Imagine an agent like the one in the interactive demo. This agent has a value function like the following, and always chooses the action with the highest value: Q(s=near berry with empty stomach, a=eat berry) = 15 Q(s=near berry with empty stomach, a=turn around) = -4 Q(s=near berry with berry in stomach, a=eat berry) = 8 Q(s=near berry with berry in stomach, a=turn around) = 4 Q(s=not near berry with empty stomach, a=look for berry) = 1 I am taking cognitive science, and I have come across this question regarding Q-Learning. I don't understand the question nor do I understand how to do it. Can anyone help? Here is the question below: The agent's eyes detect two berries in front of the agent. Each berry is one step away from the agent (one berry is to the northwest of the agent, the other berry is to the northeast of the agent). The agent eats one of the berries, and experiences a reward of 9. The agent then updates its value function based on this experience using Q-Learning. If the agent has a gamma of 0.5 and an alpha of 0.1, using the Q-learning equation below, select the correct updated value for Q(s=near berry with empty stomach, a=eat berry
Imagine an agent like the one in the interactive demo.
This agent has a value function like the following, and always chooses the action with the highest value:
Q(s=near berry with empty stomach, a=eat berry) = 15
Q(s=near berry with empty stomach, a=turn around) = -4
Q(s=near berry with berry in stomach, a=eat berry) = 8
Q(s=near berry with berry in stomach, a=turn around) = 4
Q(s=not near berry with empty stomach, a=look for berry) = 1
I am taking cognitive science, and I have come across this question regarding Q-Learning. I don't understand the question nor do I understand how to do it. Can anyone help? Here is the question below:
The agent's eyes detect two berries in front of the agent. Each berry is one step away from the agent (one berry is to the northwest of the agent, the other berry is to the northeast of the agent). The agent eats one of the berries, and experiences a reward of 9. The agent then updates its value function based on this experience using Q-Learning.
If the agent has a gamma of 0.5 and an alpha of 0.1, using the Q-learning equation below, select the correct updated value for
Q(s=near berry with empty stomach, a=eat berry)
![Q(st, at) + Q(8t, at) + a x [rt + [y × maxat+1'
Q(st+1;a4+1)] – Q(st, at)]](/v2/_next/image?url=https%3A%2F%2Fcontent.bartleby.com%2Fqna-images%2Fquestion%2Fd099b6de-2289-4e49-863e-11931e044b60%2Fe83f7733-76e5-44e4-b14c-6f0af77b6d24%2F1jz9tzh_processed.jpeg&w=3840&q=75)

Trending now
This is a popular solution!
Step by step
Solved in 2 steps

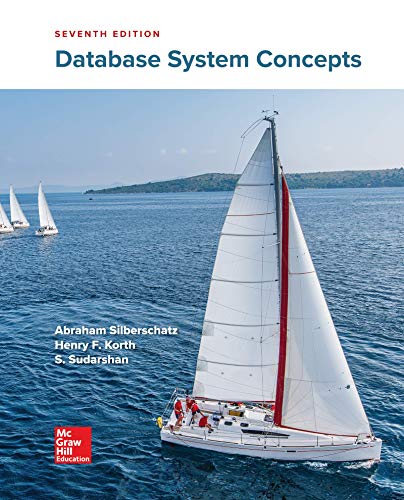
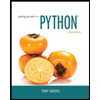
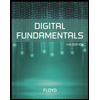
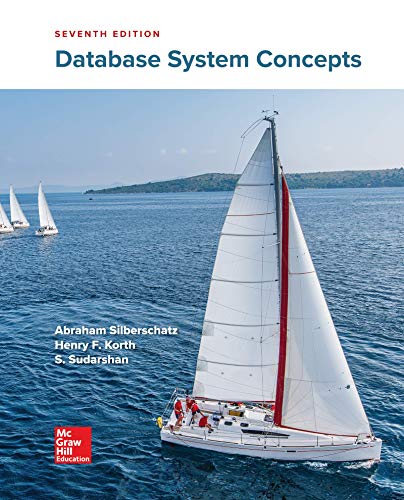
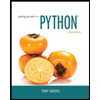
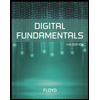
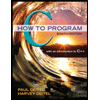
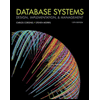
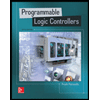