Statistic Project
docx
keyboard_arrow_up
School
University of Ottawa *
*We aren’t endorsed by this school
Course
CCNA3
Subject
Statistics
Date
Feb 20, 2024
Type
docx
Pages
37
Uploaded by stanleypo0802
Statistic Project – Trends in Major League Baseball
What is baseball?
Baseball is a game played with a bat and ball between 2 teams of 9 players each, while one team plays defence (fielders), the other team plays offence (batters)
. The object is to score runs by advancing players counter-clockwise around 4 bases. An offending player tries to hit the ball away from the reach of the defenders and score runs by running around the bases. Players of the defending team try to out the player who is batting.
There are nine standard
positions for the defensive team
in baseball, including pitcher (P), catcher (C), first base (1B), second base(2B), shortstop (SS), third base(3B), right field (RF), center field (CF), and left field(LF).
Investigation(outline) and hypothesis
I plan to investigate the relationship between Home Runs per game (HR/G) and Runs Batted In per game (RBI/G). I’m investigating this relationship for chosen decades(1920s ~ 2010s), positions( First Base, outfielder, shortstop), first and third quartile of Hits, and first and third quartile of Base on Balls (BB). I hypothesize that the Run Batted In per game will increase as the
Home Run per game increases (strong positive correlation). Definitions
Home Run per game (HR/G)
A home run
occurs when a batter hits a fair ball and scores on the play without being put out or without the benefit of an error
.
Runs Batted In per game (RBI/G)
a statistic credited to a batter whose action at bat causes one or more runs to score
First Base (FB)
the player on a
baseball
or
softball
team who fields the area nearest first base, the first of four bases a baserunner must touch in succession to score a run.
Outfielder (OF)
a player who is positioned at one of the three outfield defensive positions in baseball, farthest from the batter
Shortstop (SS)
the player position in baseball for defending the infield area on the third-base side of second base
Mean
the average of a set of values
.
Median
the middle number in a sorted, ascending or descending list of numbers
Quartiles (1
st
and 3
rd
)
type of quantile which divides the number of data points into four parts, or quarters, of more-or-less equal size
.
Base on Balls (BB)
An advance to first base given to a baseball batter who takes four pitches that are balls
.
Hits (H)
A hit
occurs when a batter strikes the baseball into fair territory and reaches base without doing so via an error or a fielder's choice
.
Correlation coefficient (R)
statistical measure of the strength of a linear relationship. Coefficient of determination (R
2
)
a number between 0 and 1 that measures how well a statistical model predicts an outcome
.
Scatterplots for All Data
Single Variable statistic
Home Runs (HR)
Runs Batted In (RBI)
Mean
16.2313237
73.9076016
Median
14
72
Correlation Coefficient (R)
0.7825
Coefficient of Determination (R
2
)
0.6123
Due to the fact that not every player played the same numbers of games, the data will not accurately reflect player’s actual performance and bias may be presented. In order to solve this, I standardized the data by comparing Home Run per game and Runs Batted In per game.
y = 1.725x + 45.909
R² = 0.6123
0
20
40
60
80
100
120
140
160
180
200
0
10
20
30
40
50
60
70
RBI
HR
HR v.s RBI
Scatterplot for all data (standardized)
Single Variable statistic
Home Runs per game (HR/G)
Runs Batted In per game (RBI/G)
Mean
0.110703
0.506843
Median
0.1
0.5
Correlation Coefficient (R)
0.7618
Coefficient of Determination (R
2
)
0.5803
The line of best fit representing the data is y = 1.6189x + 0.3276. The slope (m) of the line of best fit is 1.6189, indicating the increase of 1.6189 runs batted per game for every home run per game. The y-intercept, representing the number of runs batted in per game if there are no home runs, is 0.3276. There is a strong, positive linear correlation since the R value is approximately 0.7618, which is between 0.67 and 1. y = 1.6189x + 0.3276
R² = 0.5803
0
0.2
0.4
0.6
0.8
1
1.2
1.4
0
0.05
0.1
0.15
0.2
0.25
0.3
0.35
0.4
0.45
RBI/G
HR/G
HR/G v.s RBI/G ( standardized)
Your preview ends here
Eager to read complete document? Join bartleby learn and gain access to the full version
- Access to all documents
- Unlimited textbook solutions
- 24/7 expert homework help
Decades (1920s~2010s)
Scatterplot for the 20s
Single Variable statistic
Home Runs per game (HR/G)
Runs Batted In per game ( RBI/G)
Mean
0.056621
0.53417
Median
0.038611
0.517989
Correlation Coefficient (R)
0.7174
Coefficient of Determination (R
2
)
0.5146
The line of best fit representing the data is y = 2.0022x + 0.4208. The slope (m) of the line of best fit is 2.0022, indicating the increase of 2.0022 runs batted per game for every home run per game. The y-intercept, representing the number of runs batted in per game if there are no home runs, is 0.4208. There is a strong, positive linear correlation since the R value is approximately 0.7174, which is between 0.67 and 1. y = 2.0022x + 0.4208
R² = 0.5146
0
0.2
0.4
0.6
0.8
1
1.2
1.4
0
0.05
0.1
0.15
0.2
0.25
0.3
0.35
0.4
0.45
RBI
HR
HR v.s RBI for 20s
Scatterplot for the 30s
Single Variable statistic
Home Runs per game (HR/G)
Runs Batted In per game (RBI/G)
Mean
0.074129
0.533818
Median
0.054688
0.503876
Correlation Coefficient (R)
0.8318
Coefficient of Determination (R
2
)
0.6919
The line of best fit representing the data is y = 2.3454x + 0.36. The slope (m) of the line of best fit is 2.3454, indicating the increase of 2.3454 runs batted per game for every home run per game. The y-intercept, representing the number of runs batted in per game if there are no home runs, is 0.36. There is a strong, positive linear correlation since the R value is approximately 0.8318, which is between 0.67 and 1. Comparing to the previous decade, both mean and median has increased for the home run per game. However, the mean and median for the runs batted in per game has dropped slightly. The correlation coefficient also increased, indicating a strengthening linear correlation. y = 2.3454x + 0.36
R² = 0.6919
0
0.2
0.4
0.6
0.8
1
1.2
1.4
0
0.05
0.1
0.15
0.2
0.25
0.3
0.35
RBI
HR
HR v.s RBI for 30s
Scatterplot for the 40s
Single Variable statistic
Home Runs per game (HR/G)
Runs Batted In per game (RBI/G)
Mean
0.072061
0.461848
Median
0.055944
0.462585
Correlation Coefficient (R)
0.7732
Coefficient of Determination (R
2
)
0.5979
The line of best fit representing the data is y = 1.6928x + 0.3399. The slope (m) of the line of best fit is 1.6928, indicating the increase of 1.6928 runs batted per game for every home run per game. The y-intercept, representing the number of runs batted in per game if there are no home runs, is 0.3399. There is a strong, positive linear correlation since the R value is approximately 0.7732, which is between 0.67 and 1. Comparing to the previous decade, both mean and median for the runs batted in per game has dropped. The mean for the home run per game has increased slightly, but the median for the home run per game decreases. The correlation coefficient also decreased, showing a weakening linear correlation.
