable: {:20} Importance: {}'.format(*pair)) for pair in feature_importances] from sklearn.ensemble import RandomForestRegressor rf_most_important = RandomForestRegressor(n_estimators= 1000, random_state=42) # Extract the two most important features important_indices = features train_important = X_train(:, important_indices) test_important = X_test(:, important_indices) # Train the random forest rf_most_important.fit(train_important, y_train) # Make predictions and determine the error predictions = rf_most_important.predict(test_important) errors = abs(predictions - test_labels)
I've wrote a script to find the top three features for a random forest, but it is not work. please assist to fix the below code. #Import scikit-learn dataset library from sklearn import datasets #Load dataset iris = datasets.load_iris() # Creating a DataFrame of given iris dataset. import pandas as pd import numpy as np data=pd.DataFrame({ 'sepal length':iris.data[:,0], 'sepal width':iris.data[:,1], 'petal length':iris.data[:,2], 'petal width':iris.data[:,3], 'species':iris.target }) iris['target_names'] print(data.head()) # Import train_test_split function from sklearn.model_selection import train_test_split X=data[['sepal length', 'sepal width', 'petal length', 'petal width']] # Features y=data['species'] # Labels # Split dataset into training set and test set X_train, X_test, y_train, y_test = train_test_split(X, y, test_size=0.3) #Import Random Forest Model from sklearn.ensemble import RandomForestClassifier #Create a Gaussian Classifier rf=RandomForestClassifier(n_estimators=100) #Train the model using the training sets y_pred=rf.predict(X_test) rf.fit(X_train,y_train) y_pred=rf.predict(X_test) #Import scikit-learn metrics module for accuracy calculation from sklearn import metrics # Model Accuracy, how often is the classifier correct? print("Accuracy:", metrics.accuracy_score(y_test, y_pred)) # Predict species for a single flower. # sepal length = 3, sepal width = 5 # petal length = 4, petal width = 2 prediction = rf.predict([[3, 5, 4, 2]]) # 'setosa', 'versicolor', 'virginica' print(prediction) feature_list = ['sepal length', 'sepal width', 'petal length', 'petal width'] features = np.array(feature_list) # Get numerical feature importances importances = list(rf.feature_importances_) # List of tuples with variable and importance feature_importances = [(feature, round(importance, 2)) for feature, importance in zip(feature_list, importances)] # Sort the feature importances by most important first feature_importances = sorted(feature_importances, key = lambda x: x[1], reverse = True) # Print out the feature and importances [print('Variable: {:20} Importance: {}'.format(*pair)) for pair in feature_importances] from sklearn.ensemble import RandomForestRegressor rf_most_important = RandomForestRegressor(n_estimators= 1000, random_state=42) # Extract the two most important features important_indices = features train_important = X_train(:, important_indices) test_important = X_test(:, important_indices) # Train the random forest rf_most_important.fit(train_important, y_train) # Make predictions and determine the error predictions = rf_most_important.predict(test_important) errors = abs(predictions - test_labels)
Database System Concepts
7th Edition
ISBN:9780078022159
Author:Abraham Silberschatz Professor, Henry F. Korth, S. Sudarshan
Publisher:Abraham Silberschatz Professor, Henry F. Korth, S. Sudarshan
Chapter1: Introduction
Section: Chapter Questions
Problem 1PE
Related questions
Question
100%
I've wrote a script to find the top three features for a random forest, but it is not work.
please assist to fix the below code.
