Practice Midterm
docx
keyboard_arrow_up
School
Harvard University *
*We aren’t endorsed by this school
Course
E150
Subject
Statistics
Date
Jan 9, 2024
Type
docx
Pages
10
Uploaded by mzaid0001
Midterm Questions
1.
What is the difference between independent and dependent variables? An independent variable is something that can be predicted or manipulated. The dependent variable is the expected outcome and is measured. 2.
In the basic statistical model Y
= f(X) = e, what do each of these symbols represent?
The “Y” stands for the outcome or true y, the “f” embodies the function used in the calculation or predicted y, and the “X” represents the input or inputs used for the formula.
“e” stands for error.
3.
Please explain the null and alternative hypothesis. You may use an example of an experiment to help explain if you like. In statistical hypothesis testing, the null hypothesis of a test always predicts no effect or no relationship between variables, while the alternative hypothesis states
your research prediction of an effect or relationship.
H0: The null hypothesis: It is a statement of no difference between sample means or proportions or no difference between a sample mean or proportion and a population mean
or proportion. In other words, the difference equals 0.
Ha: The alternative hypothesis: It is a claim about the population that is contradictory to H0 and what we conclude when we reject H0.
A medical trial is conducted to test whether or not a new medicine reduces cholesterol by 25%. State the null and alternative hypotheses.
H0: The new medicine reduced cholesterol by less than 25% in patients with high cholesterol who participated in this study. p ≤ 25
Ha: The new medication reduced cholesterol by more than 25% in patients with high cholesterol who participated in this study. p > 25
4.
List your own string of 11 ordered data points (any data points are fine) below and identify the 1) range of scores; 2) median; 3) quartiles - and name them; 4) Interquartile range.
Here are the scores of 11 students on an history test 59, 62, 65, 72, 74, 76, 77, 80, 80, 86, 89 N=11
Minimum: 59
Maximum: 89
Lower quartile (Q1)= 1/4 [n+1] observation in ascending order (Q1)= [n+1/4]th observation in ascending order (Q1)= [11+1/4] observation in ascending order (Q1)= [3]th
[Q1]=3
rd
observation + [4
th
obs^n -3
rd
obs^n]
Q1= 65 [72-65]
Q1=72 Lower quartile=72 Upper quartile=3/4 [n+1]th obs^n=3/4[11+1]^th
Q3=9
th
observation in ascending order Q3=80+86/2 average of 10
th
and 11
th
observation Q3=83 Upper quartile=83
Median = [n+1/2] obs^n = 11=1/2= 6
Median=6
th
observation in ascending order Median =76
Inquartile range = Q3-Q1= 83-72=11
Inquartile range = 11
5.
As a sample size gets larger the standard error will get smaller. Why is this? The size (n) of a statistical sample affects the standard error for that sample. Because n is in the denominator of the standard error formula, the standard error decreases as n increases. Basically, the more data you collect the less the variation will be and the less likelihood for error. 6.
Please respond to the following questions:
a.
Why do we find it necessary for our studies to have a good amount of power?
There should be enough Statistical Power to ensure that we find differences if they are there.
Statistical Power is determined by several factors, most importantly the size of the statistical significance selected, the size of the effect (amount of difference) we are expecting and the sample size. Since we typically use significance levels of .05 or .
01 and we do not know the size of the effect in advance, we are often left with having
to make decisions about sample size when planning a study to achieve sufficient
Statistical Power. In short, we need to make sure that we have enough study participants to statistically detect the statistical differences observed.
b.
If we set our power at .8 as generally recommended, what is our corresponding probability of failing to detect a genuine effect, and how do you know this? There is a .2 probability of failing to detect a genuine effect. We know this because the power is set to .8 and is therefore telling us that there is a 80% chance
of not failing to detect a genuine effect. 7.
We know that in linear models statistical bias, biases parameter estimates. But how does a biased parameter estimate then bias standard errors and confidence intervals? Please explain the process, including a discussion around sums of squares.