y = 1.6928x + 0.3399
R² = 0.5979
0
0.1
0.2
0.3
0.4
0.5
0.6
0.7
0.8
0.9
1
0
0.05
0.1
0.15
0.2
0.25
0.3
0.35
0.4
RBI
HR
HR v.s RBI for 40s
Your preview ends here
Eager to read complete document? Join bartleby learn and gain access to the full version
- Access to all documents
- Unlimited textbook solutions
- 24/7 expert homework help
Scatterplot for the 50s
Single Variable statistic
Home Runs per game (HR/G)
Runs Batted In per game (RBI/G)
Mean
0.117755
0.520435
Median
0.107438
0.509554
Correlation Coefficient (R)
0.7951
Coefficient of Determination (R
2
)
0.6322
y = 1.7267x + 0.3171
R² = 0.6322
0
0.1
0.2
0.3
0.4
0.5
0.6
0.7
0.8
0.9
1
0
0.05
0.1
0.15
0.2
0.25
0.3
0.35
RBI
HR
HR v.s RBI for 50s
The line of best fit representing the data is y = 1.7267x + 0.3171. The slope (m) of the line of best fit is 1.7267, indicating the increase of 1.7267 runs batted per game for every home run per game. The y-intercept, representing the number of runs batted in per game if there are no home runs, is 0.3171. There is a strong, positive linear correlation since the R value is approximately 0.7951, which is between 0.67 and 1. Comparing to the previous decade, both mean and median for the home runs per game has increased. The mean and median for the runs batted in per game also increased slightly. The correlation coefficient also rises, indicating a strengthening linear correlation.
Scatterplot for the 60s
Single Variable statistic
Home Runs per game (HR/G)
Runs Batted In per game (RBI/G)
Mean
0.10938
0.447479
Median
0.097744
0.428571
Correlation Coefficient (R)
0.8760
Coefficient of Determination (R
2
)
0.7674
The line of best fit representing the data is y = 1.7704x + 0.2538. The slope (m) of the line of best fit is 1.7704, indicating the increase of 1.7704 runs batted per game for every home run per game. The y-intercept, representing the number of runs batted in per game if there are no home runs, is 0.2538. There is a strong, positive linear correlation since the R value is approximately 0.8760, which is between 0.67 and 1. Comparing to the previous decade, both the mean and y = 1.7704x + 0.2538
R² = 0.7674
0
0.1
0.2
0.3
0.4
0.5
0.6
0.7
0.8
0.9
0
0.05
0.1
0.15
0.2
0.25
0.3
0.35
RBI
HR
HR v.s RBI for 60s
median for the home run per game decreased slightly, as well as the mean and median for the runs batted in per game. However, the correlation coefficient has increased considerably, from 0.7951 to 0.8760, showing a strengthening linear correlation
Scatterplot for the 70s
Single Variable statistic
Home Runs per game (HR/G)
Runs Batted In per game (RBI/G)
Mean
0.107814
0.480483
Median
0.106772
0.489508
Correlation Coefficient (R)
0.8679
Coefficient of Determination (R
2
)
0.7532
The line of best fit representing the data is y = 1.9891x + 0.266. The slope (m) of the line of best fit is 1.9891, indicating the increase of 1.9891 runs batted per game for every home run per game. The y-intercept, representing the number of runs batted in per game if there are no home runs, is 0.266. There is a strong, positive linear correlation since the R value is approximately 0.8679, which is between 0.67 and 1. Comparing to the previous year, both the mean and median
for the runs batted in per game and the median for home runs per game increased. However, the mean for the home runs per game dropped slightly. The correlation coefficient dropped.
y = 1.9891x + 0.266
R² = 0.7532
0
0.1
0.2
0.3
0.4
0.5
0.6
0.7
0.8
0.9
1
0
0.05
0.1
0.15
0.2
0.25
0.3
0.35
RBI HR
HR v.s RBI for 70s
Your preview ends here
Eager to read complete document? Join bartleby learn and gain access to the full version
- Access to all documents
- Unlimited textbook solutions
- 24/7 expert homework help
Scatterplot for the 80s
Single Variable statistic
Home Runs per game (HR/G)
Runs Batted In per game (RBI/G)
Mean
0.122841
0.505749
Median
0.121429
0.510204
Correlation Coefficient (R)
0.8512
Coefficient of Determination (R
2
)
0.7246
The line of best fit representing the data is y = 1.7574x + 0.2899. The slope (m) of the line of best fit is 1.7574, indicating the increase of 1.7574 runs batted per game for every home run per game. The y-intercept, representing the number of runs batted in per game if there are no home runs, is 0.2899. There is a strong, positive linear correlation since the R value is approximately 0.8512, which is between 0.67 and 1. Comparing to the previous year, the mean and median for both home runs per game and runs batted in per game increased. However, the correlation coefficient is slightly lower, indicating a weaker linear correlation.
y = 1.7574x + 0.2899
R² = 0.7246
0
0.1
0.2
0.3
0.4
0.5
0.6
0.7
0.8
0.9
1
0
0.05
0.1
0.15
0.2
0.25
0.3
0.35
RBI
HR
HR v.s RBI for 80s
Scatterplot for the 90s
Single Variable statistic
Home Runs per game (HR/G)
Runs Batted In per game (RBI/G)
Mean
0.125018
0.525348
Median
0.118609
0.519157
Correlation Coefficient (R)
0.8745
Coefficient of Determination (R
2
)
0.7648
The line of best fit representing the data is y = 1.8322x + 0.2963. The slope (m) of the line of best fit is 1.8322, indicating the increase of 1.8322 runs batted per game for every home run per game. The y-intercept, representing the number of runs batted in per game if there are no home runs, is 0.2963. There is a strong, positive linear correlation since the R value is approximately 0.8745, which is between 0.67 and 1. Comparing to the previous year, the mean for both home runs per game and runs batted in per game increased, as well as the median for runs batted in per game. However, the median for home runs per game is lower. The correlation coefficient increased from 0.85 to 0.87, showing a strengthening linear correlation.
y = 1.8322x + 0.2963
R² = 0.7648
0
0.2
0.4
0.6
0.8
1
1.2
0
0.05
0.1
0.15
0.2
0.25
0.3
0.35
0.4
RBI
HR
HR v.s RBI in 90s
Scatterplot for the 2000s
Single Variable statistic
Home Runs per game (HR/G)
Runs Batted In per game (RBI/G)
Mean
0.135794
0.541216
Median
0.131142
0.536839
Correlation Coefficient (R)
0.8233
Coefficient of Determination (R
2
)
0.6779
The line of best fit representing the data is y = 1.7482x + 0.3038. The slope (m) of the line of best fit is 1.7482, indicating the increase of 1.7482 runs batted per game for every home run per game. The y-intercept, representing the number of runs batted in per game if there are no home runs, is 0.3038. There is a strong, positive linear correlation since the R value is approximately 0.8233, which is between 0.67 and 1. Comparing to the previous decade, the mean and median for both home runs per game and runs batted in per game has increased. However, the correlation
coefficient dropped from 0.87 to 0.82, showing a slightly weaker linear correlation.
