array([[ 1960, 54211], [ 1961, 55438], [ 1962, 56225], [ 1963, 56695], [ 1964, 57032], [ 1965, 57360], [ 1966, 57715], [ 1967, 58055], [ 1968, 58386], [ 1969, 58726], [ 1970, 59063], [ 1971, 59440], [ 1972, 59840], [ 1973, 60243], [ 1974, 60528], [ 1975, 60657], [ 1976, 60586], [ 1977, 60366], [ 1978, 60103], [ 1979, 59980], [ 1980, 60096], [ 1981, 60567], [ 1982, 61345], [ 1983, 62201], [ 1984, 62836], [ 1985, 63026], [ 1986, 62644], [ 1987, 61833], [ 1988, 61079], [ 1989, 61032], [ 1990, 62149], [ 1991, 64622], [ 1992, 68235], [ 1993, 72504], [ 1994, 76700], [ 1995, 80324], [ 1996, 83200], [ 1997, 85451], [ 1998, 87277], [ 1999, 89005], [ 2000, 90853], [ 2001, 92898], [ 2002, 94992], [ 2003, 97017], [ 2004, 98737], [ 2005, 100031], [ 2006, 100832], [ 2007, 101220], [ 2008, 101353], [ 2009, 101453], [ 2010, 101669], [ 2011, 102053], [ 2012, 102577], [ 2013, 103187], [ 2014, 103795], [ 2015, 104341], [ 2016, 104822], [ 2017, 105264]]) Question 2 Now that we have have our data, we need to split this into a training set, and a testing set. But before we split our data into training and testing, we also need to split our data into the predictive features (denoted X) and the response (denoted y). Write a function that will take as input a 2-d numpy array and return four variables in the form of (X_train, y_train), (X_test, y_test), where (X_train, y_train) are the features + response of the training set, and (X-test, y_test) are the features + response of the testing set. Function Specifications: Should take a 2-d numpy array as input. Should split the array such that X is the year, and y is the corresponding population. Should return two tuples of the form (X_train, y_train), (X_test, y_test). Should use sklearn's train_test_split function with a test_size = 0.2 and random_state = 42. Failing Code: def feature_response_split(arr): X, y = arr[:, 0], arr[:, 1] X_train, X_test, y_train, y_test = train_test_split(X.reshape(-1,1), y, test_size = 0.2, random_state = 42) return (X_train, y_train, X_test,y_test) Expected Output: X_train == array([1996, 1991, 1968, 1977, 1966, 1964, 2001, 1979, 1990, 2009, 2010, 2014, 1975, 1969, 1987, 1986, 1976, 1984, 1993, 2015, 2000, 1971, 1992, 2016, 2003, 1989, 2013, 1961, 1981, 1962, 2005, 1999, 1995, 1983, 2007, 1970, 1982, 1978, 2017, 1980, 1967, 2002, 1974, 1988, 2011, 1998]) y_train == array([ 83200, 64622, 58386, 60366, 57715, 57032, 92898, 59980, 62149, 101453, 101669, 103795, 60657, 58726, 61833, 62644, 60586, 62836, 72504, 104341, 90853, 59440, 68235, 104822, 97017, 61032, 103187, 55438, 60567, 56225, 100031, 89005, 80324, 62201, 101220, 59063, 61345, 60103, 105264, 60096, 58055, 94992, 60528, 61079, 102053, 87277]) X_test == array([1960, 1965, 1994, 1973, 2004, 2012, 1997, 1985, 2006, 1972, 2008, 1963]) y_test == array([ 54211, 57360, 76700, 60243, 98737, 102577, 85451, 63026, 100832, 59840, 101353, 56695])
Input array is :-
array([[ 1960, 54211], [ 1961, 55438], [ 1962, 56225], [ 1963, 56695], [ 1964, 57032], [ 1965, 57360], [ 1966, 57715], [ 1967, 58055], [ 1968, 58386], [ 1969, 58726], [ 1970, 59063], [ 1971, 59440], [ 1972, 59840], [ 1973, 60243], [ 1974, 60528], [ 1975, 60657], [ 1976, 60586], [ 1977, 60366], [ 1978, 60103], [ 1979, 59980], [ 1980, 60096], [ 1981, 60567], [ 1982, 61345], [ 1983, 62201], [ 1984, 62836], [ 1985, 63026], [ 1986, 62644], [ 1987, 61833], [ 1988, 61079], [ 1989, 61032], [ 1990, 62149], [ 1991, 64622], [ 1992, 68235], [ 1993, 72504], [ 1994, 76700], [ 1995, 80324], [ 1996, 83200], [ 1997, 85451], [ 1998, 87277], [ 1999, 89005], [ 2000, 90853], [ 2001, 92898], [ 2002, 94992], [ 2003, 97017], [ 2004, 98737], [ 2005, 100031], [ 2006, 100832], [ 2007, 101220], [ 2008, 101353], [ 2009, 101453], [ 2010, 101669], [ 2011, 102053], [ 2012, 102577], [ 2013, 103187], [ 2014, 103795], [ 2015, 104341], [ 2016, 104822], [ 2017, 105264]])
Question 2
Now that we have have our data, we need to split this into a training set, and a testing set. But before we split our data into training and testing, we also need to split our data into the predictive features (denoted X) and the response (denoted y).
