FinTech Assignment - DeFi
pdf
keyboard_arrow_up
School
University of Texas *
*We aren’t endorsed by this school
Course
6215
Subject
Economics
Date
Feb 20, 2024
Type
Pages
11
Uploaded by SuperHumanMorningTarsier43
FinTech Assignment DeFi
1
FinTech Assignment - DeFi
Suhas Yogish (SY23839)
1. Uniswap - Swapping and Liquidity
To calculate the price slippage for trading in a Uniswap-like liquidity pool, specifically for a WETH/USDC pair, I followed a series of steps based on the constant product formula that governs the pool's automated market maker AMM mechanism. This formula ensures that the product of the quantities of the two assets in the pool remains constant before and after a trade. Below is a detailed explanation of each step in the calculation process:
A. Determine Initial Reserves:
The Total Value Locked TVL in the pool is given as $1,200,000, and it is assumed to be equally divided between WETH and USDC. Therefore, the initial reserves are:
WETH Reserve:
Since half of the TVL is in WETH, and the current price of ETH is $2,370, the WETH reserve is calculated by dividing the half TVL by the price of ETH
USDC Reserve:
The other half of the TVL is in USDC, so the USDC reserve is simply half of the TVL
B. Calculate Initial Price:
The initial price of WETH in terms of USDC is determined by the ratio of the reserves:
C. Trade Impact and New Reserves:
When a trade is executed, the USDC reserve increases by the trade amount, and the WETH reserve decreases according to the constant product formula. The new reserves are calculated as follows:
For a $200,000 trade:
The USDC reserve increases by $200,000.
The new WETH reserve is calculated by rearranging the constant product formula to maintain the product of the reserves:
For a $2 trade:
The process is similar, with the USDC reserve increasing by $2 and calculating the new WETH reserve accordingly.
D. Calculate Final Price:
The final price after the trade is the ratio of the new reserves:
5. Determine Price Slippage:
Price slippage is the percentage difference between the initial and final prices, calculated as:
WETH Reserve =
=
Price of ETH
TVL/2
2,370
1,200,000/2
USDC Reserve = TVL/2 = 1,200,000/2
Initial Price =
WETH Reserve
USDC Reserve
New WETH Reserve =
New USDC Reserve
Constant Product
Final Price =
New WETH Reserve
New USDC Reserve
Slippage (%) =
×
(
Initial Price
Final Price − Initial Price
)
100%
FinTech Assignment DeFi
2
This calculation was applied to both trade scenarios $200,000 and $2, yielding slippage percentages that reflect the impact of trade size relative to the pool's liquidity. The slippage for the $200,000 trade was significantly higher at 77.78%
, indicating a substantial impact on the market price due to the relative size of the trade. Conversely, the slippage for the $2 trade was negligible at 0.00067%
, showing minimal market impact.
Swapping ETH for USDC
I swapped 0.00124 ETH for 3.0045 USDC.
I paid $0.44 USD in network costs/gas fees and under $0.01 in swap fees.
Adding to the Liquidity Pool
BaseScan Txn: https://basescan.org/tx/0xb4a725f5ce4ef8b3f895b7f49b7c749cfb0900402ebc370cd023ee9a584e9d23
FinTech Assignment DeFi
3
In order to maintain balance for fees, I decided to deposit $1.5 USDC (instead of $2.00 USDC.
With regards to fees, I paid the following amounts:
CoinBase authorization network fee $0.36 USD
Your preview ends here
Eager to read complete document? Join bartleby learn and gain access to the full version
- Access to all documents
- Unlimited textbook solutions
- 24/7 expert homework help
FinTech Assignment DeFi
4
Liquidity Pool Fee 0.05% fee tier
Removing Liquidity Pool
BaseScan Txn: https://basescan.org/tx/0x9b60c4402f9fdd29a00834c24385c375a0ba5e46d8f148ddbaf897bedd8c5a03
FinTech Assignment DeFi
5
Fees Yielded: $0.000226
Amount of ETH Received: 0.000574754752490328 $1.43 USD
Amount of USDC Received: $1.494
Position PnL Excl. Gas):
$2.92 $2.94 $0.02
In conclusion, I earned a total of $0.000226
in fees, and I lost $0.02
overall. This can be attributed to several reasons, such as fluctuations in the value of ETH and USDC throughout my investment period, my ability to collect a portion of the pool's fees, and the impact of substantial network and transaction costs.