y = 1.7482x + 0.3038
R² = 0.6779
0
0.1
0.2
0.3
0.4
0.5
0.6
0.7
0.8
0.9
1
0
0.05
0.1
0.15
0.2
0.25
0.3
0.35
0.4
RBI
HR
HR v.s RBI in 2000s
Your preview ends here
Eager to read complete document? Join bartleby learn and gain access to the full version
- Access to all documents
- Unlimited textbook solutions
- 24/7 expert homework help
Scatterplot for the 2010s
Single Variable statistic
Home Runs per game (HR/G)
Runs Batted In per game (RBI/G)
Mean
0.141001
0.49823
Median
0.140127
0.49635
Correlation Coefficient (R)
0.7859
Coefficient of Determination (R
2
)
0.6173
The line of best fit representing the data is y = 1.5754x + 0.2761. The slope (m) of the line of best fit is 1.5754, indicating the increase of 1.5754 runs batted per game for every home run per game. The y-intercept, representing the number of runs batted in per game if there are no home runs, is 0.2761. There is a strong, positive linear correlation since the R value is approximately 0.7859, which is between 0.67 and 1. Comparing to the previous decade, both mean and median for the home runs per game has increased. In contrast, both mean and median for the runs batted in per game has decreased. The correlation coefficient has dropped considerably, from 0.82 to 0.78, indicating a weaker linear correlation. Analysis, Discovery, and justification of hypothesis by Decades
I chose the decades as one of my subcategories since I would like to analyze the linear correlation in different decade. By comparing their correlation coefficient, mean, and median, I’m able to identify trends, similarities and differences, hidden variables, and make predictions. Furthermore, this would prove whether my hypothesis held true for every decade or if there were
any exceptions.
y = 1.5754x + 0.2761
R² = 0.6173
0
0.1
0.2
0.3
0.4
0.5
0.6
0.7
0.8
0.9
1
0
0.05
0.1
0.15
0.2
0.25
0.3
0.35
0.4
RBI
HR
HR v.s RBI in 2010s
After finding the correlation coefficient for each decade from 1920s to 2010s, it has proven that my hypothesis held truth. The correlation coefficient suggested that there’s a strong, positive correlation between home run per game and runs batted in per game. As the home run per game increased, the runs batted in per game increased. In fact, the lowest correlation coefficient,0.7174 during 1920s, would still consider as high R value. The range of R value is 0.1586, indicating that the R values are low variability in a distribution, less spread out and more consistent. The mean and median for the runs batted in per game fluctuated throughout the decades and does not seem to have any pattern nor trends. During the 80s, the mean and median value for home run per game increased significantly and suddenly, indicating that there might be a hidden variable. In addition, from the 40s to 50s, the home runs per game increased considerably, showing that there may be a hidden variable as well. From 50s to 60s, the correlation coefficient rose dramatically, which implies the
exist of hidden variable. The hidden variables will be discussed later. Overall, my hypothesis is supported by the data. The runs batted in per game will increase as home runs increase, and there’s a strong positive correlation between home run per game and runs batted In per game. However, this does not prove that there’s a cause-and-effect relationships. Therefore, further analysis and consideration of other potential factors may be necessary to fully understand the relationship between these two variables.
Positions (First Base, Outfielders, Shortstops)
Scatterplot
for the
first base
y = 1.4358x + 0.3798
R² = 0.5076
0
0.2
0.4
0.6
0.8
1
1.2
1.4
0
0.05
0.1
0.15
0.2
0.25
0.3
0.35
0.4
RBI
HR
HR v.s RBI for first base
Single Variable statistic
Home Runs per game (HR/G)
Runs Batted In per game (RBI/G)
Mean
0.139583
0.58019
Median
0.138158
0.569536
Correlation Coefficient (R)
0.7125
Coefficient of Determination (R
2
)
0.5076
The line of best fit representing the data is y = 1.4358x + 0.3798. The slope (m) of the line of best fit is 1.4358, indicating the increase of 1.4358 runs batted per game for every home run per game. The y-intercept, representing the number of runs batted in per game if there are no home runs, is 0.3798. There is a strong, positive linear correlation since the R value is approximately 0.7125, which is between 0.67 and 1.
Scatterplot
for the
outfielder
y = 1.5758x + 0.331
R² = 0.5601
0
0.2
0.4
0.6
0.8
1
1.2
0
0.05
0.1
0.15
0.2
0.25
0.3
0.35
0.4
0.45
RBI
HR
HR v.s RBI for outfielder
Your preview ends here
Eager to read complete document? Join bartleby learn and gain access to the full version
- Access to all documents
- Unlimited textbook solutions
- 24/7 expert homework help
Single Variable statistic
Home Runs per game (HR/G)
Runs Batted In per game (RBI/G)
Mean
0.116488
0.514606
Median
0.108108
0.510204
Correlation Coefficient (R)
0.7484
Coefficient of Determination (R
2
)
0.5601
The line of best fit representing the data is y = 1.5758x + 0.331. The slope (m) of the line of best fit is 1.5758, indicating the increase of 1.5758 runs batted per game for every home run per game. The y-intercept, representing the number of runs batted in per game if there are no home runs, is 0.331. There is a strong, positive linear correlation since the R value is approximately 0.7484, which is between 0.67 and 1.
Scatterplot for the shortstops
Single Variable statistic
Home Runs per game (HR/G)
Runs Batted In per game (RBI/G)
Mean
0.060905
0.401126
Median
0.043478
0.38586
Correlation Coefficient (R)
0.6617
Coefficient of Determination (R
2
)
0.4378
The line of best fit representing the data is y = 1.5529x + 0.3065. The slope (m) of the line of best fit is 1.5529, indicating the increase of 1.5529 runs batted per game for every home run per game. The y-intercept, representing the number of runs batted in per game if there are no home runs, is 0.3065. There is a moderate, positive linear correlation since the R value is approximately 0.6617, which is between 0.33 and 0.67.
y = 1.5529x + 0.3065
R² = 0.4378
0
0.1
0.2
0.3
0.4
0.5
0.6
0.7
0.8
0.9
0
0.05
0.1
0.15
0.2
0.25
0.3
0.35
RBI
HR
HR v.s RBI for shortstops
Analysis, Discovery, and justification of hypothesis by positions
I chose the positions as one of my subcategories since I would like to analyze the linear correlation for each position. By comparing their correlation coefficient, mean, and median, I’m able to identify trends, similarities and differences, hidden variables, and make predictions. Furthermore, this would prove whether my hypothesis held true for different positions or if there were any exceptions.
After finding the correlation coefficient for three positions I chose, it has again proven that my hypothesis held truth. The correlation coefficient for first base and outfielder suggested that there’s a strong, positive correlation between home run per game and runs batted in per game. As the home run per game increased, the runs batted in per game increased. The correlation coefficient was slightly below 0.67, therefore considered as a moderated – strong correlation between home run per game and runs batted in per game.
Moreover, among all three positions, the outfielder has the largest correlation coefficient, indicating that outfielder has the strongest correlation between home runs per game and runs batted in per game. T
his implies that outfielders are particularly effective and consistent at driving in runs when they hit home runs. In contrast, shortstops has the small correlation coefficient, indicating that shortstops has the relatively weaker correlation between home runs per game and runs batted in per game
Overall, my hypothesis is supported by the data. The runs batted in per game will increase as home runs increase, and there’s a strong positive correlation between home run per game and runs batted In per game. This applies to all three positions.
