height weight female eyes age uhat yhat uuu 74 195 0 1 53 -7.384037 202.384 3.74359 63 120 1 4 33 3.288961 116.711 -1.909091 65 120 1 4 22 -12.28795 132.2879 .0909091 75 210 0 1 44 -.1724911 210.1725 4.74359 66 130 1 3 29 -10.0764 140.0764 1.090909 62 150 1 1 37 41.07742 108.9226 -2.909091 69 230 1 1 34 66.55824 163.4418 4.090909 73 180 0 5 27 -14.59558 194.5956 2.74359 65 170 0 3 47 37.71205 132.2879 -5.25641 72 195 0 2 33 8.192872 186.8071 1.74359 68 120 1 1 25 -35.65331 155.6533 3.090909 70 180 0 5 32 8.769781 171.2302 -.2564103 66 164 0 4 25 23.9236 140.0764 -4.25641 70 190 0 4 24 18.76978 171.2302 -.2564103 63 120 1 3 22 3.288961 116.711 -1.909091 79 225 0 1 21 -16.32631 241.3263 8.743589 71 212 0 1 48 32.98133 179.0187 .7435898 69 195 0 1 27 31.55824 163.4418 -1.25641 74 195 0 1 53 -7.384037 202.384 3.74359 71 190 0 1 30 10.98133 179.0187 .7435898 64 119 1 4 22 -5.499493 124.4995 -.9090909 64 119 1 4 22 -5.499493 124.4995 -.9090909 66 123 0 4 23 -17.0764 140.0764 -4.25641 70 145 0 5 27 -26.23022 171.2302 -.2564103 68 185 0 1 47 29.34669 155.6533 -2.25641 65 112 1 1 23 -20.28795 132.2879 .0909091 72 190 0 3 27 3.192872 186.8071 1.74359 69 165 0 1 36 1.558235 163.4418 -1.25641 65 100 1 1 23 -32.28795 132.2879 .0909091 61 95 1 1 22 -6.13413 101.1341 -3.909091 70 145 0 1 23 -26.23022 171.2302 -.2564103 70 155 0 1 45 -16.23022 171.2302 -.2564103 66 115 1 1 24 -25.0764 140.0764 1.090909 72 190 0 3 24 3.192872 186.8071 1.74359 70 135 1 3 26 -36.23022 171.2302 5.090909 62 115 1 3 26 6.077415 108.9226 -2.909091 71 165 0 3 26 -14.01867 179.0187 .7435898 74 225 0 1 22 22.61596 202.384 3.74359 64 106 1 1 25 -18.49949 124.4995 -.9090909 71 200 0 1 25 20.98133 179.0187 .7435898 68 134 1 3 23 -21.65331 155.6533 3.090909 67 161 0 1 23 13.13514 147.8649 -3.25641 69 165 0 4 22 1.558235 163.4418 -1.25641 65 110 1 1 23 -22.28795 132.2879 .0909091 68 137 1 3 25 -18.65331 155.6533 3.090909 74 207 0 1 52 4.615963 202.384 3.74359 63 101 1 4 23 -15.71104 116.711 -1.909091 65 125 1 1 26 -7.287948 132.2879 .0909091 64 170 1 1 26 45.50051 124.4995 -.9090909 70 195 0 5 23 23.76978 171.2302 -.2564103 64 120 1 2 23 -4.499493 124.4995 -.9090909 70 240 0 2 26 68.76978 171.2302 -.2564103 64 106 1 1 25 -18.49949 124.4995 -.9090909 67 120 0 1 26 -27.86486 147.8649 -3.25641 67 120 1 4 26 -27.86486 147.8649 2.090909 67 161 0 1 23 13.13514 147.8649 -3.25641 66 110 0 4 23 -30.0764 140.0764 -4.25641 72 205 0 5 26 18.19287 186.8071 1.74359 71 200 0 1 25 20.98133 179.0187 .7435898 71 165 0 3 26 -14.01867 179.0187 .7435898 70 170 0 1 26 -1.230219 171.2302 -.2564103 71 167 0 1 23 -12.01867 179.0187 .7435898 64 118 1 4 23 -6.499493 124.4995 -.9090909 65 122 1 1 26 -10.28795 132.2879 .0909091 67 140 0 1 25 -7.864856 147.8649 -3.25641 66 190 0 1 34 49.9236 140.0764 -4.25641 65 125 1 1 26 -7.287948 132.2879 .0909091 70 135 1 3 26 -36.23022 171.2302 5.090909 68 134 1 3 23 -21.65331 155.6533 3.090909 63 101 1 4 23 -15.71104 116.711 -1.909091 62 115 1 3 26 6.077415 108.9226 -2.909091 60 134 1 2 25 40.65432 93.34567 -4.909091 (headings correspond to the column of data like I have labeled it above; for female, 1=female and 2=male; although not necessary for this exercise, the 1,2,3,4 for eyes just represents 4 different eye colors.) Using the data, estimate a linear probability model that predicts female on the basis of weight. Report the results and interpret them. b) Plot the predicted values on the y-axis (and weight on the x-axis). How many are >1 or <0? c) Use the predicted values to form a binary variable that =1 if the predicted probability of beingfemale is >0.50 and zero otherwise. Form the 2x2 table comparing this prediction to the true value offemale. What percentage of observations are correctly predicted?
