ST2023-EC220 - detailed solutions
pdf
keyboard_arrow_up
School
London School of Economics *
*We aren’t endorsed by this school
Course
220
Subject
Economics
Date
Nov 24, 2024
Type
Pages
16
Uploaded by ONYNO_
EC
Introduction to Econometrics
Exam Solutions
Summer
Section A
(Answer all questions. This section carries
/
of the overall mark.)
Question
[
.
marks]
Mining is dangerous. We are interested in understanding the relationship between the price of min-
erals and the number of mining accidents. There are two hypothesized mechanisms whereby an
increase in the price of a mineral may affect mining safety: ( ) safety may improve because mines
have more revenue and are able to put more funds into safety, ( ) the opportunity cost of safety is
higher, and mines may use more dangerous mining practices because they want to maximize pro-
duction when prices are high. We are curious about which effect is dominant. We gather data from
N mines. We have data on
y
i
, the number of accidents in the mine,
price
i
, the local market price of
the mineral that is mined, and
labour
i
, the number of labourers hired by the mine.
We consider a regression model
ln(
y
i
) =
β
0
+
β
1
ln(
price
i
) +
β
2
labour
i
+
β
3
labour
2
i
+
u
i
( . )
After estimating regression ( . ) with OLS, we obtain estimates of coef cients, shown in the Stata
output below.
(a)
Perform a t-test with the null hypothesis that the approximate elasticity of accidents with re-
spect to price is
, holding
xed labour and labour squared.
[ .
marks]
Solution :
Because
price
i
and
y
i
are both in logs,
β
1
is approximately interpreted as the elas-
ticity of accidents with respect to price holding
xed labour and labour squared. Thus we are
performing a t-test with
H
0
:
β
1
= 1
. The t-statistic is:
0
.
5751439
-
1
0
.
3267842
=
-
1
.
300
©
LSE ST
/EC
R
Page
of
6
| -
1
.
300
|
<
1
.
96
We fail to reject
H
0
at the
% signi cance level.
(b)
At present, the regression equation allows for a quadratic relationship between
labour
i
and
ln(
y
i
)
. We want to change this. How could we use dummy variables to write a regression
equation that allows for an effect of
labour
i
on
ln(
y
i
)
that differs in
ranges: ( )
labour
i
tak-
ing values from
to
, ( )
labour
i
taking values
to
, and ( )
labour
i
taking values
or greater?
(In case it is not clear, the regression you write should allow for the effect of
labour
i
on
ln(
y
i
)
to change depending on which category
labour
i
is in, but, within a category, changing
labour
i
doesn’t affect
y
i
. E.g.
labour
i
being
rather than
should have an effect, but
labour
i
being
rather than
should not have an effect).
[6 marks]
Solution :
Let us de ne dummy variables,
x
1
i
= 1[
labour
i
≤
99]
,
x
2
i
= 1[100
≤
labour
i
≤
999]
, and
x
3
i
= 1[1000
≤
labour
i
]
which are dummy variables that
labour
i
takes a value in
the range shown in brackets. One possible regression equation is:
ln(
y
i
) =
β
0
+
β
1
ln(
price
i
) +
β
2
x
1
i
+
β
3
x
2
i
+
u
i
Alternatively, one of
x
1
or
x
2
could be excluded and
x
3
could be included. Or instead, the con-
stant could be excluded and
x
3
included.
For the rest of the exam, ignore any change to the regression equation you may have written in (b).
(c)
Our data on price is the local market price at the time accidents are measured. Mines often
have contracts for prices that are signed many months in advance. Thus, the market price
recorded in our data may not be the price the mine receives. Describe how this could affect
estimates. Regardless of whether you believe this will cause bias, also describe any other po-
tential source of bias that may be a concern in regression ( ). Discuss the direction of the bias
and the intuition of each.
