is this code correct for gradient descent for two vectors (two dimensions)? **************************************** import numpy as np from matplotlib import pyplot as plt class GradientDescent: def __init__(self, function, gradient, initial_solution, learning_rate=0.1, max_iter=100, tolerance=0.0000001): self.function = function self.gradient = gradient self.solution = initial_solution self.learning_rate = learning_rate self.max_iter = max_iter
is this code correct for gradient descent for two
****************************************
import numpy as np
from matplotlib import pyplot as plt
class GradientDescent:
def __init__(self, function, gradient, initial_solution, learning_rate=0.1, max_iter=100, tolerance=0.0000001):
self.function = function
self.gradient = gradient
self.solution = initial_solution
self.learning_rate = learning_rate
self.max_iter = max_iter
self.tolerance = tolerance
def run(self):
t = 0
while t < self.max_iter:
diff = -self.learning_rate * self.gradient(*self.solution)
if np.linalg.norm(diff) < self.tolerance:
break
self.solution = tuple([self.solution[i] + diff[i] for i in range(len(diff))])
t += 1
return self.solution, self.function(*self.solution)
def fun1(x, y):
return x ** 2 + y ** 2
def gradient1(x, y):
return np.array([2 * x, 2 * y])
bounds = [-3, 3]
plt.figure()
x, y = np.meshgrid(np.linspace(bounds[0], bounds[1], 100), np.linspace(bounds[0], bounds[1], 100))
z = fun1(x, y)
plt.contour(x, y, z,levels=20)
random_solution = np.random.uniform(bounds[0], bounds[1], size=2)
gd = GradientDescent(fun1, gradient1, random_solution)
best_solution, best_value = gd.run()
plt.plot(best_solution[0], best_solution[1])

Step by step
Solved in 3 steps

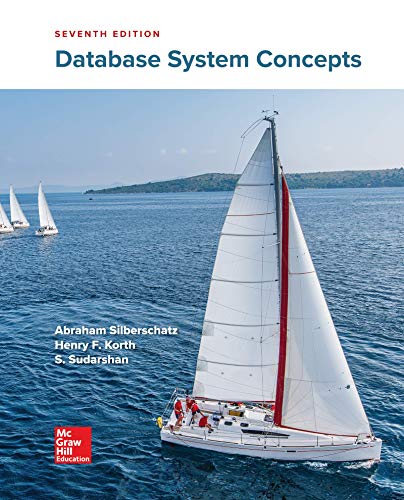
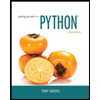
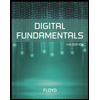
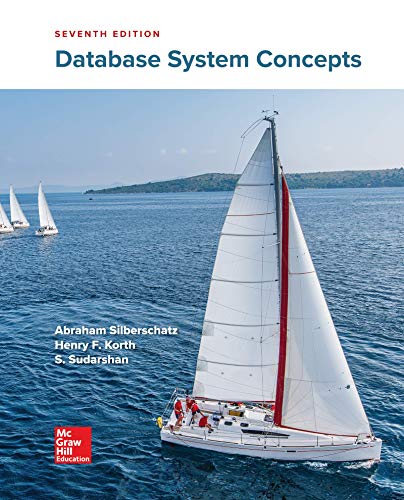
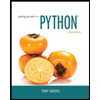
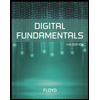
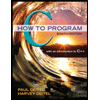
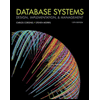
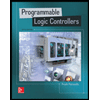