2. Consider two least-squares regressions y = Xıı + è
Advanced Engineering Mathematics
10th Edition
ISBN:9780470458365
Author:Erwin Kreyszig
Publisher:Erwin Kreyszig
Chapter2: Second-order Linear Odes
Section: Chapter Questions
Problem 1RQ
Related questions
Question
explain into details. Thank you.

Transcribed Image Text:### Least-Squares Regressions Analysis
**Problem Statement:**
Consider two least-squares regressions:
1. \( y = X_1\hat{\beta}_1 + \bar{e} \)
2. \( y = X_1\hat{\beta}_1 + X_2\hat{\beta}_2 + \hat{e} \)
Let \( R_1^2 \) and \( R_2^2 \) be the R-squared values from the two regressions. Show that \( R_2^2 \geq R_1^2 \).
**Explanation:**
This problem involves understanding how adding additional explanatory variables in a regression model affects the R-squared value. The R-squared value (\( R^2 \)) provides a measure of how well the independent variables explain the variability in the dependent variable. Increasing the number of independent variables generally cannot decrease the R-squared value, and it often increases it. This is because adding more variables either explains additional variance or results in no change at all if the variables are not significant.
**Details:**
1. **First Regression:**
\( y = X_1\hat{\beta}_1 + \bar{e} \)
Here, the dependent variable \( y \) is regressed on a single independent variable \( X_1 \) with estimated coefficient \( \hat{\beta}_1 \) and error term \( \bar{e} \). The R-squared value for this regression is denoted as \( R_1^2 \).
2. **Second Regression:**
\( y = X_1\hat{\beta}_1 + X_2\hat{\beta}_2 + \hat{e} \)
In this case, the dependent variable \( y \) is regressed on two independent variables \( X_1 \) and \( X_2 \) with estimated coefficients \( \hat{\beta}_1 \) and \( \hat{\beta}_2 \) respectively, and error term \( \hat{e} \). The R-squared value for this regression is denoted as \( R_2^2 \).
**Objective:**
To demonstrate that adding an additional variable \( X_2 \) in the regression model will result in an R-squared value (\( R_2^2 \)) that is at least as large as the R-squared value (\(
Expert Solution

This question has been solved!
Explore an expertly crafted, step-by-step solution for a thorough understanding of key concepts.
This is a popular solution!
Trending now
This is a popular solution!
Step by step
Solved in 3 steps with 2 images

Recommended textbooks for you
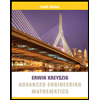
Advanced Engineering Mathematics
Advanced Math
ISBN:
9780470458365
Author:
Erwin Kreyszig
Publisher:
Wiley, John & Sons, Incorporated
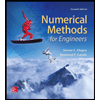
Numerical Methods for Engineers
Advanced Math
ISBN:
9780073397924
Author:
Steven C. Chapra Dr., Raymond P. Canale
Publisher:
McGraw-Hill Education
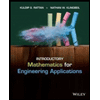
Introductory Mathematics for Engineering Applicat…
Advanced Math
ISBN:
9781118141809
Author:
Nathan Klingbeil
Publisher:
WILEY
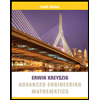
Advanced Engineering Mathematics
Advanced Math
ISBN:
9780470458365
Author:
Erwin Kreyszig
Publisher:
Wiley, John & Sons, Incorporated
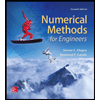
Numerical Methods for Engineers
Advanced Math
ISBN:
9780073397924
Author:
Steven C. Chapra Dr., Raymond P. Canale
Publisher:
McGraw-Hill Education
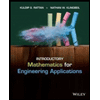
Introductory Mathematics for Engineering Applicat…
Advanced Math
ISBN:
9781118141809
Author:
Nathan Klingbeil
Publisher:
WILEY
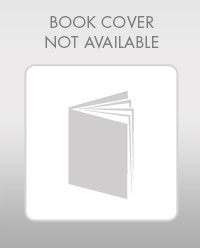
Mathematics For Machine Technology
Advanced Math
ISBN:
9781337798310
Author:
Peterson, John.
Publisher:
Cengage Learning,
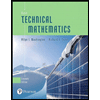
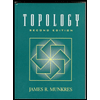