An observational study was conducted where subjects were randomly sampled and then had their resting heart rate recorded, as well as their smoking status (0 for non-smoker and 1 for smoker) and how much they exercise on average each day (in hours). A linear regression model is fit where we have response variable of resting heart rate and explanatory variables of smoking status (0 for non-smoker and 1 for smoker) and exercise amount per day in hours, along with an interaction between smoking status and exercise amount. The output is below: Coefficients: Estimate Std. Error t value Pr(>|t|) (Intercept) 84.8172 1.9553 43.377 <2e-16 *** Smoke -2.2645 5.0665 -0.447 0.6551 Exercise -7.3684 0.8075 -9.125 <2e-16 *** Smoke:Exercise 1.9562 2.5510 0.767 0.4442 Signif. codes: O ***> 0.001 (*** 0.01 (*) 0.05 . 0.1 1 Residual standard error: 8.396 on 228 degrees of freedom Adjusted R-squared: 0.2879 F-statistic: 32.13 on 3 and 228 DF, p-value: < 2.2e-16 Multiple R-squared: 0.2971, The model that was fit is: Rest; = Bo + B1 Smoke; + B2Exercise; + B3Smoke; * Exercise; + E;
An observational study was conducted where subjects were randomly sampled and then had their resting heart rate recorded, as well as their smoking status (0 for non-smoker and 1 for smoker) and how much they exercise on average each day (in hours). A linear regression model is fit where we have response variable of resting heart rate and explanatory variables of smoking status (0 for non-smoker and 1 for smoker) and exercise amount per day in hours, along with an interaction between smoking status and exercise amount. The output is below: Coefficients: Estimate Std. Error t value Pr(>|t|) (Intercept) 84.8172 1.9553 43.377 <2e-16 *** Smoke -2.2645 5.0665 -0.447 0.6551 Exercise -7.3684 0.8075 -9.125 <2e-16 *** Smoke:Exercise 1.9562 2.5510 0.767 0.4442 Signif. codes: O ***> 0.001 (*** 0.01 (*) 0.05 . 0.1 1 Residual standard error: 8.396 on 228 degrees of freedom Adjusted R-squared: 0.2879 F-statistic: 32.13 on 3 and 228 DF, p-value: < 2.2e-16 Multiple R-squared: 0.2971, The model that was fit is: Rest; = Bo + B1 Smoke; + B2Exercise; + B3Smoke; * Exercise; + E;
MATLAB: An Introduction with Applications
6th Edition
ISBN:9781119256830
Author:Amos Gilat
Publisher:Amos Gilat
Chapter1: Starting With Matlab
Section: Chapter Questions
Problem 1P
Related questions
Question
Calculate the estimate of σε
What is the estimated resting heart rate for someone who is a smoker (Smoke=1) and exercises 1 hour a day (Exercise=1)?
![**Study Context**
An observational study was conducted where subjects were randomly sampled. Their resting heart rate, smoking status (coded as 0 for non-smoker and 1 for smoker), and average daily exercise (measured in hours) were recorded.
**Objective**
A linear regression model was fitted using:
- Response Variable: Resting heart rate
- Explanatory Variables: Smoking status, exercise duration, and an interaction term between smoking status and exercise duration.
**Regression Output**
- **Coefficients:**
| Term | Estimate | Std. Error | t value | Pr(>|t|) |
|------------------|----------|------------|---------|------------|
| (Intercept) | 84.8172 | 1.9553 | 43.377 | <2e-16 *** |
| Smoke | -2.2645 | 5.0665 | -0.447 | 0.6551 |
| Exercise | -7.3684 | 0.8075 | -9.125 | <2e-16 *** |
| Smoke:Exercise | 1.9562 | 2.5510 | 0.767 | 0.4442 |
- **Significance Codes:**
- "***" : p-value < 0.001
- "**" : p-value < 0.01
- "*" : p-value < 0.05
- "." : p-value < 0.1
- **Model Statistics:**
- Residual standard error: 8.396 on 228 degrees of freedom
- Multiple R-squared: 0.2971
- Adjusted R-squared: 0.2879
- F-statistic: 32.13 on 3 and 228 DF, p-value: < 2.2e-16
**Fitted Model**
The linear regression model is formulated as:
\[ \text{Rest}_i = \beta_0 + \beta_1 \text{Smoke}_i + \beta_2 \text{Exercise}_i + \beta_3 \text{Smoke}_i \times \text{Exercise}_i + \epsilon_i \]
Where:
- \(\beta_0\), \(\beta_1\), \(\beta](/v2/_next/image?url=https%3A%2F%2Fcontent.bartleby.com%2Fqna-images%2Fquestion%2Fa073fe10-71d5-4b67-9305-488739d34eae%2Fb6045e7f-c7b8-471f-91b8-e0856f0cd75a%2Fj23988b_processed.png&w=3840&q=75)
Transcribed Image Text:**Study Context**
An observational study was conducted where subjects were randomly sampled. Their resting heart rate, smoking status (coded as 0 for non-smoker and 1 for smoker), and average daily exercise (measured in hours) were recorded.
