Weapons of Math Destruction Major and Minor Quotes
“The privileged, we’ll see time and again, are processed more by people, the masses by machines.” (Introduction)
—Cathy O’Neil
Analysis: Though the idea of being “processed by machines” may sound utterly dystopian, O’Neil shows throughout her book that this statement holds true in many areas of life. Some of her strongest examples come from recruitment and personnel management: educators like Sarah Wysocki have little bargaining power when an algorithm rates them as bad teachers. Those applying for entry-level work in retail and food service arguably have it even worse, since the huge number of applications in these areas makes automation inevitable. O’Neil does acknowledge that sometimes, humans are not the fairest judges of who should receive a loan, a job offer, or time off for good behavior. Still, if humans have their own biases and irrationalities, they can at least be reasoned with.
In Chapter 8, O’Neil gives some especially direct illustrations of the difference that human intervention can make in an otherwise automated process. She asks the reader to consider a Stanford law graduate who, in a job interview, is misidentified as a criminal by a background check. She points out that in this situation, the interviewer would likely realize the discrepancy and either reject the background check’s claim out of hand or at least follow up on it before assuming it to be true.
“Welcome to the dark side of Big Data.” (Introduction)
—Cathy O’Neil
Analysis: The introduction of Weapons of Math Destruction sets the tone for O’Neil’s discussion of mathematical models in public life. Her allusion to “the dark side of Big Data” implies a contrast with a bright side, and indeed O’Neil is candid about the fact that many people are eagerly promoting the benefits of Big Data. Much later in the book, O’Neil will cautiously express her own hope that Big Data modeling and analysis can be used for good: not to target vulnerable people and punish them but to identify those people and help them. This is not the norm, however, and the naive or cynical use of Big Data practices constitutes a “dark side” that O’Neil deems insufficiently discussed.
Given the way the term is used here as well as in the title, it’s important to be clear that O’Neil is referring to Big Data as an industry and a set of practices, some of which she deems dangerous, within that industry. Herself a data scientist, O’Neil is not arguing that the collection, analysis, and use of large-scale data are somehow inherently evil or harmful. In this sense, the term is somewhat akin to criticisms of “Big Pharma,” which take aim at the behavior of large pharmaceutical companies and not at pharmacology per se. Few would argue that manufacturing medicine on a large scale is unethical, but many would question—and have questioned—the ways that medicines are marketed, priced, and distributed, and the same is true with the critique of Big Data.
“Models are opinions embedded in mathematics.” (Chapter 1)
—Cathy O’Neil
Analysis: One reason that mathematical models have become so popular, O’Neil suggests, is that they give an impression of objectivity. The people who create models of worker performance, criminal recidivism, or scholastic aptitude often present those models as an improvement over the biased and fallible judgment of individuals. And in some senses, O’Neil acknowledges, this is true. A credit bureau doesn’t have personal prejudices; it doesn’t have children or go to church, so it doesn’t favor fellow parishioners or the parents of its child’s classmates. Sometimes there is a clear statistical improvement over purely human decision-making. Recidivism models, for instance, give some people lighter sentences than they might have received if judges’ personal biases held more sway.
Yet although they are based in statistics and mathematics, these models ultimately “embed” the goals, priorities, and beliefs of those who create them. On the small scale of O’Neil’s immediate example, a parent’s informal model for mealtime involves a balance of nutrition, cost, convenience, and personal tastes. How to weight these factors is a matter of opinion: some parents may take a hard line against junk food while others may be more lenient. This is true on a larger scale as well. Workplace personality tests, which O’Neil covers in Chapter 6, reflect often-untested beliefs about what personality traits are most important on the job. College rankings, in order to be seen as legitimate by the public, must follow a model that puts the expected winners at or near the top. The fact that a model applies mathematical reasoning to a set of data does not automatically make it fair or impartial.
“Every ranking system can be gamed.” (Chapter 3)
—Cathy O’Neil
Analysis: In her discussion of college rankings, O’Neil points out that the ranking criteria come to dominate the thinking and the budgeting of college administrators. This has two parallel effects: On the one hand, criteria not used for ranking are treated as irrelevant. For instance, school ranking systems in the United States do not traditionally take the cost of attendance into account. In effect, the exclusion of cost incentivizes schools to spend and charge as much as they can. A related effect, and the one noted here, is that a ranking system usually rewards certain narrowly defined metrics such as publications and citations. These metrics can be “improved” dramatically with little effect on the underlying quality of the school.