[8 marks]
Solution :
We observe
ln(
price
i
) = ln(
price
i
)
*
+
w
i
where
ln(
price
i
)
*
is the true natural log
of the price received by the mine and
w
i
is measurement error. Classical measurement error
is when
w
i
is uncorrelated with all regressors and with the regression error. In this case, we
have attenuation bias, and our estimate is biased toward
,
|
b
β
1
|
< β
1
. Because our estimate
is positive, the bias is negative.
If the measurement error is not classical, then measurement error will in general cause bias,
however, the direction of the bias will depend on the form of the measurement error.
Are there any other sources of bias to be concerned about? Reverse causality is probably not
a concern. The number of accidents in a single mine should not be causing the market price
of a mineral to change. A student could conceivably argue that accidents reduce production,
lower supply, and affect the market price. This is likely a minimal effect, but would cause pos-
itive bias because the outcome increasing (accidents going up) is associated with lower sup-
ply, and thus higher prices (treatment going up). Credit is given if the discussion is logical, but
students do not need to claim there is bias due to reverse causality. There may be other con-
founders. For example, the presence of toxic fumes or the use of explosive equipment makes
a mine less safe, and causes the number of accidents to go up. Mines that use such explosive
equipment might also be mining in a region where the geology requires this, and the miner-
©
LSE ST
/EC
R
Page
of
6
als in such a region may be more (conceivably less) expensive. In this case, the confounder
causes both
ln(
price
i
)
and
y
i
to increase, causing positive bias.
(d)
Our price variable is the local market price for a mine. For each mineral, there are multiple
mines in our data mining that mineral. We create a new variable,
averageother
i
, which is the
average of the log price for all other mines that mine the same mineral as
i
. Describe intu-
itively and mathematically how
averageother
i
could be used as an instrument for
ln(
price
i
)
.
What biases discussed in question (c) might it solve?
[
marks]
Solution :
Intuition: The goal of an IV is to create “good” variation in the regressor such that
we overcome bias.
averageother
i
is probably correlated with
ln(
price
i
)
because prices of
the same mineral are probably correlated across geographic markets, thus the instrument will
create variation, but is it good variation? The average price in other markets is probably not
correlated with measurement error (the difference between the market price and the contract
price for a single mine), thus our instrument creates variation which is plausibly uncorrelated
with measurement error, and will help overcome that bias.
The other major concern about bias was confounders, that factors (such as using explosive
equipment) might be correlated with price and also accidents. The instrument probably does
not help us overcome this bias. If one mine uses explosive equipment to mine a mineral, other
mines that mine the same mineral probably also use explosive equipment, which is correlated
with high prices. Thus, the variation created by the instrument would still be correlated with
unsafe mining practices, and the bias would not be overcome.
Mathematically: The
rst stage regression is:
ln(
price
i
) =
δ
0
+
δ
1
averageother
i
+
δ
2
labour
i
+
δ
2
labour
2
i
+
i
We create predicted values from this,
\
ln(
price
i
)
. This represents the portion of
ln(
price
i
)
that
is predicted by our instrument (hopefully having “good” variation that doesn’t cause bias). We
then use these predicted values instead of the original data for
ln(
price
i
)
in the original equa-
tion of interest, known as the second stage,
ln(
y
i
) =
β
0
+
β
1
\
ln(
price
i
) +
β
2
labour
i
+
β
3
labour
2
i
+
u
i
If a student wrote about bias due to reverse causality in (c), then in the answer to (d) the stu-
dent should comment on this as well. Such bias is probably overcome. Accidents in a mine
are probably not correlated with the price of the mineral in other regions, so the instrument
creates variation that is exogenous to the variation that causes bias. A student could argue
that accidents in a mine affect prices in other markets, but I think this would be hard to justify.
(e)
What assumptions are needed for the IV approach in (d) to give causal estimates? Discuss if
they seem valid in context. Which assumptions can be tested (if any)?
[8 marks]
Solution :
Relevance and Exogeneity (which comprises “as good as randomly assigned” and
“exclusion”).