height weight female eyes age uhat yhat uuu
74 195 0 1 53 -7.384037 202.384 3.74359
63 120 1 4 33 3.288961 116.711 -1.909091
65 120 1 4 22 -12.28795 132.2879 .0909091
75 210 0 1 44 -.1724911 210.1725 4.74359
66 130 1 3 29 -10.0764 140.0764 1.090909
62 150 1 1 37 41.07742 108.9226 -2.909091
69 230 1 1 34 66.55824 163.4418 4.090909
73 180 0 5 27 -14.59558 194.5956 2.74359
65 170 0 3 47 37.71205 132.2879 -5.25641
72 195 0 2 33 8.192872 186.8071 1.74359
68 120 1 1 25 -35.65331 155.6533 3.090909
70 180 0 5 32 8.769781 171.2302 -.2564103
66 164 0 4 25 23.9236 140.0764 -4.25641
70 190 0 4 24 18.76978 171.2302 -.2564103
63 120 1 3 22 3.288961 116.711 -1.909091
79 225 0 1 21 -16.32631 241.3263 8.743589
71 212 0 1 48 32.98133 179.0187 .7435898
69 195 0 1 27 31.55824 163.4418 -1.25641
74 195 0 1 53 -7.384037 202.384 3.74359
71 190 0 1 30 10.98133 179.0187 .7435898
64 119 1 4 22 -5.499493 124.4995 -.9090909
64 119 1 4 22 -5.499493 124.4995 -.9090909
66 123 0 4 23 -17.0764 140.0764 -4.25641
70 145 0 5 27 -26.23022 171.2302 -.2564103
68 185 0 1 47 29.34669 155.6533 -2.25641
65 112 1 1 23 -20.28795 132.2879 .0909091
72 190 0 3 27 3.192872 186.8071 1.74359
69 165 0 1 36 1.558235 163.4418 -1.25641
65 100 1 1 23 -32.28795 132.2879 .0909091
61 95 1 1 22 -6.13413 101.1341 -3.909091
70 145 0 1 23 -26.23022 171.2302 -.2564103
70 155 0 1 45 -16.23022 171.2302 -.2564103
66 115 1 1 24 -25.0764 140.0764 1.090909
72 190 0 3 24 3.192872 186.8071 1.74359
70 135 1 3 26 -36.23022 171.2302 5.090909
62 115 1 3 26 6.077415 108.9226 -2.909091
71 165 0 3 26 -14.01867 179.0187 .7435898
74 225 0 1 22 22.61596 202.384 3.74359
64 106 1 1 25 -18.49949 124.4995 -.9090909
71 200 0 1 25 20.98133 179.0187 .7435898
68 134 1 3 23 -21.65331 155.6533 3.090909
67 161 0 1 23 13.13514 147.8649 -3.25641
69 165 0 4 22 1.558235 163.4418 -1.25641
65 110 1 1 23 -22.28795 132.2879 .0909091
68 137 1 3 25 -18.65331 155.6533 3.090909
74 207 0 1 52 4.615963 202.384 3.74359
63 101 1 4 23 -15.71104 116.711 -1.909091
65 125 1 1 26 -7.287948 132.2879 .0909091
64 170 1 1 26 45.50051 124.4995 -.9090909
70 195 0 5 23 23.76978 171.2302 -.2564103
64 120 1 2 23 -4.499493 124.4995 -.9090909
70 240 0 2 26 68.76978 171.2302 -.2564103
64 106 1 1 25 -18.49949 124.4995 -.9090909
67 120 0 1 26 -27.86486 147.8649 -3.25641
67 120 1 4 26 -27.86486 147.8649 2.090909
67 161 0 1 23 13.13514 147.8649 -3.25641
66 110 0 4 23 -30.0764 140.0764 -4.25641
72 205 0 5 26 18.19287 186.8071 1.74359
71 200 0 1 25 20.98133 179.0187 .7435898
71 165 0 3 26 -14.01867 179.0187 .7435898
70 170 0 1 26 -1.230219 171.2302 -.2564103
71 167 0 1 23 -12.01867 179.0187 .7435898
64 118 1 4 23 -6.499493 124.4995 -.9090909
65 122 1 1 26 -10.28795 132.2879 .0909091
67 140 0 1 25 -7.864856 147.8649 -3.25641
66 190 0 1 34 49.9236 140.0764 -4.25641
65 125 1 1 26 -7.287948 132.2879 .0909091
70 135 1 3 26 -36.23022 171.2302 5.090909
68 134 1 3 23 -21.65331 155.6533 3.090909
63 101 1 4 23 -15.71104 116.711 -1.909091
62 115 1 3 26 6.077415 108.9226 -2.909091
60 134 1 2 25 40.65432 93.34567 -4.909091
(headings correspond to the column of data like I have labeled it above; for female, 1=female and 2=male; although not necessary for this exercise, the 1,2,3,4 for eyes just represents 4 different eye colors.)
Using the data, estimate a linear probability model that predicts female on the basis of weight. Report the results and interpret them. b) Plot the predicted values on the y-axis (and weight on the x-axis). How many are >1 or <0? c) Use the predicted values to form a binary variable that =1 if the predicted probability of beingfemale is >0.50 and zero otherwise. Form the 2x2 table comparing this prediction to the true value offemale. What percentage of observations are correctly predicted?

Trending now
This is a popular solution!
Step by step
Solved in 3 steps with 8 images

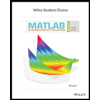
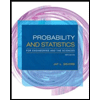
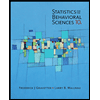
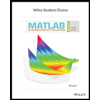
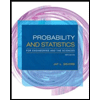
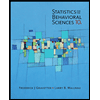
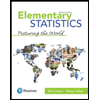
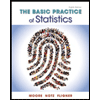
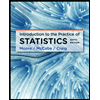