ECON 493 PS 6
pdf
keyboard_arrow_up
School
University of British Columbia *
*We aren’t endorsed by this school
Course
493
Subject
Statistics
Date
Feb 20, 2024
Type
Pages
7
Uploaded by pragnya030202
Answer 1
1.
The regression analysis for attendance yields a coefficient of 0.0278363, with a t-statistic
value of 4.01. The t-statistic exceeds the conventional significance cutoff of 1.96,
indicating significant statistical relevance. The magnitude of this effect is considerable,
translating to an increase of 2.78 percentage points in the standardized final exam score
for each additional class attended.
2.
The primary issue with the uncontrolled regression from part (1) when assessing the
causal impact of attendance on exam scores is the potential for omitted variable bias.
Factors like a student's GPA / personal ambition could also affect final exam outcomes. If
these variables are not accounted for, it could skew the observed effect of attendance on
exam scores due to selection bias. To mitigate this problem, it is necessary to adjust for
all known variables that are thought to influence exam performance.
3.
Adding termgpa to the regression:
a) Adding termgpa as a control variable partially addresses the issue of omitted
variable bias and advances the quest for establishing a causal relationship
between class attendance and exam performance. Despite a significant change
in the attendance coefficient upon controlling for termgpa, the consideration of
additional variables is imperative for a more accurate analysis.
b) The increased standard error in the attendance coefficient upon including
termgpa indicates that this control variable is more predictive of the propensity to
attend than of the final exam scores themselves. It is also critical to evaluate that
termgpa may be influenced by attendance, suggesting its potential
ineffectiveness as a control due to the possibility of introducing selection bias,
thereby complicating the causal interpretation of the attendance effect.
c) The stated attendance coefficient of -0.0351458 suggests that an increase in
attendance by one unit is associated with an approximate decrease of 3.5
percentage points in standardized final exam scores when termgpa is held
steady.
Adding priGPA to the regression:
a)
Factoring in priGPA as a control variable mitigates some of the omitted variable
bias, moving us toward isolating the causal impact of attending lectures. The
substantial shift in the attendance coefficient upon controlling for priGPA
indicates that other factors still need to be considered for a thorough analysis.
The observation that the standard error for attendance increased suggests that
priGPA more accurately forecasts attendance patterns than stndfnl.
b)
Probably not. While the standard error for the coefficient on attend increased,
unlike termgpa, priGPA is not a consequence of attendance, which qualifies it as
an effective control in this regression model.
c)
With an attendance coefficient of -0.0017479, the data suggest a minor decrease
of about 0.17 percentage points in standardized final exam scores per unit
increase in attendance, with priGPA held constant. Yet, the insignificance of this
relationship is highlighted by a low t-statistic of -0.23 and a high p-value of 0.815,
casting doubt on the existence of any substantive link between attendance and
exam performance.
Adding ACT to the regression:
a)
By incorporating the ACT score as a control variable, we address some aspects
of the omitted variable bias and edge closer to determining the causal effect of
attending lectures on exam scores. However, recognizing that other variables
also merit consideration is crucial for a robust model. The altered attendance
coefficient after adjusting for the ACT score signifies that there's more to the
equation.
b)
It's critical to consider that ACT scores may not be an effect of lecture
attendance, which contrasts with earlier assumptions about termgpa. Including
ACT as a control could introduce selection bias, thus obstructing a clear causal
inference from the attendance coefficient.
c)
With the attendance coefficient at 0.0375987, there's an indicated average
increase of 3.76 percentage points in final exam scores for each additional unit of
lecture attendance, keeping ACT scores constant. This relationship implies that
despite potential biases, there may be a positive association between lecture
attendance and exam performance.
4.