Write a function that will take as input a 2-d numpy array and return four variables in the form of (X_train, y_train), (X_test, y_test), where (X_train, y_train) are the features + response of the training set, and (X-test, y_test) are the features + response of the testing set.
Function Specifications:
- Should take a 2-d numpy array as input.
- Should split the array such that X is the year, and y is the corresponding population.
- Should return two tuples of the form (X_train, y_train), (X_test, y_test).
- Should use sklearn's train_test_split function with a test_size = 0.2 and random_state = 42.
Failing Code:
def feature_response_split(arr):
X, y = arr[:, 0], arr[:, 1]
X_train, X_test, y_train, y_test = train_test_split(X.reshape(-1,1), y, test_size = 0.2, random_state = 42)
return (X_train, y_train, X_test,y_test)
Expected Output:
X_train == array([1996, 1991, 1968, 1977, 1966, 1964, 2001, 1979, 1990, 2009, 2010, 2014, 1975, 1969, 1987, 1986, 1976, 1984, 1993, 2015, 2000, 1971, 1992, 2016, 2003, 1989, 2013, 1961, 1981, 1962, 2005, 1999, 1995, 1983, 2007, 1970, 1982, 1978, 2017, 1980, 1967, 2002, 1974, 1988, 2011, 1998])
y_train == array([ 83200, 64622, 58386, 60366, 57715, 57032, 92898, 59980, 62149, 101453, 101669, 103795, 60657, 58726, 61833, 62644, 60586, 62836, 72504, 104341, 90853, 59440, 68235, 104822, 97017, 61032, 103187, 55438, 60567, 56225, 100031, 89005, 80324, 62201, 101220, 59063, 61345, 60103, 105264, 60096, 58055, 94992, 60528, 61079, 102053, 87277])
X_test == array([1960, 1965, 1994, 1973, 2004, 2012, 1997, 1985, 2006, 1972, 2008, 1963])
y_test == array([ 54211, 57360, 76700, 60243, 98737, 102577, 85451, 63026, 100832, 59840, 101353, 56695])

Step by step
Solved in 3 steps with 1 images

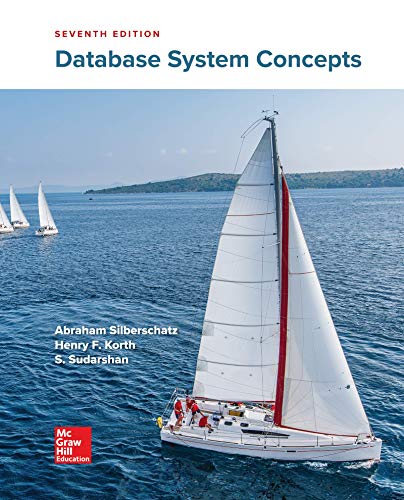
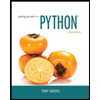
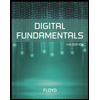
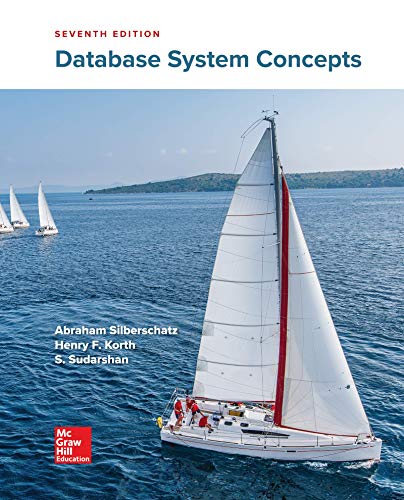
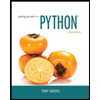
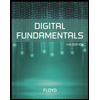
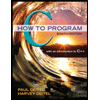
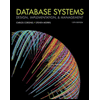
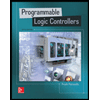