A biased parameter estimates bias standard errors and confidence intervals by assessing things such as assumptions and outliers. . The presence of an outlier, pushes the curve to the right (i.e., it makes the mean higher) and pushes it upwards too (i.e., it makes the sum of squared error larger). By comparing how far the curves shift horizontally compared to vertically you should get a clear sense that the outlier affects the sum of squared error more dramatically than it affects the parameter estimate itself. This is because we use squared errors, so any bias created by the outlier is magnified by the fact that deviations are squared.
Therefore, if the sum of squared errors is biased, so are the standard error and the confidence intervals associated with the parameter estimate. In addition, most test statistics are based on sums of squares so these will be biased too by outliers.
8.
What is the difference between Type 1 and Type 2 error? Please give an example of each.
Type I error means rejecting the null hypothesis when it's actually true, while a Type 2 error means failing to reject the null hypothesis when it's actually false. Type I errors can
be controlled. The value of alpha, which is related to the level of significance that we selected has a direct bearing on type I errors. Alpha is the maximum probability that we have a type I error. For a 95% confidence level, the value of alpha is 0.05. This means that there is a 5% probability that we will reject a true null hypothesis. In the long run,
one out of every twenty hypothesis tests that we perform at this level will result in a type I error.
Examples: Type I error (false positive): the test result says you have diabetes, but you actually don’t.
Type II error (false negative): the test result says you don’t have diabetes, but you actually do.
Your preview ends here
Eager to read complete document? Join bartleby learn and gain access to the full version
- Access to all documents
- Unlimited textbook solutions
- 24/7 expert homework help
9.
What assumption does the dataset clearly not meet in Plot 1 below? How do you know?
This data clearly does not meet the assumption of heteroscedasticity. This can be inferred because the data set shows a situation in which the assumptions of linearity and homoscedasticity
have been met. What about the dataset in Plot 2 below? What two assumptions did the dataset in Plot 2 not meet? How do you know that the dataset did not meet these assumptions?
There is a non-linear relationship between
the outcome and the predictor: there
is a clear curve in the residuals
10. A researcher was interested in what factors influence people’s fear responses to horror films. She measured gender and how much a person is prone to believe in things that are not real (fantasy proneness) on a scale from 0 to 4 (0 = not at all fantasy prone, 4 = very fantasy prone). Fear responses were measured on a scale from 0 (not at all scared) to 15 (the most scared I have ever felt). What is the theroretical model equation? A.
Research question: Does gender and proneness to believe in fantasy influence peoples
fear responses to horror films? B.
Model Equation Response to horror films = BO + B1* gender, fantasy proneness + error
11. Please refer to Table 1 below when reading and responding to this question. A researcher was interested in what factors influence people’s fear responses to horror films. She measured gender and how much a person is prone to believe in things that are not real (fantasy proneness) on a scale from 0 to 4 (0 = not at all fantasy prone, 4 = very fantasy prone). Fear responses were measured on a scale from 0 (not at all scared) to 15 (the most scared I have ever felt). What assumption is the Durbin-Watson statistic testing and
what does it tell us?
The Durbin Watson test reports a test statistic, with a value from 0 to 4, where:
2 is no autocorrelation.
0 to <2 is positive autocorrelation (common in time series data).
>2 to 4 is negative autocorrelation (less common in time series data).