Relevance:
averageother
i
is correlated with
ln(
price
i
)
. This is certainly true because price of
a mineral will be correlated across geographic markets. Relevance can be tested by evaluat-
ing if the estimate for
δ
1
is signi cant in the
rst stage regression.
Exogeneity:
Cov
(
u
i
, averageother
i
) = 0
. The instrument is uncorrelated with the error term
©
LSE ST
/EC
R
Page
of
6
Your preview ends here
Eager to read complete document? Join bartleby learn and gain access to the full version
- Access to all documents
- Unlimited textbook solutions
- 24/7 expert homework help
in the regression of interest.
“Exclusion” – the only channel whereby
averageother
i
affects
y
i
is through
ln((
price
i
)
. This
might fail if other regions having higher (or lower) prices causes a mine to more aggressively
produce, leading to more accidents. This seems unlikely, but should be considered.
“As good as randomly assigned” –
averageother
i
is uncorrelated with all unobserved deter-
minants of
y
i
. The concern regarding this was described in the answer to (d) regarding safety
(use of explosives) when mining certain minerals.
Students should describe potential concerns with each subassumption, but do not need to
describe these speci c potential concerns.
Exogeneity cannot be tested because the error term,
u
i
, is not observable.
©
LSE ST
/EC
R
Page
of
6
Section B
(Answer all questions. This section carries
/
of the overall mark.)
Question
[
.
marks]
(a)
Consider a
multiple regression
model with
k
+ 1
regressors (including intercept):
MLR.
The population model is
y
=
β
0
+
β
1
x
1
+
...
+
β
k
x
k
+
u
.
MLR.
We have a random sample,
{
(
y
i
, x
i
1
, ..., x
ik
) :
i
= 1
, ..., n
}
, from the model.
MLR.
We have no perfect collinearity among any of the regressors.
MLR.Wild
The regressors are “unrelated” to the error term (in a vague unspeci ed sense).
MLR.
The error term
u
is such that
V ar
(
u
|
x
1
, ..., x
k
) =
V ar
(
u
) =
σ
2
>
0
.
(i)
State the
rst-order conditions for deriving ordinary least squares (OLS) estimators of the
β
parameters. Hence, or otherwise, compare and contrast the OLS approach for estima-
tion of the
β
parameters with the method of moments (MM) approach.
[
marks]
Solution :
(rescale marks
to
)
n
X
i
=1
(
y
i
-
ˆ
β
0
-
ˆ
β
1
x
i
1
- · · · -
ˆ
β
k
x
ik
)
=
0
n
X
i
=1
x
i
1
(
y
i
-
ˆ
β
0
-
ˆ
β
1
x
i
1
- · · · -
ˆ
β
k
x
ik
)
=
0
.
.
.
n
X
i
=1
x
ik
(
y
i
-
ˆ
β
0
-
ˆ
β
1
x
i
1
- · · · -
ˆ
β
k
x
ik
)
=
0
(
mark)
For OLS, we choose
ˆ
β
0
,
ˆ
β
1
, . . . ,
ˆ
β
k
to minimise
n
X
i
=1
(
y
i
-
b
0
-
b
1
x
i
1
- · · · -
b
k
x
ik
)
2
with respect to
b
0
, b
1
, . . . , b
k
.
(
mark)
On the other hand, in the MM approach, (i) we make assumptions about population mo-
ments (this yields equations involving our unknown parameters); (ii) we estimate popula-
tion moments with their sample counterparts; (iii) we then solve the equations to obtain
estimators for our parameters.
(
mark)
Within the given regression setting, the MM approach yields the same set of
k
+ 1
mo-
ment equations as the system of
k
+ 1
FOC conditions from the LS approach. Hence the
estimators will be equivalent.
(
mark)
(ii)
Explain how the OLS estimator of
β
1
, say
ˆ
β
1
, can be obtained from a simple regression of
y
on some suitably-constructed univariate regressor rather than a multiple regression of
y
on an intercept and the full set of regressors
x
1
, x
2
, ..., x
k
.