2. Aave Supplying & Borrowing
The Compound protocol uses an interest rate model that aims to achieve equilibrium in each money market based on supply and demand principles. This model ensures that interest rates adjust in response to changing market conditions without the need for individual suppliers or borrowers to negotiate terms and rates. Key components of this model include:
Utilization Ratio U
This ratio unifies supply and demand into a single variable for each market, calculated as the total borrowed amount divided by the sum of the cash available and the borrowed amount
U
=
Cash + Borrows
Borrows
FinTech Assignment DeFi
6
Demand Curve:
The demand for borrowing is codified through governance and expressed as a function of the utilization ratio. This allows the borrowing interest rates to adjust based on the market's utilization level. For instance, a borrowing interest rate in a given market might be determined by a base rate plus a variable rate that's multiplied by the utilization ratio. For example:
Supplier Interest Rate:
The interest rate earned by suppliers (those providing liquidity) is indirectly determined and equals the borrowing interest rate multiplied by the utilization rate. This ensures that as the demand for borrowing increases (and thus the borrowing interest rates), suppliers benefit from higher returns on their supplied assets.
The spike in rates on Aave in October 2023 can be attributed to a broader rebound in the decentralized finance DeFi) sector, where there was a significant increase in loan volume and overall DeFi activity. This rebound was characterized by a record $182M of assets borrowed on Aave v3 on Ethereum in a single day, driven by traders' increased risk appetite and optimism in the crypto market. This optimism was partly due to speculation about the approval of a spot Bitcoin ETF and a more favorable macroeconomic environment. The demand for leverage grew as people rushed to speculate, which in turn drove up the borrowing rates due to the increased demand for borrowing assets.
Supplying ETH and Borrowing USDC
After converting all my USDC to ETH on Uniswap, I proceeded to supply my ETH on the Aave platform. I supplied a total of 0.0004143 ETH which was worth $1.01 USD. I will be yielding 1.47% APY on my investment but this a variable rate and could change when the supply of ETH changes on Aave.
Supply Txn: https://basescan.org/tx/0x8e3025e4f6891cfc29994e06f5f88b2088f44df369084b5ecd60e4f24d67750a
Borrowing Interest Rate = 2.5% +
U
× 20%
Your preview ends here
Eager to read complete document? Join bartleby learn and gain access to the full version
- Access to all documents
- Unlimited textbook solutions
- 24/7 expert homework help
FinTech Assignment DeFi
7
After supplying the ETH, I borrowed 50% of the ETH supply i.e. $0.50 USD as USDC tokens as shown below. The borrowing APY on the transaction was 4.59% which is also a variable rate similar to the supply APY.
Borrow Txn: https://basescan.org/tx/0x872f9f04057e5993e272286b56671e4eea22e1bc52a7d2e5ddb53bc008920005
The health factor seen on the transaction overview above is a metric used to measure the safety of the borrowed loan. Itʼs generally calculated as the ratio of the supply/collateral to the loan amount. When the health factor falls below 1, the collateral amount will be liquidated to payback the lenders. The health factor is a variable metric and itʼs value is dependent on the collateral amount and borrowed loan amount. The health factor change can be simplified using the formula below:
From this formula, you can see that as the borrowing amount increases, the health factor decreases, assuming the total collateral value and the liquidation threshold remain constant. This means the risk of liquidation increases with higher borrow amounts because we are closer to the threshold at which our collateral could be liquidated to cover the loan.
Health Factor =
Total Borrow Amount
Total Collateral Value x Liquidation Threshold
FinTech Assignment DeFi
8
Repaying USDC and Withdrawing ETH
I waited for about 2.5 hrs before I proceeded with repaying the borrowed USDC. I had accrued a total of $0.00002 USD in interest on my borrowing and had a balance of $0.50002 USDC. I repaid the borrowed loan using my wallet balance, however I was not able to completely repay the amount due to insufficient ETH balance to cover transaction and network costs resulting in 0.0001 USDC remaining as borrowed.
3. DCF Valuation of a DeFi Protocol
For this question, I have decided to value the Aave protocol. Aave is a leading decentralized finance protocol that enables users to lend and borrow cryptocurrencies without a centralized intermediary, operating on an open-source liquidity protocol basis. The protocol is built on the Ethereum network, allowing for a wide variety of digital assets to be borrowed and lent, from stablecoins to altcoins, governed by AAVE token holders. Aave's origins trace back to 2017 with the launch of ETHLend, which evolved into Aave in 2020 after a complete overhaul during the bear market. This transition allowed Aave to address previous issues such as liquidity and loan matching, establishing itself as a significant player in the DeFi space.