Your preview ends here
Eager to read complete document? Join bartleby learn and gain access to the full version
- Access to all documents
- Unlimited textbook solutions
- 24/7 expert homework help
Hits ( 1
st
quartile and 3
rd
quartile)
Scatterplot for 1
st
quartile of Hits
Single Variable statistic
Home Runs per game (HR/G)
Runs Batted In per game (RBI/G)
Mean
0.101875
0.428242
Median
0.096887
0.423726
Correlation Coefficient (R)
0.8506
Coefficient of Determination (R
2
)
0.7236
The line of best fit representing the data is y = 1.5777x + 0.2675. The slope (m) of the line of best fit is 1.5777, indicating the increase of 1.5777 runs batted per game for every home run per game. The y-intercept, representing the number of runs batted in per game if there are no home runs, is 0.2675. There is a strong, positive linear correlation since the R value is approximately 0.8506, which is between 0.67 and 1.
y = 1.5777x + 0.2675
R² = 0.7236
0
0.1
0.2
0.3
0.4
0.5
0.6
0.7
0.8
0.9
0
0.05
0.1
0.15
0.2
0.25
0.3
0.35
RBI/G
HR/G
HR/G v.s RBI/G in the 1st quartile of hits
Scatterplot for above 3
rd
quartile of Hits
Single Variable statistic
Home Runs per game (HR/G)
Runs Batted In per game (RBI/G)
Mean
0.111496
0.573649
Median
0.090323
0.56377
Correlation Coefficient (R)
0.7418
Coefficient of Determination (R
2
)
0.5502
The line of best fit representing the data is y = 1.616x + 0.3939. The slope (m) of the line of best fit is 1.616, indicating the increase of 1.616 runs batted per game for every home run per game. The y-intercept, representing the number of runs batted in per game if there are no home runs, is 0.3939. There is a strong, positive linear correlation since the R value is approximately 0.7418, which is between 0.67 and 1.
y = 1.616x + 0.3939
R² = 0.5502
0
0.2
0.4
0.6
0.8
1
1.2
1.4
0
0.05
0.1
0.15
0.2
0.25
0.3
0.35
0.4
0.45
RBI/G
HR/G
HR/G v.s RBI/G above 3rd Quartile of Hits
Analysis, Discovery, and justification of hypothesis by Hits
I chose the hits as one of my subcategories since I would like to analyze the linear correlation for groups of players with different hits per game. A hit
occurs when a batter strikes the baseball into fair territory and reaches base without doing so via an error or a fielder's choice
.
In baseball statistic, hits are often categorized as player's offensive productivity and would possibly be related to runs batted in and the number
of home run per game. By comparing their correlation coefficient, mean, and median, I’m able to identify trends, similarities and differences, and make predictions. Furthermore, this would prove whether my hypothesis held true for different positions or if there were any exceptions.
After finding the correlation coefficient for the 1
st
quartile of hits and 3
rd
quartile of hits, it
has proven that my hypothesis held truth. The correlation coefficient for both groups suggested that there’s a strong, positive correlation between home run per game and runs batted in per game. As the home run per game increased, the runs batted in per game increased. Both 1
st
quartile of hits and 3
rd
quartile of hits has high correlation coefficient. Surprisingly, the 3
rd
quartile of hits has relatively lower R value compared to the 1
st
quartile. The mean and median for the runs batted in per game are way higher for the 3
rd
quartile, proving that the hits per game (offensive productivity) may have impact on the runs batted in per game.
Overall, my hypothesis is supported by the data. The runs batted in per game will increase as home runs increase, and there’s a strong positive correlation between home run per game and runs batted in per game. This applies to the different quartiles of hits.
Scatterplot for the 1
st
quartile of walks (BB)
Single Variable statistic
Home Runs per game Runs Batted In per game y = 1.7175x + 0.3101
R² = 0.5491
0
0.2
0.4
0.6
0.8
1
1.2
0
0.05
0.1
0.15
0.2
0.25
0.3
0.35
RBI/G
HR/G
HR/G v.s RBI/G in the 1st Quartile of walks
Your preview ends here
Eager to read complete document? Join bartleby learn and gain access to the full version
- Access to all documents
- Unlimited textbook solutions
- 24/7 expert homework help
(HR/G)
(RBI/G)
Mean
0.084676
0.455575
Median
0.068442
0.447294
Correlation Coefficient (R)
0.7410
Coefficient of Determination (R
2
)
0.5491
The line of best fit representing the data is y = 1.7175x + 0.3101. The slope (m) of the line of best fit is 1.7175, indicating the increase of 1.7175 runs batted per game for every home run per game. The y-intercept, representing the number of runs batted in per game if there are no home runs, is 03101. There is a strong, positive linear correlation since the R value is approximately 0.7410, which is between 0.67 and 1.
Scatterplot for above 3
rd
quartile of walks (BB)
Single Variable statistic
Home Runs per game (HR/G)
Runs Batted In per game (RBI/G)
Mean
0.154434
0.585833
Median
0.151079
0.590604
Correlation Coefficient (R)
0.7403
Coefficient of Determination (R
2
)
0.548
The line of best fit representing the data is y = 1.4651x + 0.3596. The slope (m) of the line of best fit is 1.4651, indicating the increase of 1.4651 runs batted per game for every home run per game. The y-intercept, representing the number of runs batted in per game if there are no home runs, is 0.3596. There is a strong, positive linear correlation since the R value is approximately 0.7403, which is between 0.67 and 1.
y = 1.4651x + 0.3596
R² = 0.548
0
0.2
0.4
0.6
0.8
1
1.2
1.4
0
0.05
0.1
0.15
0.2
0.25
0.3
0.35
0.4
0.45
RBI/G
HR/G
HR/G v.s RBI/G above 3rd Quartile of walks
Your preview ends here
Eager to read complete document? Join bartleby learn and gain access to the full version
- Access to all documents
- Unlimited textbook solutions
- 24/7 expert homework help
Analysis, Discovery, and justification of hypothesis by walk
I chose the walks as one of my subcategories since I would like to analyze the linear correlation for groups of players with different numbers of walks.
A walk (or base on balls)
occurs when a pitcher throws four pitches out of the strike zone, none of which are swung at by the hitter
. After refrain
ing from swinging at four pitches out of the zone, the batter is awarded first base.
It appears that the number of walks could have direct impact on the runs batted in since players also receive an RBI for a bases-loaded walk or hit by pitch. By comparing
their correlation coefficient, mean, and median, I’m able to identify trends, similarities and differences, and make predictions. Furthermore, this would prove whether my hypothesis held true for different numbers of walks or if there were any exceptions.
After finding the correlation coefficient for the 1
st
quartile of hits and 3
rd
quartile of walks, both values were within 0.69 ~ 1, with the 1
st
quartile slightly higher than the 3
rd
. the values suggest that there’s a strong, positive correlation between home run per game and runs batted in per game. As the home run per game increased, the runs batted in per game increased. The mean and median of the runs in batted per game for the 3
rd
quartile of walks is significantly higher than the 1
st
quartile, suggesting that number of walks could have direct impact on the runs batted of a player and further affect the correlation between home runs per game and runs in batted per game slightly. I also realized that the mean and median value of the home run for the 3
rd
quartile of walks is considerably higher than the 1
st
quartile of walks. This indicates that the number of walks may have impact on the home run as well, which I was not expecting. Overall, the number of walks of an individual influence player’s performance, whether it’s the stats for home runs per game or runs in batted per game. However, both groups shows that there’s a strong, positive correlation between home runs per game and runs batted in per game, despite the difference between number of walks. This has proven that my hypothesis is correct. Critical analysis
Your preview ends here
Eager to read complete document? Join bartleby learn and gain access to the full version
- Access to all documents
- Unlimited textbook solutions
- 24/7 expert homework help
Outliers
An outlier is
an observation that lies an abnormal distance from other values in a random sample from a population
. Outliers are defined as any point that is 1.5 interquartile ranges (IQR) below the quadrant 1, and any points above quadrant 3, of the x and y values.