O’Neil draws her illustration from the math department at King Abdulaziz University in Saudi Arabia. This large public university is well-regarded regionally, but until the mid-2010s, its mathematics department was not particularly prominent or highly ranked. The school’s administrators found a solution that, to O’Neil at least, provides a classic example of gamesmanship. They hired prominent mathematicians as adjuncts on the understanding that they would have to teach only three weeks per year. Their status as King Abdulaziz University affiliates meant that the university would be able to claim their highly cited publications as its own for ranking purposes.
“Justice cannot just be something that one part of society inflicts upon the other.” (Chapter 5)
—Cathy O’Neil
Analysis: Here, O’Neil makes the point that the people designing and deploying WMDs (“Weapons of Math Destruction”) are not generally the same ones being targeted by those models. The developers and marketers of predictive policing software, for instance, do not generally reside in the “dangerous,” “high-crime” neighborhoods that the software flags for extra police attention. They thus do not directly experience the indignity of being frequently stopped and searched by police officers in the course of their day-to-day life. If police patrolled Chicago’s Gold Coast the way they patrol poorer neighborhoods, she suggests, its residents would be outraged.
Elsewhere, in her chapters on payday lending, for-profit colleges, and consumer finance, O’Neil has more to say about the way algorithmic results are “inflicted” on poor individuals and communities. She observes from firsthand experience that the people who design targeted advertising campaigns would never buy much of what they sell. Recruiting managers at for-profit schools, for instance, have a clear and often rather unflattering model of those they are selling to. In cases like these, O’Neil argues, justice demands that some features be excluded from the models, even if their inclusion would potentially make the model more accurate. This is true, she suggests, even in cases where the goal is to prevent serious crime.
“We can use the scale and efficiency that make WMDs so pernicious in order to help people. It all depends on the objective we choose.” (Chapter 6)
—Cathy O’Neil
Analysis: Much of O’Neil’s own career has focused on the positive, prosocial use of Big Data algorithms. After leaving her job as a hedge fund quant, as she details in Chapter 2, O’Neil began looking for ways to use data science to benefit society at large, not just a select group. Throughout Weapons of Math Destruction, O’Neil gives examples of models that use dubious data to assess the employability and creditworthiness of individuals. Some of these models have substantial flaws that limit their accuracy and exclude potentially valuable customers or employees. Others, however, are exceptionally apt at identifying vulnerable individuals: people in dire enough financial straits that they will consider a payday loan, for instance. These algorithms are “working” in that they successfully—and, for their makers, profitably—identify people with common traits.
The problem, for O’Neil, is the goal of this targeting. Scale and efficiency are in themselves neutral qualities. When a harmful activity is carried out efficiently and at scale, the results are disastrous, as O’Neil shows in examples of predatory advertising and biased employment screenings. Here, however, O’Neil asks the reader to consider the possibility that the same scalable, efficient modeling strategies could be pressed into service to find and help vulnerable individuals, instead of offering them overpriced degrees or loans with exorbitant interest rates. The same fundamental tools can be wielded for drastically different purposes.
“[These systems] urgently require the context, common sense, and fairness that only humans can provide.” (Chapter 8)
—Cathy O’Neil
Analysis: One of O’Neil’s points of disagreement with Big Data “evangelists” concerns the role of human beings. Some of the most enthusiastic proponents of predictive modeling, she says, suggest that Big Data models and algorithms should be largely left to their own devices without human interference. If properly set up, they argue, the process will play out with an efficiency that can only be diminished, not improved, by human meddling.
O’Neil rejects this idea as naively optimistic. She argues instead that humans have a very real role to play in supervising the creation and deployment of algorithms. Here, she cites three related ways in which people can (and, in her view, should) scrutinize and correct the models used in finance, employment, and politics. Fairness, O’Neil argues, is a human value that has not been successfully communicated to machines and is often directly at odds with efficiency. An algorithm can satisfy certain parameters, like making sure that all job applicants get the same battery of questions, but it cannot decide whether people are being treated fairly. Likewise, some things that promote efficiency within a scheduling model—like clopenings—fail to make sense in the context of an individual’s daily or weekly routine. A human can look at such a schedule and see that it makes unreasonable demands of the worker, but a machine cannot make such a subjective judgment.