**Objective**
A linear regression model was fitted using:
- Response Variable: Resting heart rate
- Explanatory Variables: Smoking status, exercise duration, and an interaction term between smoking status and exercise duration.
**Regression Output**
- **Coefficients:**
| Term | Estimate | Std. Error | t value | Pr(>|t|) |
|------------------|----------|------------|---------|------------|
| (Intercept) | 84.8172 | 1.9553 | 43.377 | <2e-16 *** |
| Smoke | -2.2645 | 5.0665 | -0.447 | 0.6551 |
| Exercise | -7.3684 | 0.8075 | -9.125 | <2e-16 *** |
| Smoke:Exercise | 1.9562 | 2.5510 | 0.767 | 0.4442 |
- **Significance Codes:**
- "***" : p-value < 0.001
- "**" : p-value < 0.01
- "*" : p-value < 0.05
- "." : p-value < 0.1
- **Model Statistics:**
- Residual standard error: 8.396 on 228 degrees of freedom
- Multiple R-squared: 0.2971
- Adjusted R-squared: 0.2879
- F-statistic: 32.13 on 3 and 228 DF, p-value: < 2.2e-16
**Fitted Model**
The linear regression model is formulated as:
\[ \text{Rest}_i = \beta_0 + \beta_1 \text{Smoke}_i + \beta_2 \text{Exercise}_i + \beta_3 \text{Smoke}_i \times \text{Exercise}_i + \epsilon_i \]
Where:
- \(\beta_0\), \(\beta_1\), \(\beta
Expert Solution

This question has been solved!
Explore an expertly crafted, step-by-step solution for a thorough understanding of key concepts.
This is a popular solution!
Trending now
This is a popular solution!
Step by step
Solved in 2 steps with 2 images

Recommended textbooks for you
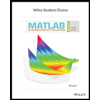
MATLAB: An Introduction with Applications
Statistics
ISBN:
9781119256830
Author:
Amos Gilat
Publisher:
John Wiley & Sons Inc
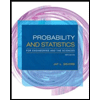
Probability and Statistics for Engineering and th…
Statistics
ISBN:
9781305251809
Author:
Jay L. Devore
Publisher:
Cengage Learning
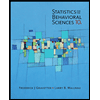
Statistics for The Behavioral Sciences (MindTap C…
Statistics
ISBN:
9781305504912
Author:
Frederick J Gravetter, Larry B. Wallnau
Publisher:
Cengage Learning
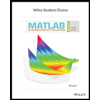
MATLAB: An Introduction with Applications
Statistics
ISBN:
9781119256830
Author:
Amos Gilat
Publisher:
John Wiley & Sons Inc
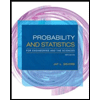
Probability and Statistics for Engineering and th…
Statistics
ISBN:
9781305251809
Author:
Jay L. Devore
Publisher:
Cengage Learning
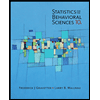
Statistics for The Behavioral Sciences (MindTap C…
Statistics
ISBN:
9781305504912
Author:
Frederick J Gravetter, Larry B. Wallnau
Publisher:
Cengage Learning
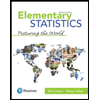
Elementary Statistics: Picturing the World (7th E…
Statistics
ISBN:
9780134683416
Author:
Ron Larson, Betsy Farber
Publisher:
PEARSON
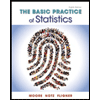
The Basic Practice of Statistics
Statistics
ISBN:
9781319042578
Author:
David S. Moore, William I. Notz, Michael A. Fligner
Publisher:
W. H. Freeman
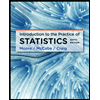
Introduction to the Practice of Statistics
Statistics
ISBN:
9781319013387
Author:
David S. Moore, George P. McCabe, Bruce A. Craig
Publisher:
W. H. Freeman