Does correcting the sugar cane model for heteroscedasticity improve its performance? Interpret the regression coefficients.
Correlation
Correlation defines a relationship between two independent variables. It tells the degree to which variables move in relation to each other. When two sets of data are related to each other, there is a correlation between them.
Linear Correlation
A correlation is used to determine the relationships between numerical and categorical variables. In other words, it is an indicator of how things are connected to one another. The correlation analysis is the study of how variables are related.
Regression Analysis
Regression analysis is a statistical method in which it estimates the relationship between a dependent variable and one or more independent variable. In simple terms dependent variable is called as outcome variable and independent variable is called as predictors. Regression analysis is one of the methods to find the trends in data. The independent variable used in Regression analysis is named Predictor variable. It offers data of an associated dependent variable regarding a particular outcome.
Does correcting the sugar cane model for heteroscedasticity improve its performance?
Interpret the regression coefficients.
![REGRESSION:Lhs=SIZE:Rhs=ONE SIZEAGE:Ploet$
Ordinary
least squares regression . .
....
LHS=SIZE
Mean
100.91310
Standard deviation
88.04303
No. of observations =
Regression Sum of Squares
Sum of Squares
Sum of Squares
Standard error of e =
R-squared
Model test F[ 1, 98]
100 DegFreedom Mean square
1 373682.99814
373683.
Residual
393723.
98 4017.58107
Total
767406.
99 7751.57518
63.38439 Root MSE
62.74735
Fit
.48694 R-bar squared
.48171
93.01194 Prob F > F*
.00000
Standard
Prob.
95% Confidence
SIZEĻ Coefficient
Error
t t|>T*
Interval
-1.00 .3217 -40.0573 13.2852
Constant| -13.3860
SIZEAGE| 58.9499*** 6.11243
13.44002
9.64 .0000
46.8200 71.0798
***, **, * ==> Significance at 1%, 5%, 10% level.](/v2/_next/image?url=https%3A%2F%2Fcontent.bartleby.com%2Fqna-images%2Fquestion%2F9ad7de14-e4ff-4bf9-a279-511561965a4a%2Fea363f35-88cc-43f8-a7fa-3ed035102a85%2Fsi76ltf_processed.png&w=3840&q=75)
![Ordinary least squares regression
......
LHS=SUGAR Mean
100.93910
Standard deviation =
58.13665
No. of observations =
100 DegFreedom Mean square
1 11556.17976
Regression Sum of Squares
Residual Sum of Squares
Sum of Squares
Standard error of e
R-squared
Model test F[ 1, 98]
11556.2
323051.
98 3296.43801
=
Total
334607.
99 3379.86975
57.41461 Root MSE
56.83757
.03454 R-bar squared
3.50566 Prob F > F*
Fit
.02468
.06414
Standard
Prob.
95% Confidence
SUGARĮ Coefficient
Error
t t|>T*
Interval
----
Constant| -24.2462
SUGAGE| 63.1839*
-.36 .7186 -157.4168 108.9243
1.87 .0641
67.10644
33.74596
-3.7839 130.1516
***, **, * ==> Significance at 1%, 5%, 10% level.](/v2/_next/image?url=https%3A%2F%2Fcontent.bartleby.com%2Fqna-images%2Fquestion%2F9ad7de14-e4ff-4bf9-a279-511561965a4a%2Fea363f35-88cc-43f8-a7fa-3ed035102a85%2Fx4dgei_processed.png&w=3840&q=75)

Step by step
Solved in 2 steps

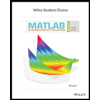
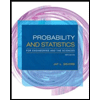
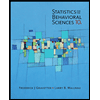
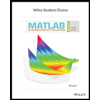
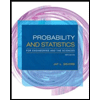
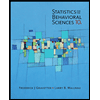
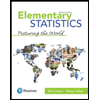
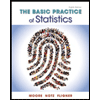
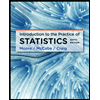