docx
keyboard_arrow_up
School
Liberty University *
*We aren’t endorsed by this school
Course
BASIC
Subject
Statistics
Date
Jan 9, 2024
Type
docx
Pages
14
Uploaded by JusticeFogPrairieDog37
Sindy Saintclair
Monday, November 28 2021
Lesson 7 – Uniform, Binomial, Student’s T, and F-Distributions
Learning
Objectives
and
Questions
Notes and Answers
Understan
d the
properties
of the
uniform
and
binomial
distributio
ns
The Uniform Distribution
All options have an equal opportunity of being selected
Looks flat and rectangular in shape if discrete, or in other
words whole numbered
If on a continuous variable, the same box shape would be
seen but, on a histogram, there would be little variations
because of what comes after the decimal place.
Not very common and does not play a large role in statistics
Parameters of the Continuous Uniform Distribution
Mean – midpoint between the min and max
Median – same as the mean
Range – max minus min
Standard Deviation – 30% of the range
Though the bars are not labeled in this graph, suppose they
are labeled 8 – 18. Since the variable is discrete, the value of
9.3 will never happen, so the probability of that outcome is 0.
Each number from 8 – 18 is possible and equally as likely as
any other. Each number 8 – 18 has a probability of about
0.091 of occurring. However, numbers less than 8 or greater
than 18 are not possible, so their probability is 0.
A common example of a discrete uniform variable is the
rolling of the single 6-sided die. Each number 1 -6 has an
equal probability of occurring (1/6 or 0.167), and it is
impossible to roll a 0 or a 7 or greater with a single die. A
spinner with equal sized pie shape pieces is another example
of a discrete uniform variable.
Population Parameters of the Uniformly Distributed
Continuous Variable
These numbers were generated using 2 and 4 as the
boundaries. The data are bucketed into buckets that are 0.1
units wide, and it gives the impression of being discrete, but
you can imagine if the ‘curve’ was smoothed a bit, and
infinitely many random numbers were created, it would look a
lot like the continuous distribution shown above.
Mean: 3
Median: 3
Standard Deviation: 0.578
Min: 2
Max: 4
Range: 2
The Binomial and Multinomial Distributions
Binomial
– when you have multiple trials that either end in a
success or failure – only 2 outcomes such as heads or tails or
life or death.
-
commonly used
For example, if you wanted a pink poodle, then it would be
considered a success and all other outcomes would be
considered failures.
Another example would be taking allergy medication. If
getting relief from your Sx is a ‘success,’ then every time
medication is taken becomes a binomial trial, and the
‘success’ probability may be something like 0.8.
Or when running a red light, avoiding a citation would be
defined as ‘success’ then every time you run a red light is a
binomial trial, and the probability of success may be
something like 0.95.
In sports like taking a shot on the basketball court, making the
shot is defined a ’success,’ making every time I shoot a
binomial trial, and the probability of success may be
something like 0.4.
Or when the quarterback throws a pass, then his or her
completion would be defined as a ‘success,’ thus making
every attempt of the quarterback a binomial trial, and the
probability of success may be something like 0.6.
Recoding Multiple Outcomes to be Binomial
If there are more than 2 possible outcomes, you can easily
define a single outcome as ‘success’ and any other outcome
as ‘failure.’
-
rolling a 6-sided die: if rolling a 5 is ‘success’, then each
roll is a binomial trial with a probability of success equal
to 0.167.
-
election polling: if a poll response of “republican’ is
defined as ‘success’, then each time someone answers
the poll is a binomial trial, and the probability of success
might be something like 0.41.
Recoding Quantitative Data to be Categorical and Binomial
If the response is quantitative rather than categorical, you can
still use binomial distribution to model the process:
-
looking up the salary of a state employee: If “success”
is defined as a salary greater than $45K per year, then
each time a salary is observed is a binomial trial. The
probability of success might be something like 0.55.
Multinomial
– if you didn’t want to limit your choices to just
two, you can have three or more outcomes. All four poodle
colors in the analysis can be used instead of breaking them
into just pink or non-pink categories.
