i. Define the independent and dependent variables in this problem. ii. Develop the equation of the simple linear regression line to predict the numberm of cases and delivery time. iii. Define and interpret the coefficients of determination and correlation.
Correlation
Correlation defines a relationship between two independent variables. It tells the degree to which variables move in relation to each other. When two sets of data are related to each other, there is a correlation between them.
Linear Correlation
A correlation is used to determine the relationships between numerical and categorical variables. In other words, it is an indicator of how things are connected to one another. The correlation analysis is the study of how variables are related.
Regression Analysis
Regression analysis is a statistical method in which it estimates the relationship between a dependent variable and one or more independent variable. In simple terms dependent variable is called as outcome variable and independent variable is called as predictors. Regression analysis is one of the methods to find the trends in data. The independent variable used in Regression analysis is named Predictor variable. It offers data of an associated dependent variable regarding a particular outcome.
Management of a soft-drink bottling company has the business objective of developing a method for allocating delivery costs to customers. Although one cost clearly relates to travel time within a particular route, another variable cost reflects the time required to unload the cases of soft drink at the delivery point. To begin, management decided to develop a regression model to predict delivery time based on the number of cases delivered. A sample of 20 deliveries within a territory was selected. The delivery times and the number of cases delivered were organized in the following table:
CUSTOMER |
NUMBER OF CASES |
DELIVERY TIME (MINUTS) |
1 |
52 |
32.1 |
2 |
64 |
34.8 |
3 |
73 |
36.2 |
4 |
85 |
37.8 |
5 |
95 |
37.8 |
6 |
103 |
39.7 |
7 |
116 |
38.5 |
8 |
121 |
41.9 |
9 |
143 |
44.2 |
10 |
157 |
47.1 |
11 |
161 |
43.0 |
12 |
184 |
49.4 |
13 |
202 |
57.2 |
14 |
218 |
56.8 |
15 |
243 |
60.6 |
16 |
254 |
61.2 |
17 |
267 |
58.2 |
18 |
275 |
63.1 |
19 |
287 |
65.6 |
20 |
298 |
67.3 |
output
Model Summary
model | R | R square | Adjusted R Square |
Std. Error of the Estimate |
1 | .986 | .972 | .970 | 13.98483 |
a. Predictors: (Constant), Time
ANOVA
model | sum of square | df | mean square | F | SIG |
1regression Residual total |
1211099.442 3520.358 124619.800 |
1 18 19 |
121099.442 195.575
|
619.196
|
.000
|
a. Dependent Variable: Cases
Coefficients
model |
Unstandardized Coefficients B |
Unstandardized Coefficients Std.error | Standardized Coefficients Beta |
t | sig |
1(constant) Time |
-167.547 6.940 |
13.917 .279 |
.986 |
-12.039 24.884 |
.000 .000 |
a. Dependent Variable: Cases
i. Define the independent and dependent variables in this problem.
ii. Develop the equation of the simple linear regression line to predict the numberm of cases and delivery time.
iii. Define and interpret the coefficients of determination and correlation.
iv. Test the significance of the model at 0.05

Trending now
This is a popular solution!
Step by step
Solved in 2 steps

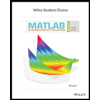
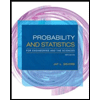
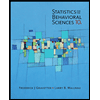
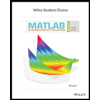
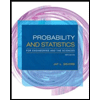
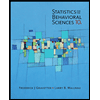
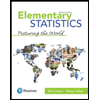
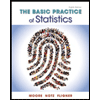
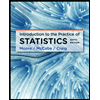