In a regression model involving 44 observations, the following estimated regression equation was obtained. For this model Model SS = 600 and Residual SS = 400. The computed F statistics for testing the overall significance of the above model is
Correlation
Correlation defines a relationship between two independent variables. It tells the degree to which variables move in relation to each other. When two sets of data are related to each other, there is a correlation between them.
Linear Correlation
A correlation is used to determine the relationships between numerical and categorical variables. In other words, it is an indicator of how things are connected to one another. The correlation analysis is the study of how variables are related.
Regression Analysis
Regression analysis is a statistical method in which it estimates the relationship between a dependent variable and one or more independent variable. In simple terms dependent variable is called as outcome variable and independent variable is called as predictors. Regression analysis is one of the methods to find the trends in data. The independent variable used in Regression analysis is named Predictor variable. It offers data of an associated dependent variable regarding a particular outcome.
In a regression model involving 44 observations, the following estimated regression equation was obtained.
For this model Model SS = 600 and Residual SS = 400.
The computed F statistics for testing the overall significance of the above model is
![### Multiple Linear Regression Model
In statistics and machine learning, a Multiple Linear Regression (MLR) model represents a linear approximation of the relationship between a dependent variable (often denoted as \( \hat{y} \)) and multiple independent variables (\( x_1, x_2, x_3, \ldots \)). The general form of an MLR equation is:
\[ \hat{y} = \beta_0 + \beta_1 x_1 + \beta_2 x_2 + \ldots + \beta_n x_n \]
where:
- \( \hat{y} \) is the predicted value of the dependent variable.
- \( \beta_0 \) is the y-intercept (constant term).
- \( \beta_1, \beta_2, \ldots, \beta_n \) are the coefficients for the independent variables \( x_1, x_2, \ldots, x_n \).
In the provided image, we have a specific Multiple Linear Regression model equation:
\[ \hat{y} = 29 + 18x_1 + 43x_2 + 87x_3 \]
Here:
- \( \hat{y} \) is the predicted value.
- \( 29 \) is the constant term or intercept.
- \( 18 \) is the coefficient for the independent variable \( x_1 \).
- \( 43 \) is the coefficient for the independent variable \( x_2 \).
- \( 87 \) is the coefficient for the independent variable \( x_3 \).
This equation indicates that the prediction \( \hat{y} \) increases by 18 units for each unit increase in \( x_1 \), by 43 units for each unit increase in \( x_2 \), and by 87 units for each unit increase in \( x_3 \), starting from a base value of 29 when all \( x \) variables are zero.](/v2/_next/image?url=https%3A%2F%2Fcontent.bartleby.com%2Fqna-images%2Fquestion%2F45c9d9ea-9d85-4e17-bbcb-fa0288237f89%2F42a2e963-c2ff-4c3b-98dc-69b8ee236a61%2Fjwdmw7b.png&w=3840&q=75)

Trending now
This is a popular solution!
Step by step
Solved in 3 steps with 3 images

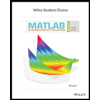
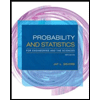
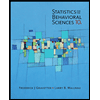
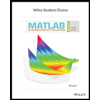
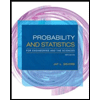
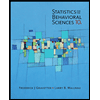
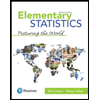
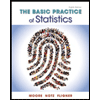
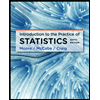