-
based on categorical outcomes
Compute
a single
Single Sample t-Tests
-
similar to single sample z-test
Your preview ends here
Eager to read complete document? Join bartleby learn and gain access to the full version
- Access to all documents
- Unlimited textbook solutions
- 24/7 expert homework help
sample,
dependen
t, and
independe
nt t test in
MS Excel
-
instead of testing against the normal distribution, it
uses the student’s t-distribution, which looks a lot like
the normal distribution and looks more alike as the
sample size gets bigger
-
eventually with a large enough sample size, it may look
like a normal distribution
-
both the z-test and the single sample t-test compare a
score to the population
-
Another difference between normal distribution and the
t distribution is that the t distribution adds another
parameter such as the
degrees of freedom (df)
How does the distribution shape change with
sample size?
df = n – 1
Although different analyses have different ways to calculate
df, they will all depend on n, the sample size.
Example: Hobbit Movie Rating Example
x̅ = 4.25; µ = 4.00
difference was so
large
because the n, or sample size was
10,000. That
displays such a significant
difference. The n is
sometimes disguised in
the degrees of freedom.
µ = population mean
s = sample standard deviation
n = sample size
x̅ = individual score
The Student’s t-Distribution
In the early 1900s, a man named William Gosset- worked at
the Guinness brewery in Dublin. He was exploring how to
make determinations about populations with sample sizes
that could be quite small. One of the things he was looking
into was chemical properties in barley when the sample size
was as small as 3. Even though he didn’t invent the method,
he published his findings in
Biometrika
. He used the
pseudonym ‘student.’ This is why his work has gone under the
name “Student’s t.”
-
a good way to determine probabilities for normally
distributed populations where the population standard
deviation (sigma =σ) is unknown.
In order to determine probabilities, one more parameter
needs to be explained. There is this thing called
degrees of
freedom
for the t-distribution. Degrees of freedom will always
be associated with the sample size. For the t-distribution, if
the sample size is
n
, then the degrees of freedom is (
n
-1).
Below is the t-distribution for 3 degrees of freedom (df):
Below is the t-distribution for 15 df:
I note that the t-distribution is very similar to the normal
distribution. The normal distribution is overlaid on each of the
above graphs of the t-distribution, in a light grey color. As the
df increases, the t-distribution looks more and more like the
normal distribution, to the point that at 30 or more df, the t-
distribution and the normal distribution are indistinguishable.
The t-distribution is useful for determining probabilities when
sigma is unknown. If you have a situation where you think the
population mean is mu, and you take a sample of size
n
from
that population, you can then calculate what is often called
the
t-score.
There are
4 variables
in this equation.
There is the population mean,
There is the size of the sample used, n
There is the sample standard deviation,
s
There is the sample mean,
x̅
This equation looks similar to the z-
score equation used in the previous lesson, and it is. In
general, the main difference is that the z-score is used when
sigma is known
, and the t-score is used when sigma is not
known.
Calculating a Single Sample t Test
Suppose there is a population of some manufactured product,
say a widget. The plant manager wants you to test the widget
for warping and wants to know at what temperature warping
begins. She says it needs to be able to run in a hot
environment, say 280 degrees, so she wants to assume that
warping doesn’t begin until 305 degrees in order for there to
be some buffer.
You have the necessary equipment, and you begin to test the
widgets. She says you can only use 7 widgets for testing,
because they will have to be scrapped and cannot be sold.
You select the 7 widgets and test them. The data you collect
for beginning for warpage is as follows:
302.7
These values are plugged into a spreadsheet, and
then use
295.8
the spreadsheet to calculate the mean and the
standard
306.3
deviation, as follows:
289.7
301.9
You now have all you need, so plug
these
297.0
values into the equation for
t
:
299.7
t
=
299.01
−
305
5.4254
√
7
=−
2.92
Now that t has been calculated, determine the probability
associated with that
t
. Use this applet for the
t
probability.
Plug the numbers in after making sure that only the left tail is
highlighted in green. Then at the bottom left, enter -2.92.
Last, go to the top where it asks for degrees of freedom. Since
your sample size is 7, then you have 6 df (7-1 = 6). Plug a 6
into that spot. And the applet should appear like this:
Your preview ends here
Eager to read complete document? Join bartleby learn and gain access to the full version
- Access to all documents
- Unlimited textbook solutions
- 24/7 expert homework help
The probability associated with these values is 0.0133, which
mean that if you assume the population of widgets has a
‘warpage’ point of 305 degrees, then the probability that you
would get a
t
= -2.92 or lower (which is why I highlighted the
left tail) is 0.0133. In other words, it is not likely. It is more
likely that my assumption that the average warpage begins at
305 degrees is wrong.
Dependent t-Tests
Paired Data
Paired vs Independent
Data is paired, or dependent, when they are linked together in
some way. For example, if I have the same person’s data at
different time points, they are linked together by the person.
