In this scenario, you would activate early stopping after the 7th epoch because the error in the 6th epoch (0.5677) was higher than the best error observed so far (0.3245), and then the error in the 7th epoch (0.6798) was also higher than the best error, indicating a consecutive increase for two epochs
When implementing early stopping, the validation error is typically compared to the best validation error observed so far. In your specific case, where you tolerate an increase in error for two consecutive epochs before activating early stopping, you would compare the validation error for each epoch to the best validation error recorded.
For example, if you have validation errors (0.5643, 0.6785, 0.3245, 0.4536, 0.4476, 0.5677, 0.6798), you would track the best validation error (initially set to a high value) and compare each new validation error to it.
In this scenario, you would activate early stopping after the 7th epoch because the error in the 6th epoch (0.5677) was higher than the best error observed so far (0.3245), and then the error in the 7th epoch (0.6798) was also higher than the best error, indicating a consecutive increase for two epochs.

The question asks you to explain the concept of early stopping in machine learning. Specifically, it asks you to describe how early stopping is implemented when you tolerate an increase in error for two consecutive epochs before stopping, using a provided example to illustrate the process
Step by step
Solved in 3 steps

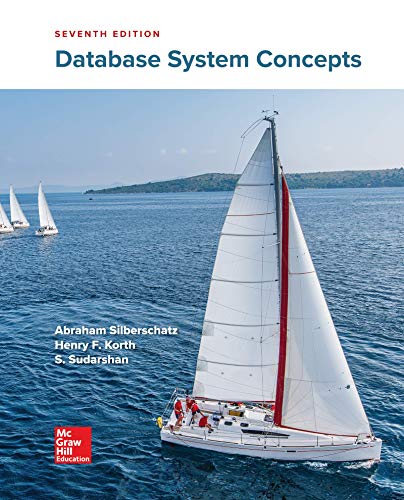
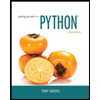
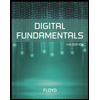
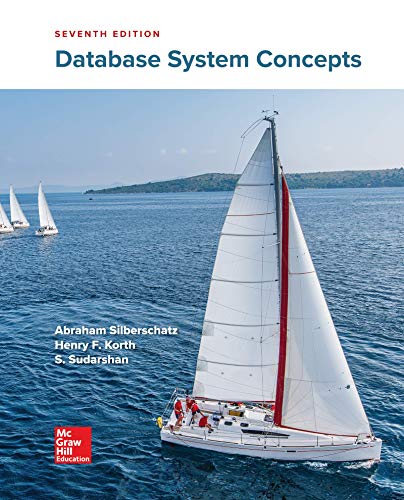
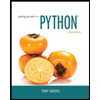
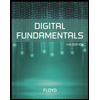
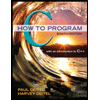
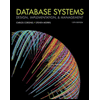
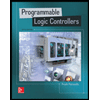