#Import scikit-learn dataset library
from sklearn import datasets
#Load dataset
iris = datasets.load_iris()
# Creating a DataFrame of given iris dataset.
import pandas as pd
import numpy as np
data=pd.DataFrame({
'sepal length':iris.data[:,0],
'sepal width':iris.data[:,1],
'petal length':iris.data[:,2],
'petal width':iris.data[:,3],
'species':iris.target
})
iris['target_names']
print(data.head())
# Import train_test_split function
from sklearn.model_selection import train_test_split
X=data[['sepal length', 'sepal width', 'petal length', 'petal width']] # Features
y=data['species'] # Labels
# Split dataset into training set and test set
X_train, X_test, y_train, y_test = train_test_split(X, y, test_size=0.3)
#Import Random Forest Model
from sklearn.ensemble import RandomForestClassifier
#Create a Gaussian Classifier
rf=RandomForestClassifier(n_estimators=100)
#Train the model using the training sets y_pred=rf.predict(X_test)
rf.fit(X_train,y_train)
y_pred=rf.predict(X_test)
#Import scikit-learn metrics module for accuracy calculation
from sklearn import metrics
# Model Accuracy, how often is the classifier correct?
print("Accuracy:", metrics.accuracy_score(y_test, y_pred))
# Predict species for a single flower.
# sepal length = 3, sepal width = 5
# petal length = 4, petal width = 2
prediction = rf.predict([[3, 5, 4, 2]])
# 'setosa', 'versicolor', 'virginica'
print(prediction)
feature_list = ['sepal length', 'sepal width', 'petal length', 'petal width']
features = np.array(feature_list)
# Get numerical feature importances
importances = list(rf.feature_importances_)
# List of tuples with variable and importance
feature_importances = [(feature, round(importance, 2)) for feature, importance in zip(feature_list, importances)]
# Sort the feature importances by most important first
feature_importances = sorted(feature_importances, key = lambda x: x[1], reverse = True)
# Print out the feature and importances
[print('Variable: {:20} Importance: {}'.format(*pair)) for pair in feature_importances]
from sklearn.ensemble import RandomForestRegressor
rf_most_important = RandomForestRegressor(n_estimators= 1000, random_state=42)
# Extract the two most important features
important_indices = features
train_important = X_train(:, important_indices)
test_important = X_test(:, important_indices)
# Train the random forest
rf_most_important.fit(train_important, y_train)
# Make predictions and determine the error
predictions = rf_most_important.predict(test_important)
errors = abs(predictions - test_labels)
from sklearn import datasets
#Load dataset
iris = datasets.load_iris()
# Creating a DataFrame of given iris dataset.
import pandas as pd
import numpy as np
data=pd.DataFrame({
'sepal length':iris.data[:,0],
'sepal width':iris.data[:,1],
'petal length':iris.data[:,2],
'petal width':iris.data[:,3],
'species':iris.target
})
iris['target_names']
print(data.head())
# Import train_test_split function
from sklearn.model_selection import train_test_split
X=data[['sepal length', 'sepal width', 'petal length', 'petal width']] # Features
y=data['species'] # Labels
# Split dataset into training set and test set
X_train, X_test, y_train, y_test = train_test_split(X, y, test_size=0.3)
#Import Random Forest Model
from sklearn.ensemble import RandomForestClassifier
#Create a Gaussian Classifier
rf=RandomForestClassifier(n_estimators=100)
#Train the model using the training sets y_pred=rf.predict(X_test)
rf.fit(X_train,y_train)
y_pred=rf.predict(X_test)
#Import scikit-learn metrics module for accuracy calculation
from sklearn import metrics
# Model Accuracy, how often is the classifier correct?
print("Accuracy:", metrics.accuracy_score(y_test, y_pred))
# Predict species for a single flower.
# sepal length = 3, sepal width = 5
# petal length = 4, petal width = 2
prediction = rf.predict([[3, 5, 4, 2]])
# 'setosa', 'versicolor', 'virginica'
print(prediction)
feature_list = ['sepal length', 'sepal width', 'petal length', 'petal width']
features = np.array(feature_list)
# Get numerical feature importances
importances = list(rf.feature_importances_)
# List of tuples with variable and importance
feature_importances = [(feature, round(importance, 2)) for feature, importance in zip(feature_list, importances)]
# Sort the feature importances by most important first
feature_importances = sorted(feature_importances, key = lambda x: x[1], reverse = True)
# Print out the feature and importances
[print('Variable: {:20} Importance: {}'.format(*pair)) for pair in feature_importances]
from sklearn.ensemble import RandomForestRegressor
rf_most_important = RandomForestRegressor(n_estimators= 1000, random_state=42)
# Extract the two most important features
important_indices = features
train_important = X_train(:, important_indices)
test_important = X_test(:, important_indices)
# Train the random forest
rf_most_important.fit(train_important, y_train)
# Make predictions and determine the error
predictions = rf_most_important.predict(test_important)
errors = abs(predictions - test_labels)
Expert Solution

This question has been solved!