If, for each experimental unit in the first sample, there is a
corresponding experimental unit of the second example, then
the samples are probably paired. Here are some examples of
paired data:
-
pre- and post-scores are taken before and after a new
training program. The pre- and post- scores for an
individual are paired.
-
BP is measured before and after Tx for several pts. The
before and after measurements for each patient are
paired.
-
Several adults are given 2 different exams covering the
same material. The test proctor is trying to determine if
the tests are essentially the same level of difficulty. The
two test scores for each individual are paired.
-
2 different brands of bicycle tires are being compared to
see if one of them wear better than the other. Several
bikes are equipped with one tire from each brand, and
they are given to subjects to ride for 3 months, and the
amount of wear is measured for each tire. Each
individual bike will produce two measurements, one for
each tire. These two measurements for each bike will be
paired.
Calculating the dependent t-test by hand
Dependent t-test equation
It can be read as d bar minus 0 divided by the standard
deviation of d bar.
D bar is the mean of the difference between the first and
second score for each count. The S d bar is the standard error
of the differences between the first and second score.
Standard Error of the Difference Equation
Calculating the Standard Error of the Difference
S
D̅
= 59.23 / sqrt(9) = 59.23 / 3 = 19.74
Calculating the Dependent t-Test
t = -5.61 – 0 / 19.74 = -0.28
The smaller the t value, the less likely it would be significant
When the df of 8, and the t value 0.28 are inserted into the
Student’s t Probabilities applet, the p value is 0.7866, which is
higher than 0.05. This means that I must accept the null
hypothesis, which means that there is no difference between
cats drinking water out of the bowl and cats drinking water
out of the faucet.
So why do you care if the samples are paired? When
comparing two samples, you are trying to see if the amount of
variation sample to sample is big enough to call them
“different.” If there is a pairing that can be identified, the
‘pair-to-pair’ variation can be removed from the analysis.
Anytime you can eliminate one or more sources of variation,
your analysis becomes more powerful and more accurate.
Calculating Dependent t- Tests in MS Excel
Independent t-Tests
Data is independent if they do not relate to each other. For
instance, if you are testing two different weight loss programs,
but the programs are composed of completely different
people.
1. Hypotheses:
-
Null – the true mean difference is equal to zero
H
0
:
D
̅
= 0
-
Alternative – the true mean difference does not equal
zero
(H
a
:
D
̅
≠
0).
-
In the hypothesis test, the variable representing the
difference is
D
, pronounced “d bar.”
̅
Example
-
Children who helped prepare the meal vs children who
did not help prepare the meal
-
Not siblings or in any way related
-
2-tailed hypothesis
-
Null hypothesis: No difference between groups on
calorie intake
-
Alternative: Groups differ on calorie intake
Two samples are independent if the participants in group 1 tell
you nothing about the participants in group 2. They do not
consist of the same people, and they are not paired in any
way. I will conduct hypothesis tests to determine whether the
means of each group differ
(µ
1
– u
2
).
We can skip finding the
difference step.
Your preview ends here
Eager to read complete document? Join bartleby learn and gain access to the full version
- Access to all documents
- Unlimited textbook solutions
- 24/7 expert homework help
Case Study: Concussion Rates in Male and Female
Athletes
State the Null and Alternative Hypotheses and the
Level of Significance
The null hypothesis is that there is no difference in the
number of concussions for men and women.
H
0
: μ
1
= μ
2
The alternative hypothesis is that men and women will have
different rates of concussion.
H
a
: μ
1
≠ μ
2
The alpha is 0.05 level of significance.
Give the relevant summary statistics
I will use x bar1 to denote the mean number of concussions
for men, s1 for the male standard deviation, and n1 for the
male concussion sample size. For women, you will use x-bar2,
s2, and n2, respectively.
Test for Assumptions
For an independent t test to be accurate, the data must be
normally distributed for each group. I must examine the
groups separately otherwise it would obscure any differences
between the groups.
Neither of them look normally distributed! No bell-shaped
curve here.
Give the Test Statistic and its Value
The test statistic for a hypothesis test comparing two means
with independent samples is a t. The calculation for the two
sample t-test is not trivial. It can be calculated by hand, but in
trying to be consistent with the goal of keeping calculations
simple and to a minimum, you will utilize the pre-packaged
functions in MS Excel.