[
marks]
©
LSE ST
/EC
R
Page
of
6
Solution :
(rescale marks
to
) We have the so-called regression anatomy formula to
express
ˆ
β
1
as:
ˆ
β
1
=
∑
n
i
=1
ˆ
r
i
1
y
i
∑
n
i
=1
ˆ
r
2
i
1
where
ˆ
r
i
1
is the OLS residual for regression of
x
i
1
on all
k
other regressors.
ˆ
β
1
is interpreted as the estimated coef cient from a regression of
y
on
x
j
after the ef-
fects of all
k
other regressors are partialled out.
(
mark for formula,
mark for explaining
ˆ
r
i
1
clearly,
mark for giving the partialling out interpretation.)
For simplicity, let us now consider the
simple regression model
obtained by setting
k
= 1
in
MLR. –MLR.
above.
(iii) Derive the sampling error of
ˆ
β
1
, providing full step-by-step algebraic detail.
[ .
marks]
Solution :
(rescale marks
to
.
) [So long as it is correct and detailed, a full mark
answer does not have to match the exact structure presented below.]
We drop the second subscript on
x
i
1
since there is only one non-constant regressor.
Step
:
Write down formula for
ˆ
β
1
. It is convenient to use
ˆ
β
1
=
∑
n
i
=1
(
x
i
-
¯
x
)
y
i
∑
n
i
=1
(
x
i
-
¯
x
)
2
which is one of several equivalent forms. Let
SST
x
=
∑
n
i
=1
(
x
i
-
¯
x
)
2
and write
ˆ
β
1
=
∑
n
i
=1
(
x
i
-
¯
x
)
y
i
SST
x
Existence of
ˆ
β
1
is guaranteed by MLR.
(
mark)
Step
:
Replace
y
i
with
β
0
+
β
1
x
i
+
u
i
(which uses MLR. – )
•
Numerator of
ˆ
β
1
becomes
n
X
i
=1
(
x
i
-
¯
x
)
y
i
=
n
X
i
=1
(
x
i
-
¯
x
)(
β
0
+
β
1
x
i
+
u
i
)
=
β
0
n
X
i
=1
(
x
i
-
¯
x
) +
β
1
n
X
i
=1
(
x
i
-
¯
x
)
x
i
+
n
X
i
=1
(
x
i
-
¯
x
)
u
i
=
β
1
SST
x
+
n
X
i
=1
(
x
i
-
¯
x
)
u
i
by
∑
n
i
=1
(
x
i
-
¯
x
) = 0
and
∑
n
i
=1
(
x
i
-
¯
x
)
x
i
=
∑
n
i
=1
(
x
i
-
¯
x
)
2
©
LSE ST
/EC
R
Page 6 of
6
Your preview ends here
Eager to read complete document? Join bartleby learn and gain access to the full version
- Access to all documents
- Unlimited textbook solutions
- 24/7 expert homework help
(
marks)
Step
:
Decompose our estimator as follows
•
So we get
ˆ
β
1
=
β
1
SST
x
+
∑
n
i
=1
(
x
i
-
¯
x
)
u
i
SST
x
=
β
1
+
∑
n
i
=1
(
x
i
-
¯
x
)
u
i
SST
x
•
Now de ne
w
i
=
(
x
i
-
¯
x
)
SST
x
•
Then we have
ˆ
β
1
=
β
1
+
n
X
i
=1
w
i
u
i
•
Sampling error
ˆ
β
1
-
β
1
is written by a linear function of
u
i
’s
•
w
i
’s are all functions of
X
=
{
x
1
, . . . , x
n
}
(
mark)
(iv) Propose the weakest form of MLR.Wild that would be needed to establish consistency of
ˆ
β
1
for
β
1
, and call it
MLR.Weak
. Prove consistency under MLR.Weak.
[
marks]
Solution :
(rescale marks from 6 to
)
We drop the second subscript on
x
i
1
since there is only one non-constant regressor.
