Weapons of Math Destruction Main Ideas
Technology
O’Neil’s understanding of technology and its proper role in society is central to Weapons of Math Destruction. As she establishes early on in the book, O’Neil does not believe that people can or should do away with mathematical modeling as a tool for addressing society’s needs. Nor does she present a broad argument about resisting technological change. O’Neil does, however, argue that certain uses of technology—such as handing off important decisions to algorithms without oversight—cause widespread harm and promote injustice. Sometimes, the design of the model is to blame, as when a school ranking system uses criteria that reflect the wealth of the attendees more than the effectiveness of the education. In other cases, such as personality testing in corporate recruitment, the model itself is potentially very useful, but it is applied inappropriately.
The upside of mathematical modeling and related technologies is not a focus in O’Neil’s book, mainly because there are so many “evangelists” already preaching the benefits. With that said, O’Neil does give some concrete examples of how these technologies are being harnessed for benign and even laudable purposes. She notes, for instance, that the same kinds of tools used to filter spam from email have been adapted to screen for HIV. O’Neil also holds out hope that the technologies currently used to target vulnerable people will be repurposed to help them. She envisions a future in which algorithms identify the people who most need housing assistance, not the ones most likely to sign on to a predatory loan.
Discrimination
Throughout Weapons of Math Destruction, O’Neil shows how discrimination persists even in areas where overt discriminatory practices have been formally outlawed. Big Data, she suggests, is facilitating the creation of tools that target people based on income, race, health and disability status, and perceived creditworthiness even when these factors are officially off the table. Lenders and insurers in the United States once used race, for instance, as a criterion in screening applicants for loans and policies, a practice that in the housing industry was known as redlining. The banning of this practice prevents some forms of discrimination, but O’Neil cautions that many ostensibly nonracial metrics accomplish the same result. For instance, any score or screening that considers one’s zip code is using a proxy that correlates with both race and socioeconomic status. Judging people who live in inner-city Detroit as less creditworthy may not technically be racial discrimination, but statistically it yields the same result.
Likewise, as O’Neil mentions in Chapter 6, companies are broadly prohibited from using medical exams and intelligence tests in their hiring decisions. Yet many companies get around this by using “personality tests” that O’Neil suggests indirectly screen for mental illness, and they run corporate wellness programs that penalize people with poor health metrics. Credit scores, too, are legally barred from consideration in hiring, but companies perform their own more limited credit checks to assess an applicant’s trustworthiness and responsibility. The net effect is to penalize some groups for conditions that may not be under their control or immediately relevant to their job performance—in other words, to discriminate.
Democracy
Another pillar of O’Neil’s argument is that Big Data models and algorithms promote the fragmentation of the voter base and undermine democracy. This topic looms largest at the end of the book, where political campaigns serve as examples of the sophisticated “microtargeting” now used to reach potential voters and consumers alike. In voter microtargeting models, politicians provide different messages to potential voters depending on their likely motivations for voting. Wealthier individuals hear about favorable aspects of a candidate’s tax policy while less wealthy people hear about plans to build and protect the social safety net. Those in the Pacific Northwest may get a message about the candidate’s policies to combat global warming, a message not sent to their peers in coal country. In itself, the idea of tailoring one’s message to one’s audience is nothing new, but microtargeting tools allow politicians to take the process to extremes. The result, for O’Neil, is a system in which politicians masquerade as many—sometimes incompatible—personas to many people, with no one quite sure what policies will actually be pursued after election day. Moreover, statistical models give political campaigns a highly sophisticated ability to determine which voters could “swing” an election and which ones may be convinced to donate to the campaign. The result is that these voters’ opinions count for more in drafting and publicizing the candidates’ platforms. To O’Neil, this is fundamentally undemocratic, since it makes some votes count for more than others.
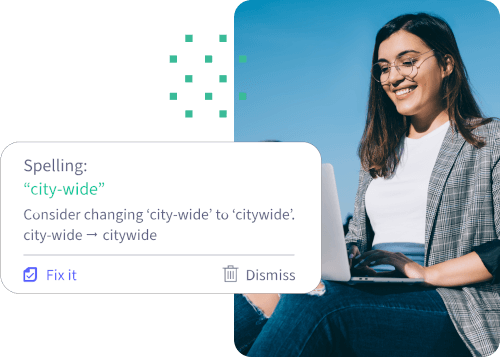