Upon reviewing the data in part (3), priGPA, or the cumulative GPA before the current
term, emerges as the preferred control variable for exploring the causal influence of
lecture attendance on exam scores. The selection of priGPA is strategic because it is not
influenced by current lecture attendance, unlike termgpa and ACT scores, which may
both be affected by the treatment variable, attendance. Using termgpa or ACT as
controls could potentially lead to selection bias, as they might be consequences of
attending lectures. By contrast, priGPA is established prior to and therefore independent
of the current term's lecture attendance, rendering it a stable and reliable control for
regression analysis aimed at identifying causal relationships. The attendance coefficient,
marked at -0.0017479 with a t-statistic of -0.23 and a p-value of 0.815, fails to meet the
criteria for statistical significance. The t-statistic's absolute value is below the critical
value of 1.96, and the p-value exceeds the threshold of 0.05, leading to the conclusion
that the attendance coefficient is statistically inconsequential. Consequently, the null
hypothesis, which states there is no difference from zero for the attendance coefficient,
cannot be rejected based on the t-statistic, p-value, and the 95% confidence interval
provided. Thus, it is not possible to assert that class attendance has a tangible impact on
the standardized final exam scores.
5.
Adding hwrte to the regression:
In this regression analysis, the completing homework variable is considered as a
potential factor that could unveil the causal relationship between lecture attendance and
student performance. The coefficient for completing homework stands at 0.0031609 with
a t-statistic of 1.17, which is below the significance threshold of 1.96, indicating its
statistical insignificance. Furthermore, the coefficient for attendance shows a reduction
from -0.0017479 to -0.0008707 upon including homework in the model. With a
corresponding t-statistic of -0.93 and a p-value of 0.353 for attendance, these values fall
short of the significance benchmarks. As such, both the t-statistic and the p-value do not
support rejecting the null hypothesis that the attendance coefficient differs from zero.
Therefore, it can be concluded that there is no statistically significant evidence to
suggest that lecture attendance influences standardized final exam scores, even after
accounting for the variable of completing homework.
6.
Adding skipped to the regression in (4):
The variable 'skipped' is excluded from the regression due to collinearity, which arises
when two or more predictor variables in the model are highly interrelated. This
interdependence complicates the model's ability to isolate the specific impact of each
predictor. The rationale for the removal of 'skipped' is that it is inherently related to
'attend'—not attending class inherently means the class was skipped. Therefore, 'attend'
and 'skipped' are so closely linked that they do not provide distinct information for the
purposes of regression analysis, thus justifying the omission of 'skipped' to avoid
redundancy and to clarify the model's predictive capability.
Answer 2
1.
The coefficients for 'black' and 'Hispanic' in the regression model quantify the average
height differences between black or Hispanic individuals and those who are
not—typically white or other races in the sample. In this analysis, the coefficient for
'black' is -0.20 , suggesting that black males are, on average, 0.20 inches shorter than
their non-black counterparts. Conversely, the coefficient of 0.04 for black females,
implying they are, on average, 0.04 inches taller than non-black females. A similar
approach is taken when interpreting the 'Hispanic' coefficient, which compares the
average height of Hispanic individuals to non-Hispanic ones within the specified gender
groups.
2.
To test if there's a statistically significant difference in the Hispanic variable's coefficient
between males and females, an interaction term between gender (coded as 0 for males
and 1 for females) and the Hispanic variable can be introduced in the regression model.
The null hypothesis (H0) posited would assert that the Hispanic coefficient is identical for
both genders. In contrast, the alternative hypothesis (H1) would contend that the
Hispanic coefficient varies between males and females. The regression model would
include these interaction terms to observe any significant differences in the coefficient,
allowing for a nuanced analysis of the variable's impact by gender.
Heighti = α + β1Blacki + β2Hispanici + γASVAB + β4Dummyi + β5Hispanici*Dummyi
Your preview ends here
Eager to read complete document? Join bartleby learn and gain access to the full version
- Access to all documents
- Unlimited textbook solutions
- 24/7 expert homework help
3.
Running separate regressions for males and females gives distinct coefficients for the
constant term, the 'Hispanic' variable, and 'cognitive ability'. From the male-specific
regression we have an intercept α(constant) of 68.24, a 'Hispanic' coefficient (β1) of
-2.20, and a 'cognitive ability' coefficient (γ) of 0.046. The female-specific regression
provides an intercept of 62.27, a 'Hispanic' coefficient of -1.76, and a 'cognitive ability'
coefficient of 0.038. The coefficient for 'white' (β2) is not provided for either regression,
which implies that it is either omitted or subsumed within the constant term as the
reference category against which the 'Hispanic' variable's effect is measured.