Outlier may possibly influence correlation coefficient. Therefore, it is vital to remove outliers in each decade subcategory and compare the new correlation coefficient of all data to the former one which outlier were presented. In addition, outliers
may allow me to better determine the hidden variables later. 20s
Q1
Q3
IQR
1.5 IQR
Q1-1.5IQR
Q3+1.5 IQR
Range for Outliers
Home Runs per game (HR/G)
0.01714184
0.0630478
7
0
.04590603
0
.0688590
45
-0.05171725
0.1319069
15
X
≤-
0.05171725
X
≥
0.13190691
5
Runs batted in per game (RBI/G)
0.40641858
0.6392197
7
0
.23280119
0
.3492017
85
0
.05721695
0.9884215
55
y
≤
0
.05721695
y
≥
0.98842155
5
Outliers for the 20s are the points where the x value is greater than or equal to 0.131906915 or less than or equal to -
0.05171725 and where the y value is greater than or equal to 0.988421555 or less than or equal to 0
.05721695. Points that have an outlier value:
(0.148148
, 0.651852), (0.165414
, 0.766917), (0.301471
, 0.838235), (0.197279, 0.619048), (0.269737, 0.861842), (0.133333
, 0.806667), (0.229008
, 0.748092), (0.205479, 0.883562), (0.303226, 1.129032
), (0.397351, 1.086093
).
Outliers: (0.303226, 1.129032
), (0.397351, 1.086093
).
30s
Your preview ends here
Eager to read complete document? Join bartleby learn and gain access to the full version
- Access to all documents
- Unlimited textbook solutions
- 24/7 expert homework help
Q1
Q3
IQR
1.5 IQR
Q1-1.5IQR
Q3+1.
5 IQR
Range for Outliers
Home Runs per game (HR/G)
0.027777
78
0.1005244
8
0.0727467
0.10912
005
-
0.08134227
0.2096
4453
x
≤
-0.08134227
x
≥
0.20964453
Runs batted in per game (RBI/G)
0.382027
83
0.640271
0.25824317
0.38736
4755
-
0.05336925
0.8985
1417
y
≤ -0.05336925
y
≥
0.89851417
Outliers for the 30s are the points where the x value is greater than or equal to 0.20964453 or less than or equal to -0.08134227
and where the y value is greater than or equal to 0.89851417 or less than or equal to -0.05336925
. Points that have an outlier value:
(
0.210526
, 0.914474), (0.322148
, 1.09396), (0.248175
, 0.751825), (0.198718, 0.987179
), (0.304636
, 1.10596), (0.24
, 0.846667), (0.25974, 1.188312
), (0.235669
, 1.012739
)
Outliers: (
0.210526
, 0.914474), (0.322148
, 1.09396), (0.304636
, 1.10596), (0.25974, 1.188312
), 0.235669
, 1.012739
)
40s
Q1
Q3
IQR
1.5 IQR
Q1-1.5IQR
Q3+1.
5 IQR
Range for Outliers
Home Runs per game (HR/G)
0.02409812
0.09878049
0.07463
237
0.112023
555
-
o.0879254
35
0.1734
1286
x
≤
-0.087925435
x
≥
0.17341286
Runs batted in
per game (RBI/G)
0.35125899
0.55555556
0.20429
657
0.306444
855
0.0448141
35
0.8620
00415
y
≤
0.044814135
y
≥
0.862000415
Your preview ends here
Eager to read complete document? Join bartleby learn and gain access to the full version
- Access to all documents
- Unlimited textbook solutions
- 24/7 expert homework help
Outliers for the 40s are the points where the x value is greater than or equal to 0.17341286
or less than or equal to -0.087925435 and where the y value is greater than or equal to 0.862000415 or less than or equal to 0.044814135
. Points that have an outlier value:
(
0.188312
, 0.831169), (0.219355
, 0.76129), (0.219858
, 0.609929), (0.210145
, 0.615942), (0.232258
, 0.690323), (0.331169
, 0.896104
), (0.175676
, 0.506757), (0.191489
, 0.602837), (0.335526
, 0.835526), (0.205128
, 0.730769)
Outlier: (0.331169
, 0.896104
)
50s
Q1
Q3
IQR
1.5 IQR
Q1-
1.5IQR
Q3+1.
5 IQR
Range for Outliers
Home Runs per game (HR/G)
0.06
0.16535433
0.1053543
3
0.158031
495
-
o.o98031
495
0.3233
85825
x
≤
-0.098031495
x
≥
0.323385825
Runs batted in
per game (RBI/G)
0.40140
845
0.65384615
0.2524377
0.378656
55
0.022751
9
0.9062
8385
y
≤
0.0227519
y
≥
0.90628385
Outliers for the 50s are the points where the x value is greater than or equal to 0.323385825 or less than or equal to -0.098031495 and where the y value is greater than or
equal to 0.90628385 or less than or equal to 0.0227519
. Points that have an outlier value: no points
Outlier: no outliers
Your preview ends here
Eager to read complete document? Join bartleby learn and gain access to the full version
- Access to all documents
- Unlimited textbook solutions
- 24/7 expert homework help
60s
Q1
Q3
IQR
1.5 IQR
Q1-1.5IQR
Q3+1.5 IQR
Range for Outliers
Home Runs per game (HR/G)
0.05140363
0.1562454
8
0.10484
185
0.157262
775
-
0.1058591
45
0.31350
8255
x
≤
-0.105859145
x
≥
0.313508255
Runs batted in per game (RBI/G)
0.32751992
0.5454143
8
0.21789
446
0.326841
69
0.0006782
8
0.87225
607
y
≤
0.00067828
y
≥
0.87225607
Outliers for the 60s are the points where the x value is greater than or equal to
0.313508255 or less than or equal to -0.105859145 and where the y value is greater than or
equal to 0.87225607or less than or equal to 0.00067828
. Points that have an outlier value: (
0.316901, 0.676056)
Outliers: no outliers.