In the above table The Durbin Watson statistic is .295 which falls between 0 to >2. Therefore, there is a positive autocorrelation between gender, fantasy proneness and feeling fear after watching a horror film 12. Recent research has shown that lecturers are among the most stressed workers. A researcher wanted to know exactly what it was about being a lecturer that created this stress and subsequent burnout. She recruited 75 lecturers and administered several questionnaires that measured: Burnout
(high score = burnt out), Perceived Control
(high score = low
perceived control), Coping Ability
(high score = low
ability to cope with stress), Stress from Teaching
(high score = teaching creates a lot of stress for the person), Stress from Research
(high score = research creates a lot of stress for the person), and Stress from Providing Pastoral Care
(high score = providing pastoral care creates a lot of stress for the person). The outcome of interest was burnout, and Cooper’s (1988) model of stress indicates that perceived control and coping style are important predictors of this variable. The remaining predictors were measured to see the unique contribution of different aspects of a lecturer’s work to their burnout. Please refer to Table 2 below to in responding to the following question: How much variance in burnout does the final model explain for the sample? R3=0.803= 80.3%
80.3 % of the varaition in burnout is shared by the third and final model in the population
13. How would you interpret the beta value for ‘stress from teaching’ in the final model (model 3)?
The regression coefficient of Stress from teaching is -0.360 This can be interpreted as for every unit increase in the stress from teaching , the burnout score decreases by 0.360 units.
14. Let’s say we wanted to look at an additional variable in the example above, years of teaching experience. Our analysis indicates that the z-score for skewness is -1.45 and the z-score for kurtosis is 2.39. Should we be concerned about these values? Are they significant and if so, at which p
-level?
The -1.45 z-score for skewness indicates a positively skewed distribution since it is >0.
2.39 kurtosis is within the normal range The are significant at a p-level of 0. 15. We learned that on average, samples from a normally distributed population will have skew/kurtosis of 0.
a.
Please convert the values of skewness and kurtosis in Table below (Table 4) to a test of whether the values are significantly different from 0 using z
-scores. Please
Your preview ends here
Eager to read complete document? Join bartleby learn and gain access to the full version
- Access to all documents
- Unlimited textbook solutions
- 24/7 expert homework help
convert negative scores into absolute values of those scores. Make sure to show your work by inserting the appropriate equations and corresponding numbers.
b.
Please interpret your equations using complete sentences.
Skewness= -.482 kurtosis=-.750
1.
Skewness test
z-test: -.482-0/0.172=-2.8023
p-value: P(z<-
2.8023) * 2=0.005<0.05. The null is rejected and we can conclude that there is significant evidence that the skewness is different from zero.
2.
Kurtosis Test Ho kurtosis=0 Ha kurtosis does not =0
z-test: -.750-0/.342= -2.192
p-value P(z< -2.192) * 2= 0.0283 <0.05. The null is rejected and we can conclude that the skewness is different from 0. 16. Please interpret Levene’s Test below.
Levene’s test is to test the equality of variances for two or more groups. It tests the null hypothesis, that the population variances are equal. If, the resulting P_value of Levene's test is less than some significance level ( like 0.05, 0.01, 0.1) , it means that the obtained differences in sample variances are unlikely to have occurred based on random sampling from a population with equal variances.
For the given level of significance 0.05, in this table the significant value for all rows are greater than 0.05, so we can conclude that the population variances are equal for all groups based on Mean, Median, Median and with adjusted df and trimmed mean.
17. A frequency distribution in which there are too few scores at the extremes of the distribution is said to be what? Platykurtic
18. In the regression models we’ve gone over so far, what does B0 represent? b0 is the intercept of the regression line; that is the predicted value when x = 0
19. Your textbook covers a lot of different ways to transform biased data, but what is a major problem with transforming data?
When data (or residuals) are not normal, the non-normality is often an important part of the research. If you transform it away, you are throwing away that critical piece.
20. A salesperson for a large car brand wants to determine whether there is a relationship between an individual's income and the price they pay for a car. As such, the individual's "income" is the predictor variable and the "price" they pay for a car is the outcome variable. The salesperson wants to use this information to determine which cars to offer potential customers in new areas where average income is known. He runs a Regression analysis. Given this information, please interpret R and R
2
using Table 6 below. Please write in complete sentences, making sure to accurately indicate what both R and R
2 mean.
R is the correlation between the predicted values and the observed values of Y. In the above table the R reveals that there is 87% correlation between the income of a person and the price they pay for a car. The most common interpretation of r-squared is how well the regression model fits the observed data. For example, in the table above there is an r-squared of 76%. This reveals that 76% of the data fit the regression model. Generally, a higher r-squared indicates a better fit for the model.