Aave's competitive advantages include its decentralized governance model, algorithmic money market mechanism, and innovative features like flash loans, which allow for uncollateralized loans within a single transaction. The protocol's ability to offer loans in different cryptocurrencies than the deposited ones adds to its flexibility and attractiveness for users. Moreover, Aave has expanded its offerings beyond money markets, engaging in areas like NFT gaming with Aavegotchi, highlighting its adaptability and forward-thinking approach.
Regarding the macro and industry economic trends, Aave's growth and challenges can be influenced by the broader adoption of DeFi, regulatory environments, and the inherent volatility of cryptocurrencies. The protocol's success depends on maintaining high liquidity levels, ensuring system robustness against black swan events, and navigating the capital inefficiency issues due to the overcollateralization requirement in DeFi lending. Aave's ability to innovate and adapt to changing market conditions and regulatory landscapes will be crucial for its sustained growth and competitiveness
Forecasting Revenues and Costs
The DeFi industry is poised for substantial growth, with forecasts suggesting a 47.5% revenue increase to $26.15 billion in 2024, driven by factors like regulatory developments and the broader adoption of DeFi services. Aave, as a leading DeFi protocol, is expected to potentially outperform the market's average growth rate due to its strong position in the sector. While operational expenses for Aave are likely to scale with revenue growth, leveraging economies of scale, strategic investments and expansions might lead to higher short-term costs to support long-term growth, reflecting a cautiously optimistic outlook for Aave's future revenue projections amidst the competitive and regulatory landscape of the DeFi space.
Based on the assumptions and the projected growth rate in the DeFi sector, here are the forecasted revenues, costs, and Free Cash Flows FCF for Aave over the next 5 years:
Year
Revenue Million USD
Costs Million USD
Free Cash Flow Million USD
1
102.91
9.26
93.65
2
151.79
13.66
138.13
3
223.90
20.15
203.74
4
330.25
29.73
300.52
5
487.11
43.84
443.27
import numpy as np
import pandas as pd
# Assumptions
revenue_growth_rate = 0.475 # DeFi market's projected growth of 47.5% for 2024
cost_growth_rate = 0.475 # Assuming costs grow at the same rate as revenue
initial_revenue = 69.77 # in million USD
initial_costs = 6.28 # in million USD
forecast_years = 5 # Forecast period
FinTech Assignment DeFi
9
# Forecast Revenues and Costs
years = np.arange(1, forecast_years + 1)
revenues = initial_revenue * (1 + revenue_growth_rate) ** years
costs = initial_costs * (1 + cost_growth_rate) ** years
# Calculating Free Cash Flows (FCF)
fcf = revenues - costs
# Display the forecasted revenues, costs, and FCF
forecast_df = pd.DataFrame({
'Year': years,
'Revenue (M USD)': revenues,
'Costs (M USD)': costs,
'Free Cash Flow (M USD)': fcf
})
forecast_df
Computing beta of the AAVE token against S&P500
The beta of Aave token relative to the S&P 500 ETF SPY is approximately 2.20
. This indicates that Aave is more volatile than the market SPY, with its price movements being over twice as reactive to market changes.
import pandas as pd
import statsmodels.api as sm
# Load the cleaned CSV files for Aave and SPY
tickerSymbols = ['AAVE-USD']
endDate = datetime.datetime.now()
startDate = endDate - datetime.timedelta(days=2*365)
aave_prices_cleaned = pd.DataFrame()
for ticker in tickerSymbols:
tickerData = yf.Ticker(ticker)
tickerDf = tickerData.history(period='1d', start=startDate, end=endDate)
aave_prices_cleaned[ticker] = tickerDf['Close']
tickerSymbols = ['SPY']
endDate = datetime.datetime.now()
startDate = endDate - datetime.timedelta(days=2*365)
sp500_prices_cleaned = pd.DataFrame()
for ticker in tickerSymbols:
tickerData = yf.Ticker(ticker)
tickerDf = tickerData.history(period='1d', start=startDate, end=endDate)
sp500_prices_cleaned[ticker] = tickerDf['Close']
# Convert the 'Date' columns to datetime format and normalize to remove time for alignment
aave_prices_cleaned['Date'] = pd.to_datetime(aave_prices_cleaned['Date']).dt.normalize()
sp500_prices_cleaned['Date'] = pd.to_datetime(sp500_prices_cleaned['Date']).dt.normalize()
# Merge the datasets on the 'Date' column to align them
merged_data_cleaned = pd.merge(aave_prices_cleaned, sp500_prices_cleaned, on='Date', how='inner
Your preview ends here
Eager to read complete document? Join bartleby learn and gain access to the full version
- Access to all documents
- Unlimited textbook solutions
- 24/7 expert homework help
FinTech Assignment DeFi
10
# Calculate daily returns for Aave and SPY
merged_data_cleaned['AAVE_Returns'] = merged_data_cleaned['AAVE-USD'].pct_change()
merged_data_cleaned['SPY_Returns'] = merged_data_cleaned['SPY'].pct_change()
# Drop the first row since it will have NaN values for returns
merged_data_cleaned = merged_data_cleaned.dropna()
# Prepare data for regression
X_cleaned = merged_data_cleaned['SPY_Returns']
y_cleaned = merged_data_cleaned['AAVE_Returns']
X_cleaned = sm.add_constant(X_cleaned) # Adds a constant term to the predictor
# Perform the regression with the cleaned data
model_cleaned = sm.OLS(y_cleaned, X_cleaned)
results_cleaned = model_cleaned.fit()
# The beta of Aave relative to SPY with the cleaned data
beta_aave_cleaned = results_cleaned.params[1]
# Display the beta value and the summary of the regression results
print(f"Beta of Aave: {beta_aave_cleaned}")
print(results_cleaned.summary())
Capital Asset Pricing Model (CAPM)
Standard assumptions are:
Risk-free rate (
Rf
The yield on a 10-year U.S. Treasury bond is used. Iʼll use 1.75% for this exercise.