70s
Q1
Q3
IQR
1.5 IQR
Q1-1.5IQR
Q3+1.5 IQR
Range for Outliers
Home Runs per game (HR/G)
0.0511416
8
0.1502451
0.09910
342
0.148655
13
-
0.0975134
5
0.298900
23
x
≤
-0.09751345
x
≥
0.29890023
Runs batted in per game (RBI/G)
0.3525093
0.59046864
0.23795
934
0.356939
01
-
0.0044297
1
0.947407
65
y
≤
-0.00442971
y
≥
0.94740765
Outliers for the 70s are the points where the x value is greater than or equal to 0.29890023
or less than or equal to -0.09751345 and where the y value is greater than or equal to
0.94740765or less than or equal to -0.00442971
. Points that have an outlier value:
Your preview ends here
Eager to read complete document? Join bartleby learn and gain access to the full version
- Access to all documents
- Unlimited textbook solutions
- 24/7 expert homework help
(
0.329114
, 0.943038)
Outlier: no outliers
80s
Q1
Q3
IQR
1.5 IQR
Q1-1.5IQR
Q3+1.5 IQR
Range for Outliers
Home Runs per game (HR/G)
0.0621118
0.17763158
0.1155
1978
0.17327
967
-
o.1111678
7
0.3509112
5
x
≤
-0.11116787
x
≥
0.35091125
Runs batted in per game (RBI/G)
0.4014598
5
0.61589404
0.2144
3419
0.32165
1285
0.0798085
65
0.9375453
25
y
≤
0.079808565
y
≥
0.937545325
Outliers for the 80s are the points where the x value is greater than or equal to 0.35091125
or less than or equal to - 0.11116787 and where the y value is greater than or equal to
0.937545325 or less than or equal to 0.079808565
. Points that have an outlier value: no points
Outliers: no outliers
90s
Q1
Q3
IQR
1.5 IQR
Q1-1.5IQR
Q3+1.5 IQR
Range for Outliers
Home Runs per game (HR/G)
0.0625982
7
0.176184
11
0.11358
584
0.1703
7876
-
0.10778049
0.3465628
7
x
≤
-0.10778049
x
≥
0.34656287
Runs batted in per game (RBI/G)
0.3926644
5
0.640202
28
0.24753
783
0.3713
06745
0.02135770
5
1.0115090
25
y
≤
0.021357705
y
≥
1.011509025
Your preview ends here
Eager to read complete document? Join bartleby learn and gain access to the full version
- Access to all documents
- Unlimited textbook solutions
- 24/7 expert homework help
Outliers for the 90s are the points where the x value is greater than or equal to 0.34656287
or less than or equal to -0.10778049 and where the y value is greater than or equal to
1.011509025 or less than or equal to 0.021357705
. Points that have an outlier value: (
0.356688
, 0.936306), (0.371795, 0.788462)
Outliers: no outliers
2000s
Q1
Q3
IQR
1.5 IQR
Q1-1.5IQR
Q3+1.5 IQR
Range for Outliers
Home Runs per game (HR/G)
0.08426704
0.17987179
0.09
5604
75
0.14340
7125
-
0.0591400
85
0.3232789
15
x
≤
-0.059140085
x
≥
0.323278915
Runs batted in per game (RBI/G
)
0.43312212
0.64675245
0.21
3630
33
0.32044
5495
0.1126766
25
0.9671979
45
y
≤
0.112676625
y
≥
0.967197945
Outliers for the 2000s are the points where the x value is greater than or equal to
0.323278915 or less than or equal to -0.059140085 and where the y value is greater than or
equal to 0.967197945 or less than or equal to 0.112676625
. Points that have an outlier value: (
0.346154
, 0.692308), (0.326389
, 0.944444)
Outlier: no outliers
Your preview ends here
Eager to read complete document? Join bartleby learn and gain access to the full version
- Access to all documents
- Unlimited textbook solutions
- 24/7 expert homework help
2010s
Q1
Q3
IQR
1.5 IQR
Q1-
1.5IQR
Q3+1.5 IQR
Range for Outliers
Home Runs per game (HR/G)
0.09655
172
0.1838235
3
0.08830
633
0.1324594
95
-
0.03590
7775
0.316283
025
x
≤
-0.035907775
x
≥
0.316283025
Runs batted in per game (RBI/G
)
0.4
0.5960264
9
0.19602
649
0.2940397
35
0.10596
0265
0.890066
225
y
≤
0.105960265
y
≥
0.890066225
Outliers for the 2010s are the points where the x value is greater than or equal to
0.316283025 or less than or equal to -0.035907775 and where the y value is greater than or
equal to 0.890066225 or less than or equal to 0.105960265
. Points that have an outlier value: (0.371069, 0.830189)
Outliers: no outliers
Your preview ends here
Eager to read complete document? Join bartleby learn and gain access to the full version
- Access to all documents
- Unlimited textbook solutions
- 24/7 expert homework help
Outlier analysis
After removing all the outliers in each decade, the correlation coefficient is approximately 0.7543, a decrease from 0.7618 when outliers exist. This seems surprising to me as I believed that the removal of outliers will enhance data’s overall correlation. The new correlation coefficient still supports my hypothesis that there’s a linear, strong, positive correlation between home run per game and runs in batted per game. I did a bit of research on what I discovered. “In most practical circumstances an outlier decreases the value of a correlation coefficient and weakens the regression relationship, but it’s also possible that in some circumstances an outlier may increase a correlation value and improve regression. This is an example of influential
outlier. Influential outliers are points in a data set that influence the regression equation and improve correlation.” In another word, the outliers helped to maintain and enhance the linear form of the data points and their removal negatively impacted the correlation present. As a result, even though the correlation coefficient only decreased by a little (0.075), I decided stick with my original graphs with the existence of outliers to do further analysis later, since it has slightly stronger correlation between home run per game and runs batted in per game. When determine the outliers, I also realized that the outliers in each decade decrease as the time pass on. This explained the reason why the coefficient correlation improved decades after decades.
y = 1.5792x + 0.3307
R² = 0.5689
0
0.2
0.4
0.6
0.8
1
1.2
0
0.05
0.1
0.15
0.2
0.25
0.3
0.35
0.4
HR/G
RBI/G
HR/G v.s RBI/G (outliers removed)
Your preview ends here
Eager to read complete document? Join bartleby learn and gain access to the full version
- Access to all documents
- Unlimited textbook solutions
- 24/7 expert homework help
Hidden Variables:
Dead Ball era
The
Dead ball Era
was a period in the early 20th Century characterized by low scoring and an emphasis on
pitching
and
defense
. L
eague
batting averages
dropped as low as .239 in 1908, producing the lowest league
run
average in history, with teams averaging only 3.4 runs per game.
The possible causes were that teams played in spacious ballparks that limited hitting for power. As a further hindrance to scoring, the
ball
used then, compared to modern baseballs, was "dead" both by design and from overuse (
ball scuffing).
For pitchers, it was the era of the "spit ball" completely legal at the time. Many pitchers relied on the spit ball and other trickery to keep batters on their toes. Some of the most skilled pitchers of all time developed in baseball's Dead ball Era, Batters used heavy bats, choked up on the handle and didn't attack the pitch aggressively.
Also, the foul strike rule was a major rule change that, in just a few years, sent baseball from a high-scoring game to a game where scoring any runs was a struggle. Under the foul strike rule, a batter who
fouls off
is charged with a
strike
unless he already has two strikes against him. Some players and fans complained about the low-scoring games, and league officials sought to remedy the situation.