“Being poor in a world of WMDs is getting more and more dangerous and expensive.” (Conclusion)
—Cathy O’Neil
Analysis: This quotation sums up the effects of WMDs (“Weapons of Math Destruction”) that O’Neil enumerates throughout the book. She shows that several distinct types of models in several different industries tend to penalize poor people. These models correlate poverty with criminal recidivism, untrustworthiness on the job, reckless driving, and many other traits and behaviors that may hamper a person’s life chances. Worse, these individual models combine to create a “poverty trap” in which the effects of one model become inputs for the next. A person who is deemed a credit risk will also, increasingly, be labeled a risky hire and an easy mark for overpriced loans and low-value credentials.
O’Neil acknowledges not everyone involved in creating these systems is out to target poor people directly. Some, such as those who build predatory advertising campaigns based on customers’ ignorance and desperation, surely are. But others, as O’Neil notes, are just doing what data scientists are trained to do: looking for correlations and determining how different variables fit together. Whether or not they are aware of it, however, they are collectively assembling a trap that is easy for vulnerable individuals to fall into and hard to escape from.
“Big Data processes codify the past. They do not invent the future.” (Conclusion)
—Cathy O’Neil
Analysis: This statement reflects one of O’Neil’s main arguments in favor of greater human oversight for “Big Data processes,” a collective term for the models and algorithms she cites elsewhere in the book. Because these processes take their data from what has happened in the past, they easily “learn” and apply patterns that reflect the circumstances of the past. For example, in 2017—the publication year of the revised edition of Weapons of Math Destruction—women made up about 35% of the physician workforce in the United States. The figure was much lower even a decade earlier. An algorithm trained on data from the mid-20th century might well conclude that being male was an essential success factor for being a physician, even though history has since proven otherwise. In fact, in Chapter 6, O’Neil recounts a similar pattern of gender bias when a London medical school attempted to automate parts of its admissions screening. The system replicated the patterns it was given, including trends later recognized as unjust.
This kind of reliance on past data poses a problem for anyone who wishes to use Big Data to “invent” a more equitable future. O’Neil’s solution is to involve humans—ideally, a group of stakeholders from throughout society and not just data professionals—in the evaluation and development of algorithms for fairness and reliability. Without such oversight, an automated process may well conflate “the way things were” with “the way things should be.”
“We need to stop relying on blind faith and start putting the ‘science’ into data science.” (Afterword)
—Cathy O’Neil
Analysis: One of the ironies of the Big Data industry, in O’Neil’s view, is that so much gets taken on faith. The people who develop, employ, and market Big Data models and algorithms, she says, often believe in the impartiality of their products, since computers do not have personal prejudices or vague hunches to cloud their judgment. In many cases, however, the models are based on flawed assumptions, or they rely on proxies that do not tightly correlate with the thing they purport to measure. They are wound up and set loose, so to speak, without any systematic attempt to incorporate new feedback for further refinement. O’Neil earlier offers the example of personality tests in hiring: if no follow-up data are collected about the applicants rejected as a poor fit, there is no way of assessing the tests for false negatives.
If the algorithms truly are rigorous and fair, O’Neil suggests, they should be able to stand up to scrutiny. Yet few companies or governments have been willing to allow outside experts to conduct meaningful audits of the algorithms they employ. O’Neil suggests that this kind of transparency, if widely demanded, would represent a major step forward. If acted upon, it would help these algorithms live up to the scientific image they project to the public.
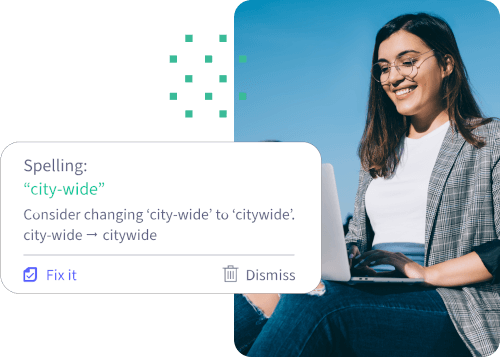