Expected market return (
Rm
Historically, the S&P 500 has returned about 7% to 10% annually over long periods. Iʼll use 8% for this calculation.
Using the CAPM model with the given assumptions and the calculated beta of Aave 2.20, the discount rate Cost of Equity) for Aave is approximately 15.5%
.
# Standard assumptions for CAPM components
risk_free_rate = 0.0175 # 1.75%
expected_market_return = 0.08 # 8%
# Beta of Aave as calculated from the regression
beta_aave = 2.20
# CAPM formula to calculate the discount rate (Cost of Equity)
discount_rate = risk_free_rate + beta_aave * (expected_market_return - risk_free_rate)
print(discount_rate)
Discounted FCFF and Terminal Value
Iʼve assumed a conservative terminal growth rate of 2.5%
, which is slightly above the long-term inflation rate but reflects a mature phase of growth. The present value PV of the forecasted Free Cash Flows FCF for Aave over the 5-year forecast period, along with the Terminal Value TV discounted to present value, are as follows:
Year
PV of FCFF Million USD
1
81.08
2
103.54
3
132.23
4
168.87
FinTech Assignment DeFi
11
Year
PV of FCFF Million USD
5
215.65
Present Value of Terminal Value: $1,700.34 Million USD
Total Present Value: $2,401.72 Million USD
Based on the DCF valuation I performed, the intrinsic value of the Aave protocol is estimated to be approximately $2.402 billion
. When compared to Aave's current market capitalization of $1.259 billion
, the intrinsic value is significantly higher. This suggests that, according to our DCF model and the assumptions used, Aave appears to be undervalued by the market could present a potential investment opportunity.
Related Documents
Recommended textbooks for you
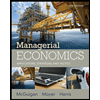
Managerial Economics: Applications, Strategies an...
Economics
ISBN:9781305506381
Author:James R. McGuigan, R. Charles Moyer, Frederick H.deB. Harris
Publisher:Cengage Learning
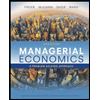
Managerial Economics: A Problem Solving Approach
Economics
ISBN:9781337106665
Author:Luke M. Froeb, Brian T. McCann, Michael R. Ward, Mike Shor
Publisher:Cengage Learning
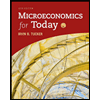
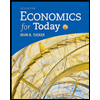
Recommended textbooks for you
- Managerial Economics: Applications, Strategies an...EconomicsISBN:9781305506381Author:James R. McGuigan, R. Charles Moyer, Frederick H.deB. HarrisPublisher:Cengage LearningManagerial Economics: A Problem Solving ApproachEconomicsISBN:9781337106665Author:Luke M. Froeb, Brian T. McCann, Michael R. Ward, Mike ShorPublisher:Cengage Learning
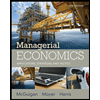
Managerial Economics: Applications, Strategies an...
Economics
ISBN:9781305506381
Author:James R. McGuigan, R. Charles Moyer, Frederick H.deB. Harris
Publisher:Cengage Learning
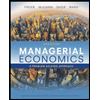
Managerial Economics: A Problem Solving Approach
Economics
ISBN:9781337106665
Author:Luke M. Froeb, Brian T. McCann, Michael R. Ward, Mike Shor
Publisher:Cengage Learning
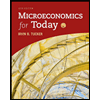
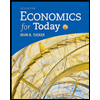