The dead-ball era ended suddenly. By 1921, offenses were scoring 40% more runs and hitting four times as many home runs as they had in 1918. Solution were the cleaner baseballs, change in baseball construction (
Ben Shibe
invented the
cork
-centered ball, The change in the ball dramatically
increased the batting average), banned
of spitball, ballpark(
changes in the dimensions of the ballparks.), Baby Ruth( theory that the prolific success of Babe Ruth at hitting home runs led players around the league to forsake their old methods of hitting (described above) and adopt a "free-swinging" style designed to hit the ball hard and with an uppercut stroke, with the intention of hitting more home runs). Due to the dead ball era, there are less home run per game and runs batted in per game. As a result, dead ball era is a hidden variable and causes the R
value to decrease. The correlation coefficient of the 20s were significantly lower than the 30s, which explain the impact of dead ball era. The steroid era
"The steroids era" refers to a period of time in Major League Baseball when a number of players were believed to have used performance-enhancing drugs, resulting in increased offensive output
throughout the game. It is generally considered to have run from the late '80s through the late 2000s. Though steroids have been banned in MLB since 1991, the league did not implement leaguewide PED testing until 2003. Anabolic steroids help build muscle tissue and increase body
mass by acting like the body's natural male hormone, testosterone.
During the 1990s, Major League Baseball experienced an increase in offensive output that resulted in some unprecedented
home run totals for the power hitters of the decade. While just three players reached the 50-home
run mark in any season between 1961 and 1994, many sluggers would start to surpass that number in the mid-90s.
The average number of players who hit more than 40 HR in a single season significantly increased during the steroid era
. Former MLB player
Jose Canseco has estimated that around 85 percent
of MLB players use steroids. Ken Carminati, another former player, estimated approximately 50 percent. This could possibly explain the dramatic increase of mean and median for home run per game from 70s to 80s. The steroids era causes the R value to
Your preview ends here
Eager to read complete document? Join bartleby learn and gain access to the full version
- Access to all documents
- Unlimited textbook solutions
- 24/7 expert homework help
increase as the home run per game increased. As the PED testing were introduced worldwide in the 2000s, the R value decreased significantly. This may be a result of players being more aware of the use of steroids.
World War II
World War II
was a
world war
that lasted from 1939 to 1945. It involved the
vast majority of the world's countries
—including the United States. Baseball was far and away the favorite sport in the country in the 1940s. Fans watched as many players left to join the military in the conflict. in all, 500 Major League players and more than 2,000 minor league players left to join the military,
according to
the American Veterans Center. While the statistical numbers were down during these years with so many talented players gone, the game itself grew in popularity as the war went on. The significant reduction of players during World War II causes the lower of sample size, which leads to the occurrence of sampling bias. The mean and median for both home run per game and runs batted in per game were considerably lower, comparing to the post war statistic. This explained that during war, the level of game decreased, as well as the statistics.
As a result, World War II is a hidden variable potentially affecting the correlation between home run per game and runs batted in per game. Great depression
The Great Depression was period of worldwide economic depression
between 1929 and 1939
. The major effect of the Great Depression on baseball was a decrease in attendance at professional baseball games. Because of the Depression, people had less money available for leisure activities. Baseball games were a luxury that could no longer be afforded by the common American. Many teams, strong and weak ones alike, kept costs down by reducing the number of coaches, or by eliminating them and employing player-managers. Owners also reduce the number of rosters. Even the best players — Babe Ruth among them — took pay cuts. Connie Mack sold many of the stars from the pennant-winning Philadelphia Athletics teams of 1929, 1930 and 1931. Since less player was playing during great depression, the sampling size were reduced, leading to the occurrence of sampling bias.
Covid 19
The
COVID-19 pandemic
has caused disruption to major leage baseball. Leagues across the world experienced delayed starts, cancelled seasons, limited or no fan attendance, game postponements, and other restrictions. Players who had covid 19 may experience a decline in performance. In a study that included 71 hitters and 61 pitchers who were confirmed to have had Covid,
The Athletic
found that performance was well down from expectations in the first two weeks back off the injured list. In the sample population, hitters’ median OPS went down 63 points compared to pre-season projections; Pitchers’ median ERA went up 11 points compared to pre-season projections; 69 percent of the pitchers lost velocity compared to the 15 days before,
with a median velocity loss of 0.4 mph; 54 percent of the hitters lost exit velocity compared to the 15 days before, with a median exit velocity loss of 0.6 mph. It is clear that covid 19 has tremendously impacted player’s overall performance. As a result, I would consider covid 19 as a hidden varible contributing to the lowering of correlation coefficient.
Your preview ends here
Eager to read complete document? Join bartleby learn and gain access to the full version
- Access to all documents
- Unlimited textbook solutions
- 24/7 expert homework help
Conclusion
Based on the data, graphs, and analysis, I believe that my hypothesis held true. The data shows that there’s a strong, positive, linear correlation between home runs per game and runs batted in per game. All the subcategories reveal the same results: the runs batted in per game increases as the home run per game increased. This correlation corresponds and applies to every decades investigated, different positions chosen, different quartiles of hits, and different quartile of walks.
The result is very consistent without an exception. All the correlation coefficient value are within
0.67~1, suggest a strong positive correlation. Therefore, I can conclude that there’s a strong, positive, linear correlation between home runs per game and runs batted in per game, however, not necessarily a cause-and-effect relationship. I also create another data excluding the outliers data. The correlation coefficient is surprisingly lower, indicating that the removal of outliers has weaken the overall correlation of data. This is found to be an example of influential
outlier, which outliers are points in a data set that influence the regression equation and improve correlation. In addition, I include mean and median for every graph, allowing me to better identify the hidden variables, trends, and its effect on data’s correlation. I’ve also investigated five possible hidden variables that may have impact on the correlation coefficient. This is proven
by the data as well. For example, during the steroid era, the number of home runs increased dramatically. The dramatic increase in mean and median for home run per game from 70s to 80s explained this situation. The steroids era causes the R value to increase as the home run per game
increased. As the PED testing were introduced worldwide in the 2000s, the R value decreased significantly and may be a result of players being more aware of the use of steroids. Sampling technique
I collected the data from Major league baseball website for the regular season hitting statistic. Using systematic sampling, I randomly select two numbers from one to nine, to determine the ending of year for each decade. I selected every ending with three and seven. This way, I would have two random years that were systematically chosen for each decade, minimizing the possibility of sampling bias. Using the data collected for each decade, I created scatterplots that shows the correlation between home runs per game and runs batted in per game. I’ve chosen to use linear model since the data weren’t increasing exponentially, and this model worked out well
for my data. I graphed the scatterplot for all the subcategories discussed. For the graph that exclude outliers, I first separate all the data by decades. In each decades, I determined the first quartile, third quartile, and interquartile range. Then, I eliminated all the outliers within a decade by finding and excluding values that are 1.5 interquartile range above third quadrant and 1.5 interquartile range below first quadrant. I repeated this process for all the decades, from 1920s to 2010s. Lastly, I combine all the decades and create a scatterplot that reveals the new correlation coefficient with the absence of outliers.
