Identify which of the variables is most likely to be the explanatory variable, and which is most likely to be the response variable. Explain. Identify whether each variable is categorical or quantitative, and classify the relationship as C→Q, C→C, or Q→Q, and explain how you know. Determine what type of data display and description is appropriate for the relationship, and explain why. Create an appropriate data display and describe the relationship using vocabulary appropriate to the type of data. Describe any potential lurking (confounding) variables and describe how this impacts association (correlation) versus causation. Describe what other information you can get from this data, or what other questions you might have about it.
Identify which of the variables is most likely to be the explanatory variable, and which is most likely to be the response variable. Explain. Identify whether each variable is categorical or quantitative, and classify the relationship as C→Q, C→C, or Q→Q, and explain how you know. Determine what type of data display and description is appropriate for the relationship, and explain why. Create an appropriate data display and describe the relationship using vocabulary appropriate to the type of data. Describe any potential lurking (confounding) variables and describe how this impacts association (correlation) versus causation. Describe what other information you can get from this data, or what other questions you might have about it.
MATLAB: An Introduction with Applications
6th Edition
ISBN:9781119256830
Author:Amos Gilat
Publisher:Amos Gilat
Chapter1: Starting With Matlab
Section: Chapter Questions
Problem 1P
Related questions
Question

Transcribed Image Text:### Study on Children's Height and Reading Level
1. **Overview of Study Findings**
A study comparing children's height to their reading level showed a notable correlation. Specifically, the study found that:
- **70% of children who were less than 48 inches tall** had a reading level at or below 4th grade.
- In contrast, **80% of children who were 48 inches or taller** had a reading level above 4th grade.
2. **Sample Size and Height Distribution**
The study included a total of **200 students**. Among these students, **110 were less than 48 inches tall**.
This correlation indicates that there may be a positive relationship between height and reading levels among the children studied. Further research could explore the underlying factors contributing to this trend, such as age, nutritional status, or socioeconomic background.

Transcribed Image Text:### Analyzing Relationships Between Variables
1. **Identify the Variables**
- **Explanatory Variable**: Determine the variable which is likely causing an effect.
- **Response Variable**: Identify the variable that is being influenced or changed.
- **Explanation**: Explain the reasoning behind your choices.
2. **Classify the Variables**
- **Categorical vs Quantitative**: Determine if each variable is categorical (C) or quantitative (Q).
- **Relationship Type**:
- **C → Q**: Categorical to Quantitative
- **C → C**: Categorical to Categorical
- **Q → Q**: Quantitative to Quantitative
- **Explanation**: Justify how you classified the relationship.
3. **Data Display and Description**
- **Appropriate Data Display**: Choose a suitable way to display the data (e.g., bar graph, scatter plot) based on the relationship.
- **Explanation**: Explain why this display method is appropriate for visualizing the relationship.
4. **Create and Describe a Data Display**
- **Data Visualization**: Create a visualization that accurately represents the data.
- **Description**: Use appropriate terminology to describe the relationship shown in the display.
5. **Identify Lurking Variables**
- **Confounding Variables**: Describe any variables that might influence both the explanatory and response variables.
- **Impact on Analysis**: Discuss how these variables might affect the perceived correlation or causation.
- **Further Questions**: Outline additional questions or information that could be explored to deepen the analysis.
### Example Questions to Guide the Analysis
- What patterns can be identified in the data?
- Are there any noticeable trends or outliers?
- How strong is the apparent association between the variables?
- What could be the real-world implications of the findings?
By following these steps, you can systematically analyze and interpret relationships between different types of variables, ensuring a thorough and insightful examination of the data.
Expert Solution

This question has been solved!
Explore an expertly crafted, step-by-step solution for a thorough understanding of key concepts.
Step by step
Solved in 3 steps

Recommended textbooks for you
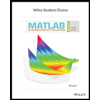
MATLAB: An Introduction with Applications
Statistics
ISBN:
9781119256830
Author:
Amos Gilat
Publisher:
John Wiley & Sons Inc
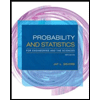
Probability and Statistics for Engineering and th…
Statistics
ISBN:
9781305251809
Author:
Jay L. Devore
Publisher:
Cengage Learning
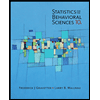
Statistics for The Behavioral Sciences (MindTap C…
Statistics
ISBN:
9781305504912
Author:
Frederick J Gravetter, Larry B. Wallnau
Publisher:
Cengage Learning
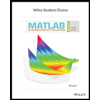
MATLAB: An Introduction with Applications
Statistics
ISBN:
9781119256830
Author:
Amos Gilat
Publisher:
John Wiley & Sons Inc
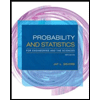
Probability and Statistics for Engineering and th…
Statistics
ISBN:
9781305251809
Author:
Jay L. Devore
Publisher:
Cengage Learning
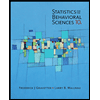
Statistics for The Behavioral Sciences (MindTap C…
Statistics
ISBN:
9781305504912
Author:
Frederick J Gravetter, Larry B. Wallnau
Publisher:
Cengage Learning
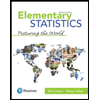
Elementary Statistics: Picturing the World (7th E…
Statistics
ISBN:
9780134683416
Author:
Ron Larson, Betsy Farber
Publisher:
PEARSON
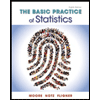
The Basic Practice of Statistics
Statistics
ISBN:
9781319042578
Author:
David S. Moore, William I. Notz, Michael A. Fligner
Publisher:
W. H. Freeman
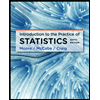
Introduction to the Practice of Statistics
Statistics
ISBN:
9781319013387
Author:
David S. Moore, George P. McCabe, Bruce A. Craig
Publisher:
W. H. Freeman