Explore an expertly crafted, step-by-step solution for a thorough understanding of key concepts.
This is a popular solution!
Trending now
This is a popular solution!
Step by step
Solved in 4 steps with 4 images

Knowledge Booster
Learn more about
Need a deep-dive on the concept behind this application? Look no further. Learn more about this topic, computer-science and related others by exploring similar questions and additional content below.Recommended textbooks for you
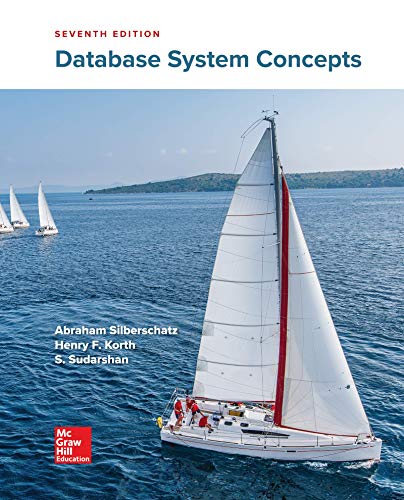
Database System Concepts
Computer Science
ISBN:
9780078022159
Author:
Abraham Silberschatz Professor, Henry F. Korth, S. Sudarshan
Publisher:
McGraw-Hill Education
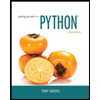
Starting Out with Python (4th Edition)
Computer Science
ISBN:
9780134444321
Author:
Tony Gaddis
Publisher:
PEARSON
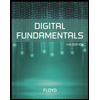
Digital Fundamentals (11th Edition)
Computer Science
ISBN:
9780132737968
Author:
Thomas L. Floyd
Publisher:
PEARSON
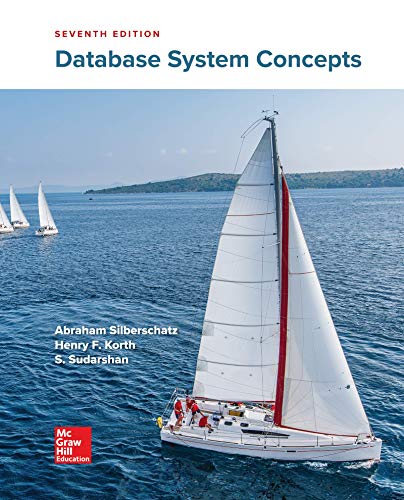
Database System Concepts
Computer Science
ISBN:
9780078022159
Author:
Abraham Silberschatz Professor, Henry F. Korth, S. Sudarshan
Publisher:
McGraw-Hill Education
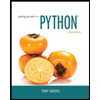
Starting Out with Python (4th Edition)
Computer Science
ISBN:
9780134444321
Author:
Tony Gaddis
Publisher:
PEARSON
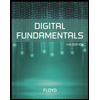
Digital Fundamentals (11th Edition)
Computer Science
ISBN:
9780132737968
Author:
Thomas L. Floyd
Publisher:
PEARSON
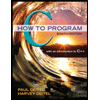
C How to Program (8th Edition)
Computer Science
ISBN:
9780133976892
Author:
Paul J. Deitel, Harvey Deitel
Publisher:
PEARSON
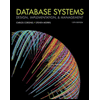
Database Systems: Design, Implementation, & Manag…
Computer Science
ISBN:
9781337627900
Author:
Carlos Coronel, Steven Morris
Publisher:
Cengage Learning
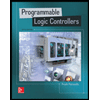
Programmable Logic Controllers
Computer Science
ISBN:
9780073373843
Author:
Frank D. Petruzella
Publisher:
McGraw-Hill Education