Do-file:
. use "/Users/pragnyasanghvi/Downloads/attend.dta"
. regress stndfnl attend, robust
Linear regression
Number of obs
=
674
F(1, 672)
=
16.08
Prob > F
=
0.0001
R-squared
=
0.0219
Root MSE
=
.98242
------------------------------------------------------------------------------
|
Robust
stndfnl | Coefficient std. err.
t
P>|t|
[95% conf. interval]
-------------+----------------------------------------------------------------
attend |
.0278363
.0069417
4.01
0.000
.0142063
.0414662
_cons |
-.702364
.1816686
-3.87
0.000
-1.05907
-.3456577
------------------------------------------------------------------------------
. regress stndfnl attend termgpa, robust
Linear regression
Number of obs
=
674
F(2, 671)
=
112.49
Prob > F
=
0.0000
R-squared
=
0.2968
Root MSE
=
.83362
------------------------------------------------------------------------------
|
Robust
stndfnl | Coefficient std. err.
t
P>|t|
[95% conf. interval]
-------------+----------------------------------------------------------------
attend | -.0351458
.0075528
-4.65
0.000
-.0499757
-.0203159
termgpa |
.8518788
.0593675
14.35
0.000
.7353104
.9684471
_cons | -1.273935
.1709605
-7.45
0.000
-1.609617
-.9382536
------------------------------------------------------------------------------
. regress stndfnl attend priGPA, robust
Linear regression
Number of obs
=
674
F(2, 671)
=
48.04
Prob > F
=
0.0000
R-squared
=
0.1377
Root MSE
=
.92311
------------------------------------------------------------------------------
|
Robust
stndfnl | Coefficient std. err.
t
P>|t|
[95% conf. interval]
-------------+----------------------------------------------------------------
attend | -.0017479
.0074481
-0.23
0.815
-.0163722
.0128764
priGPA |
.6843534
.0775414
8.83
0.000
.5321006
.8366063
_cons | -1.698644
.2101562
-8.08
0.000
-2.111287
-1.286001
------------------------------------------------------------------------------
. regress stndfnl attend ACT, robust
Linear regression
Number of obs
=
674
F(2, 671)
=
68.73
Prob > F
=
0.0000
R-squared
=
0.1702
Root MSE
=
.90557
------------------------------------------------------------------------------
|
Robust
stndfnl | Coefficient std. err.
t
P>|t|
[95% conf. interval]
-------------+----------------------------------------------------------------
attend |
.0375987
.0064706
5.81
0.000
.0248938
.0503037
ACT |
.1107491
.0101418
10.92
0.000
.0908356
.1306626
_cons | -3.448301
.3042148
-11.34
0.000
-4.045628
-2.850973
------------------------------------------------------------------------------
. regress stndfnl attend priGPA, robust
Linear regression
Number of obs
=
674
F(2, 671)
=
48.04
Prob > F
=
0.0000
R-squared
=
0.1377
Root MSE
=
.92311
------------------------------------------------------------------------------
|
Robust
stndfnl | Coefficient std. err.
t
P>|t|
[95% conf. interval]
-------------+----------------------------------------------------------------
attend | -.0017479
.0074481
-0.23
0.815
-.0163722
.0128764
priGPA |
.6843534
.0775414
8.83
0.000
.5321006
.8366063
_cons | -1.698644
.2101562
-8.08
0.000
-2.111287
-1.286001
------------------------------------------------------------------------------
. regress stndfnl attend priGPA hwrte, robust
Linear regression
Number of obs
=
674
F(3, 670)
=
33.20
Prob > F
=
0.0000
R-squared
=
0.1400
Root MSE
=
.92257
------------------------------------------------------------------------------
|
Robust
stndfnl | Coefficient std. err.
t
P>|t|
[95% conf. interval]
-------------+----------------------------------------------------------------
attend |
-.008707
.0093685
-0.93
0.353
-.0271021
.0096881
priGPA |
.6782941
.0781394
8.68
0.000
.5248666
.8317216
hwrte |
.0031609
.002702
1.17
0.242
-.0021446
.0084663
_cons | -1.777928
.2193038
-8.11
0.000
-2.208533
-1.347322
------------------------------------------------------------------------------
. regress stndfnl attend priGPA skipped, robust
note: skipped omitted because of collinearity.