However, it is not always the case that a high r-squared is good for the regression model. The quality of the statistical measure depends on many factors, such as the nature of the variables employed in the model, the units of measure of the variables, and the applied data transformation. Thus, sometimes, a high r-squared can indicate the problems with the regression model.
A low r-squared figure is generally a bad sign for predictive models. However, in some cases, a good model may show a small value. There is no universal rule on how to incorporate the statistical measure in assessing a model. The context of the experiment or forecast is extremely important and, in different scenarios, the insights from the metric can vary.
21. Please refer to Table 7 below (Output from the same Regression Analysis run to produce Table 6) when reading and responding to the following questions: a.
It is clear that the degrees of freedom for SS
M is 1, and the degrees of freedom for SS
R
is 18. How did we get to these numbers? More specifically, what is the formula for the degrees of freedom for SS
M
and SS
R
?
SSM = Σi=1n (yi^ - y)2
SSR=
R-squared = SSR / SST
The degrees of freedom associated with SSR will always be 1 for the simple linear regression model. b.
How did we come to the outcome of 44182633.37 for the Regression Sum of Squares (SS
M
)?
SSM=1(.873-874.779)^2
Your preview ends here
Eager to read complete document? Join bartleby learn and gain access to the full version
- Access to all documents
- Unlimited textbook solutions
- 24/7 expert homework help
c.
What does significance in this table mean?
The significance score based on the p value and since it is less than the 0.05 it means that the analysis conducted is significant and the independent factor affects the dependent variable . This means that price is dependent on Income.
Related Documents
Recommended textbooks for you
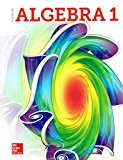
Glencoe Algebra 1, Student Edition, 9780079039897...
Algebra
ISBN:9780079039897
Author:Carter
Publisher:McGraw Hill
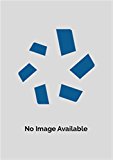
Big Ideas Math A Bridge To Success Algebra 1: Stu...
Algebra
ISBN:9781680331141
Author:HOUGHTON MIFFLIN HARCOURT
Publisher:Houghton Mifflin Harcourt
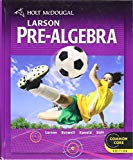
Holt Mcdougal Larson Pre-algebra: Student Edition...
Algebra
ISBN:9780547587776
Author:HOLT MCDOUGAL
Publisher:HOLT MCDOUGAL
Recommended textbooks for you
- Glencoe Algebra 1, Student Edition, 9780079039897...AlgebraISBN:9780079039897Author:CarterPublisher:McGraw HillBig Ideas Math A Bridge To Success Algebra 1: Stu...AlgebraISBN:9781680331141Author:HOUGHTON MIFFLIN HARCOURTPublisher:Houghton Mifflin HarcourtHolt Mcdougal Larson Pre-algebra: Student Edition...AlgebraISBN:9780547587776Author:HOLT MCDOUGALPublisher:HOLT MCDOUGAL
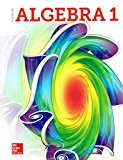
Glencoe Algebra 1, Student Edition, 9780079039897...
Algebra
ISBN:9780079039897
Author:Carter
Publisher:McGraw Hill
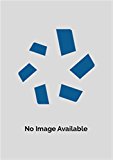
Big Ideas Math A Bridge To Success Algebra 1: Stu...
Algebra
ISBN:9781680331141
Author:HOUGHTON MIFFLIN HARCOURT
Publisher:Houghton Mifflin Harcourt
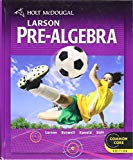
Holt Mcdougal Larson Pre-algebra: Student Edition...
Algebra
ISBN:9780547587776
Author:HOLT MCDOUGAL
Publisher:HOLT MCDOUGAL