Our assumption MLR.Weak is: (i)
E
(
u
) = 0
, and (ii)
E
(
xu
) = 0
.
(
marks)
OLS estimator is written as:
ˆ
β
1
=
β
1
+
n
-
1
∑
n
i
=1
(
x
i
-
¯
x
)
u
i
n
-
1
∑
n
i
=1
(
x
i
-
¯
x
)
2
Look at numerator of sampling error term:
n
-
1
n
X
i
=1
(
x
i
-
¯
x
)
u
i
=
1
n
n
X
i
=1
x
i
u
i
-
¯
x
¯
u
By LLN,
plim
(¯
x
)
=
E
(
x
)
plim
(¯
u
)
=
E
(
u
) = 0
plim
1
n
n
X
i
=1
x
i
u
i
!
=
E
(
xu
) = 0
(
marks)
By properties of the plim operator,
plim
(
ˆ
β
1
)
=
β
1
+
plim
(
1
n
∑
n
i
=1
x
i
u
i
)
-
plim
(¯
x
¯
u
)
plim
(
1
n
∑
n
i
=1
(
x
i
-
¯
x
)
2
)
=
β
1
+
E
(
xu
)
-
E
(
x
)
E
(
u
)
V ar
(
x
)
=
β
1
©
LSE ST
/EC
R
Page
of
6
(
marks)
(v)
Propose how to strengthen MLR.Weak to establish unbiasedness of
ˆ
β
1
for
β
1
, and call the
new assumption
MLR.Strong
. Prove unbiasedness under MLR.Strong.
[
marks]
Solution :
(rescale marks from 6 to
)
We drop the second subscript on
x
i
1
since there is only one non-constant regressor.
Our assumption MLR.Strong is:
E
(
u
i
|
x
i
) = 0
. Together with MLR. , this will suf ce for
unbiasedness.
(
marks)
•
We have
E
(
ˆ
β
1
|
X
)
=
E
β
1
+
n
X
i
=1
w
i
u
i
X
!
=
β
1
+
n
X
i
=1
E
(
w
i
u
i
|
X
)
=
β
1
where we use linearity of the expectations operator
(
marks)
•
Thus, for any
X
, we get the same conditional expectation
E
(
ˆ
β
1
|
X
) =
β
1
and the law of iterated expectations implies
E
(
ˆ
β
1
) =
β
1
(
marks)
(b)
Suppose we have an “original”
tted regression given by
log
Y
i
=
b
1
+
b
2
log
X
i
+
e
i
,
where
b
1
and
b
2
are coef cients estimated via OLS, and
e
i
is the OLS residual, for
i
= 1
, ..., n
.
Now suppose units of measurement change so that
X
*
i
=
λX
i
, where
λ
is some non-zero
nite constant, and that
log
Y
i
is regressed on
and
log
X
*
i
. Our “new”
tted regression is
log
Y
i
=
b
*
1
+
b
*
2
log
X
*
i
+
e
*
i
,
where
b
*
1
and
b
*
2
are new coef cients estimated via OLS, and
e
*
i
is the new OLS residual.
(i)
Derive the relationship between the original and new coef cients estimated via OLS.(
Hint:
Start by showing that
log
X
*
i
-
log
X
*
= log
X
i
-
log
X,
where a bar denotes the sample mean.)
[
marks]
©
LSE ST
/EC
R
Page 8 of
6
Solution :
(rescale marks from
to
) Following the hint, we have
log
X
*
i
-
log
X
*
= log
λX
i
-
1
n
n
X
j
=1
log
X
*
j
= log
λX
i
-
1
n
n
X
j
=1
log
λX
j
= log
λ
+ log
X
i
-
1
n
n
X
j
=1
(log
λ
+ log
X
j
) = log
X
i
-
1
n
n
X
j
=1
log
X
j
= log
X
i
-
log
X
Hence
b
*
2
=
b
2
.