Linear regression
Number of obs
=
674
F(2, 671)
=
48.04
Prob > F
=
0.0000
R-squared
=
0.1377
Root MSE
=
.92311
------------------------------------------------------------------------------
|
Robust
stndfnl | Coefficient std. err.
t
P>|t|
[95% conf. interval]
-------------+----------------------------------------------------------------
attend | -.0017479
.0074481
-0.23
0.815
-.0163722
.0128764
priGPA |
.6843534
.0775414
8.83
0.000
.5321006
.8366063
skipped |
0 (omitted)
Your preview ends here
Eager to read complete document? Join bartleby learn and gain access to the full version
- Access to all documents
- Unlimited textbook solutions
- 24/7 expert homework help
_cons | -1.698644
.2101562
-8.08
0.000
-2.111287
-1.286001
------------------------------------------------------------------------------
.
Related Documents
Related Questions
The coefficient of correlation in a simple regression analysis is = -0.6. The coefficient of determination for this regression would be
0.36
- 0.36
0.6
0.13
O 0.6 or + 0.6
arrow_forward
Please see attached image.
In analyzing the effects of an after-school reading program, you run a regression analysis with program participation as the independent variable (0 = control group; 1 = intervention group) and scores on a reading comprehension exam after the program as the dependent variable.
Is the effect of the after-school reading program statistically significant? How can you tell, and what does this mean?
arrow_forward
SEE MORE QUESTIONS
Recommended textbooks for you
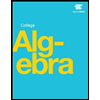
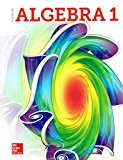
Glencoe Algebra 1, Student Edition, 9780079039897...
Algebra
ISBN:9780079039897
Author:Carter
Publisher:McGraw Hill
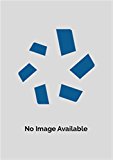
Big Ideas Math A Bridge To Success Algebra 1: Stu...
Algebra
ISBN:9781680331141
Author:HOUGHTON MIFFLIN HARCOURT
Publisher:Houghton Mifflin Harcourt
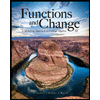
Functions and Change: A Modeling Approach to Coll...
Algebra
ISBN:9781337111348
Author:Bruce Crauder, Benny Evans, Alan Noell
Publisher:Cengage Learning
Related Questions
- The coefficient of correlation in a simple regression analysis is = -0.6. The coefficient of determination for this regression would be 0.36 - 0.36 0.6 0.13 O 0.6 or + 0.6arrow_forwardPlease see attached image. In analyzing the effects of an after-school reading program, you run a regression analysis with program participation as the independent variable (0 = control group; 1 = intervention group) and scores on a reading comprehension exam after the program as the dependent variable. Is the effect of the after-school reading program statistically significant? How can you tell, and what does this mean?arrow_forward
Recommended textbooks for you
- Glencoe Algebra 1, Student Edition, 9780079039897...AlgebraISBN:9780079039897Author:CarterPublisher:McGraw HillBig Ideas Math A Bridge To Success Algebra 1: Stu...AlgebraISBN:9781680331141Author:HOUGHTON MIFFLIN HARCOURTPublisher:Houghton Mifflin Harcourt
- Functions and Change: A Modeling Approach to Coll...AlgebraISBN:9781337111348Author:Bruce Crauder, Benny Evans, Alan NoellPublisher:Cengage Learning
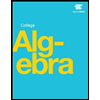
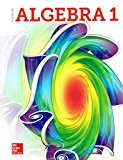
Glencoe Algebra 1, Student Edition, 9780079039897...
Algebra
ISBN:9780079039897
Author:Carter
Publisher:McGraw Hill
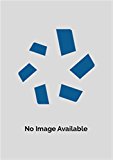
Big Ideas Math A Bridge To Success Algebra 1: Stu...
Algebra
ISBN:9781680331141
Author:HOUGHTON MIFFLIN HARCOURT
Publisher:Houghton Mifflin Harcourt
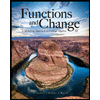
Functions and Change: A Modeling Approach to Coll...
Algebra
ISBN:9781337111348
Author:Bruce Crauder, Benny Evans, Alan Noell
Publisher:Cengage Learning