Improvement
I believe that there is a lot of improvement that could be made to enhance the overall analysis for
this project. First, I could increase the sample size for decades. Instead of selecting two years for each decade, I should select four years for each decades in order to maximize the accuracy and better determine the trends. Also, instead of choosing three positions, I should have chosen all hitting positions in order to better analyze the correlation in each position and the relationships between each position. Also, when selecting the ending year for each decade, I could have use
Your preview ends here
Eager to read complete document? Join bartleby learn and gain access to the full version
- Access to all documents
- Unlimited textbook solutions
- 24/7 expert homework help
nine pokers, each containing different numbers. In this case, the sampling bias will be minimized
since it’s purely random, instead of personal preference. Prediction
To predict the future increases of runs batted in for every home run, I created a graph that shows the relationship between decade and the slopes of all the line of best fit for the decade subcategory. I put the slope as the dependent variable and decades as independent variable. Decades
20s
30s
40s
50s
60s
70s
80s
90s
2000s
2010s
Slope
2.0022
2.3454
1.6928
1.7267
1.7704
1.9891
1.757
4
1.8322
1.7482
1.5754
The equation of the line of best fit for this relation is y=-0.0043x+10.197. To make prediction for
specific decade, simply substitute the decade value into x, to solve for the slope, y.
E.g., during 1960s, the number of runs batted in will increase by 1.769 for every home run made.
Your preview ends here
Eager to read complete document? Join bartleby learn and gain access to the full version
- Access to all documents
- Unlimited textbook solutions
- 24/7 expert homework help
References
Wikimedia Foundation. (2022, December 6).
Dead-Ball Era
. Wikipedia. Retrieved December 19, 2022, from https://en.wikipedia.org/wiki/Dead-ball_era
Team, I. S. E. (2018, July 5).
What was baseball like during World War 2?: Historic girls baseball
. Imagine Sports. Retrieved December 19, 2022, from https://imaginesports.com/news/baseball-during-world-war-2
Corso, J. (2017, October 3).
Major League Baseball's popularity during WWII
. Bleacher Report. Retrieved December 19, 2022, from https://bleacherreport.com/articles/161265-major-
league-baseballs-popularity-during-wwii
Sarris, E. (n.d.).
How does Covid Impact MLB Players' Performance? what athletes, trainers and
the stats say
. The Athletic. Retrieved December 19, 2022, from https://theathletic.com/3488516/2022/08/26/mlb-players-covid-return-effects/
Ramy Elitzur Associate Professor. (2022, July 20).
Pandemic moneyball: How covid-19 has affected baseball odds
. The Conversation. Retrieved December 19, 2022, from https://theconversation.com/pandemic-moneyball-how-covid-19-has-affected-baseball-
odds-157203
How did the Great Depression affect baseball in the 1930s
. Cram. (n.d.). Retrieved December 19, 2022, from https://www.cram.com/essay/How-Did-The-Great-Depression-Affect-
Baseball/FCC6LWLQWV
Cautions about correlation and regression: STAT 800
. PennState: Statistics Online Courses. (n.d.). Retrieved December 19, 2022, from https://online.stat.psu.edu/stat800/lesson/cautions-about-correlation-and-regression
ESPN Internet Ventures. (n.d.).
The steroids era
. ESPN. Retrieved December 19, 2022, from https://www.espn.com/mlb/topics/_/page/the-steroids-era
Wikimedia Foundation. (2022, October 28).
Base on balls
. Wikipedia. Retrieved December 19, 2022, from https://en.wikipedia.org/wiki/Base_on_balls
Walk (BB): Glossary
. MLB.com. (n.d.). Retrieved December 19, 2022, from https://www.mlb.com/glossary/standard-stats/walk
The official site of Major League Baseball
. MLB.com. (n.d.). Retrieved December 19, 2022, from https://www.mlb.com/
Your preview ends here
Eager to read complete document? Join bartleby learn and gain access to the full version
- Access to all documents
- Unlimited textbook solutions
- 24/7 expert homework help
Your preview ends here
Eager to read complete document? Join bartleby learn and gain access to the full version
- Access to all documents
- Unlimited textbook solutions
- 24/7 expert homework help
Recommended textbooks for you
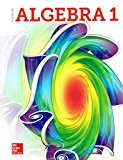
Glencoe Algebra 1, Student Edition, 9780079039897...
Algebra
ISBN:9780079039897
Author:Carter
Publisher:McGraw Hill
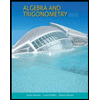
Algebra and Trigonometry (MindTap Course List)
Algebra
ISBN:9781305071742
Author:James Stewart, Lothar Redlin, Saleem Watson
Publisher:Cengage Learning
Algebra & Trigonometry with Analytic Geometry
Algebra
ISBN:9781133382119
Author:Swokowski
Publisher:Cengage
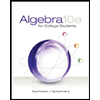
Algebra for College Students
Algebra
ISBN:9781285195780
Author:Jerome E. Kaufmann, Karen L. Schwitters
Publisher:Cengage Learning
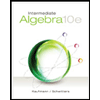
Intermediate Algebra
Algebra
ISBN:9781285195728
Author:Jerome E. Kaufmann, Karen L. Schwitters
Publisher:Cengage Learning
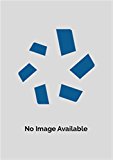
Big Ideas Math A Bridge To Success Algebra 1: Stu...
Algebra
ISBN:9781680331141
Author:HOUGHTON MIFFLIN HARCOURT
Publisher:Houghton Mifflin Harcourt
Recommended textbooks for you
- Glencoe Algebra 1, Student Edition, 9780079039897...AlgebraISBN:9780079039897Author:CarterPublisher:McGraw HillAlgebra and Trigonometry (MindTap Course List)AlgebraISBN:9781305071742Author:James Stewart, Lothar Redlin, Saleem WatsonPublisher:Cengage LearningAlgebra & Trigonometry with Analytic GeometryAlgebraISBN:9781133382119Author:SwokowskiPublisher:Cengage
- Algebra for College StudentsAlgebraISBN:9781285195780Author:Jerome E. Kaufmann, Karen L. SchwittersPublisher:Cengage LearningIntermediate AlgebraAlgebraISBN:9781285195728Author:Jerome E. Kaufmann, Karen L. SchwittersPublisher:Cengage LearningBig Ideas Math A Bridge To Success Algebra 1: Stu...AlgebraISBN:9781680331141Author:HOUGHTON MIFFLIN HARCOURTPublisher:Houghton Mifflin Harcourt
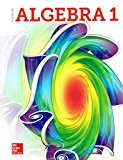
Glencoe Algebra 1, Student Edition, 9780079039897...
Algebra
ISBN:9780079039897
Author:Carter
Publisher:McGraw Hill
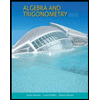
Algebra and Trigonometry (MindTap Course List)
Algebra
ISBN:9781305071742
Author:James Stewart, Lothar Redlin, Saleem Watson
Publisher:Cengage Learning
Algebra & Trigonometry with Analytic Geometry
Algebra
ISBN:9781133382119
Author:Swokowski
Publisher:Cengage
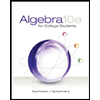
Algebra for College Students
Algebra
ISBN:9781285195780
Author:Jerome E. Kaufmann, Karen L. Schwitters
Publisher:Cengage Learning
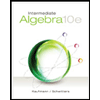
Intermediate Algebra
Algebra
ISBN:9781285195728
Author:Jerome E. Kaufmann, Karen L. Schwitters
Publisher:Cengage Learning
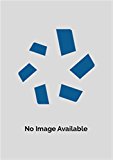
Big Ideas Math A Bridge To Success Algebra 1: Stu...
Algebra
ISBN:9781680331141
Author:HOUGHTON MIFFLIN HARCOURT
Publisher:Houghton Mifflin Harcourt