Simply use =t.test( ) however, instead of choosing option 1
for a paired test at the end choose option 3, for an
independent test with unequal variance. The calculation for t
is slightly different depending on whether or not the variances
between two samples are assumed to be equal. Since you do
not know anything about the population standard deviation or
variance for the two samples, it doesn’t seem reasonable to
assume they are equal. However, if you assume they are
unequal and they are equal, then the two formulas converge
to the same value. For this reason, take the conservative
approach and always assume the variances are unequal—or
heteroscedastic.
Since your alternative hypothesis contains the
≠
sign, you
have a two-sided t-test.
State your Decision
Now, you will apply the
p
value MS Excel spit out for you.
Since the
p
-val is greater than the level of significance, you
will fail to reject the null hypothesis:
0.58 > 0.05
Present your Conclusion in a Sentence, Relating the
Result to the Context of the Problem
There is insufficient evidence to suggest that there is a
difference in the number of concussions between male and
female college athletes.
or
Male and female athletes get the same number of
concussions in college.
Understan
d the
importanc
e of effect
size
Once you have a t-score and corresponding p value, you may
also want to calculate the effect size. More robust and
accurate in determining whether a test is meaningful or not
because formula does not include sample size as used in
degrees in freedom to calculate significance.
Cohen’s D Formula – mean of the difference scores over the
standard deviation of the difference scores; measures the
proportion of variance in the dependent variable that is
accounted for by the independent variable.
Your preview ends here
Eager to read complete document? Join bartleby learn and gain access to the full version
- Access to all documents
- Unlimited textbook solutions
- 24/7 expert homework help
Plug in the values: -5.61 / 59.23 = -0.95
Effect Size
Small less than or equal to 0.2
Medium 0.3 – 0.5
Large greater than or equal to 0.6
a better indicator than
p
value of how strong the findings of a
particular test are, because
p
value depends on sample size
via the degrees of freedom, but effect size does not. You can
calculate effect size using a measure called Cohen’s D.
Learn
about the
F-
Distributi
on and
the role it
plays in
ANOVAs
The F Distribution
For what is the F Distribution Used?
-
analysis of variance (ANOVAs)
-
Regressions – modeling
-
Data comparing more than 2 groups
Compares one group to another, 2 or more groups
More skewed than t test distribution; its shape is determined
by the degrees of freedom, m and n
Does not approximate the normal distribution
The n and the m in the figure correspond to the two values of
degrees of freedom. Please note from looking at this graph
that a value for F less than 0 is impossible.
The “peak” of the distribution is usually around 1, and the
distribution goes on forever to the right side. Much like the
normal distribution, the right side the curve never actually
touches the horizontal axis, but gets closer as it gets farther
out.
-
Related Documents
Recommended textbooks for you
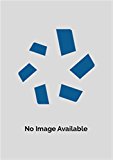
Big Ideas Math A Bridge To Success Algebra 1: Stu...
Algebra
ISBN:9781680331141
Author:HOUGHTON MIFFLIN HARCOURT
Publisher:Houghton Mifflin Harcourt
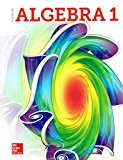
Glencoe Algebra 1, Student Edition, 9780079039897...
Algebra
ISBN:9780079039897
Author:Carter
Publisher:McGraw Hill
Algebra & Trigonometry with Analytic Geometry
Algebra
ISBN:9781133382119
Author:Swokowski
Publisher:Cengage
Recommended textbooks for you
- Big Ideas Math A Bridge To Success Algebra 1: Stu...AlgebraISBN:9781680331141Author:HOUGHTON MIFFLIN HARCOURTPublisher:Houghton Mifflin HarcourtGlencoe Algebra 1, Student Edition, 9780079039897...AlgebraISBN:9780079039897Author:CarterPublisher:McGraw HillAlgebra & Trigonometry with Analytic GeometryAlgebraISBN:9781133382119Author:SwokowskiPublisher:Cengage
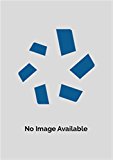
Big Ideas Math A Bridge To Success Algebra 1: Stu...
Algebra
ISBN:9781680331141
Author:HOUGHTON MIFFLIN HARCOURT
Publisher:Houghton Mifflin Harcourt
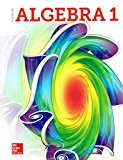
Glencoe Algebra 1, Student Edition, 9780079039897...
Algebra
ISBN:9780079039897
Author:Carter
Publisher:McGraw Hill
Algebra & Trigonometry with Analytic Geometry
Algebra
ISBN:9781133382119
Author:Swokowski
Publisher:Cengage