Hello, I have issues getting my Decision tree code working utilizing a Utility class similar to the following. I am having a recursion issue towards the bottom of the learn function, with the self.tree['left'].learn( line and the following line. I need the code to fill out the decision tree and not hit a recursive state. My PYTHON code is as follows:
Hello, I have issues getting my Decision tree code working utilizing a Utility class similar to the following. I am having a recursion issue towards the bottom of the learn function, with the self.tree['left'].learn( line and the following line. I need the code to fill out the decision tree and not hit a recursive state. My PYTHON code is as follows:
class DecisionTree(object):
def __init__(self, max_depth):
# Initializing the tree as an empty dictionary or list, as preferred
self.tree = {}
self.max_depth = max_depth
def learn(self, X, y, par_node = {}, depth=0):
k = 0
label_start = y[0]
num_labels = len(y)
for i in range(0, num_labels):
if y[i] != label_start:
k = 1
if k == 0:
self.tree['class'] = 'child'
self.tree['label'] = label_start
return
split_attribute, split_value, X_left, X_right, y_left, y_right = Utility.best_split(self,X, y)
self.tree['split_attribute'] = split_attribute
self.tree['split_value'] = split_value
self.tree['class'] = 'parent'
self.tree['left'] = DecisionTree(self.max_depth)
self.tree['right'] = DecisionTree(self.max_depth)
par_node = self.tree
self.tree['left'].learn(X_left, y_left, par_node, depth+1)
self.tree['right'].learn(X_right, y_right, par_node, depth+1)
if depth == self.max_depth:
return
#############################################
def classify(self, record):
#############################################
tree_temp = self.tree
while tree_temp['class'] == 'parent':
if isinstance(record[tree_temp['split_attribute']],slice):
if record[tree_temp['split_attribute']] == tree_temp['split_value']:
tree_temp = tree_temp['left'].tree
else:
tree_temp = tree_temp['right'].tree
else:
if record[tree_temp['split_attribute']] < tree_temp['split_value']:
tree_temp = tree_temp['left'].tree
else:
tree_temp = tree_temp['right'].tree
return tree_temp['label']
#############################################
def best_split(self,X,y):
split_attribute = 0
split_val = 0
X_left, X_right, y_left, y_right = [], [], [], []
info_gain_current = -1
for i in range(0, len(X[0])):
split_values = []
for j in range(len(X)):
split_values.append(X[j][i])
if isinstance(X[0][i], slice):
split_mean = X[0][i]
else:
split_mean = np.mean(split_values)
t_X_left, t_X_right, t_y_left, t_y_right = Utility.partition_classes(self,X, y, i, split_mean)
info_gain = Utility.information_gain(self,y, [t_y_left, t_y_right])
if info_gain >= info_gain_current:
split_attribute = i
split_val = split_mean
info_gain_current = info_gain
X_left = t_X_left
X_right = t_X_right
y_left = t_y_left
y_right = t_y_right
return (split_attribute, split_val, X_left, X_right, y_left, y_right)

Trending now
This is a popular solution!
Step by step
Solved in 2 steps

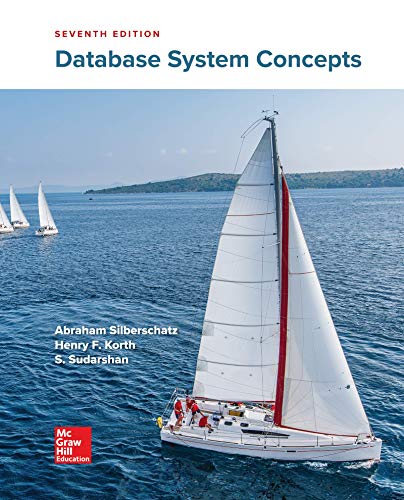
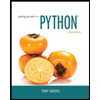
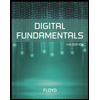
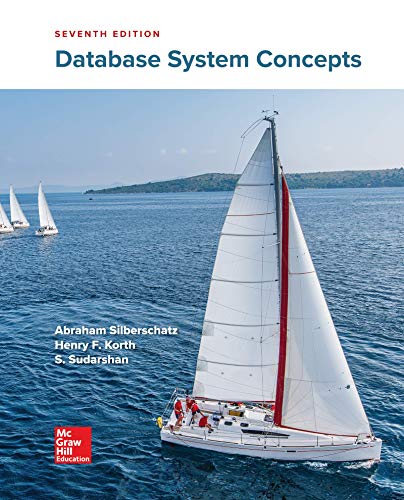
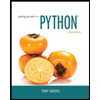
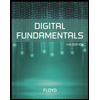
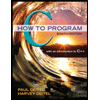
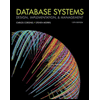
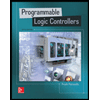