(
marks)
We will also need
b
*
1
.
b
*
1
=
log
Y
-
b
*
2
log
X
*
=
log
Y
-
b
2
1
n
n
X
j
=1
(log
λ
+ log
X
j
)
=
log
Y
-
b
2
log
λ
-
b
2
log
X
=
b
1
-
b
2
log
λ.
(
marks)
(ii)
Derive the relationship between the original and new OLS residuals.
[
mark]
Solution :
(rescale marks from
to
) The residual
e
*
i
is given by
e
*
i
= log
Y
i
-
b
*
1
-
b
*
2
log
X
*
i
= log
Y
i
-
(
b
1
-
b
2
log
λ
)
-
b
2
(log
X
i
+ log
λ
) =
e
i
.
(iii) Explain how the
t
-statistics (for the non-intercept regressor only) and the
R
2
for the two
regressions, original and new, are related.
[
marks]
Solution :
(rescale marks from
to
) Hence the estimator of the variance of the distur-
bance term is unchanged and so the standard error of
b
*
2
is the same as that for
b
2
. As a
consequence, the
t
statistic must be the same.
R
2
must also be the same:
R
2
*
= 1
-
∑
e
*
2
i
∑ (
log
Y
i
-
log
Y
)
= 1
-
∑
e
2
i
∑ (
log
Y
i
-
log
Y
)
=
R
2
.
[Students don’t have to give the formula for
R
2
for full marks. Intuition is enough.]
©
LSE ST
/EC
R
Page
of
6
Your preview ends here
Eager to read complete document? Join bartleby learn and gain access to the full version
- Access to all documents
- Unlimited textbook solutions
- 24/7 expert homework help
Question
[
.
marks]
(a)
Suppose that you are hired as class teacher for an undergraduate econometrics course and
that your students ask you the questions listed below. Please provide suitable answers.
(i)
“Can you provide a real (or hypothetical) example of heteroscedasticity?”
[
marks]
Solution :
(rescale marks from
to
)Any sensible example that reveals an understand-
ing of heteroscedasticity should earn full marks. It can be algebra and words, or a dia-
gram and words, or even just well-explained words alone.
(ii)
“What is the Gauss-Markov theorem? Why is it useful?”
[
marks]
Solution :
(rescale marks from
to
)Using the language of Question
(a), under MLR. –
MLR. , MLR.Strong and MLR. , ...
(
mark)
the OLS estimator is the Best Linear Unbiased Estimator (BLUE).
(
mark)
If we take any linear unbiased estimator
˜
β
1
, then it always holds
V ar
(
ˆ
β
1
|
X
)
≤
V ar
(
˜
β
1
|
X
)
(
mark)
Implication: If we are interested in linear unbiased estimators, then we need look no fur-
ther than OLS
(
mark)
(iii) “Say I estimate a simple regression model via OLS. How do I prove that
tted values of
the dependent variable are uncorrelated with the OLS residuals?”
[
marks]
Solution :
(rescale marks from
to
) The numerator of the sample correlation coef -
cient for
ˆ
Y
and
e
can be decomposed as follows, using the fact that
¯
e
= 0
:
1
n
n
X
i
=1
ˆ
Y
i
-
ˆ
Y
(
e
i
-
¯
e
) =
1
n
n
X
i
=1
(
[
b
1
+
b
2
X
i
]
-
b
1
+
b
2
¯
X
)
e
i
=
1
n
b
2
n
X
i
=1
(
X
i
-
¯
X
)
e
i
= 0
by OLS
rst-order conditions. Hence the correlation is zero.
(iv) “I want to test for joint signi cance of all regressors excluding the intercept in a multiple
regression model. What assumptions will I need? What test statistic shall I use? What is
the distribution of the test statistic under the null? What rejection rule should I use?”
[ .
marks]
Solution :
(rescale marks from
to
.
) Using the language of Wooldridge (and lecture
notes), we will need assumptions MLR
(linearity), MLR
(random sampling), MLR
(no
perfect collinearity), MLR
(strict exogeneity), MLR
(homoscedasticity), and MLR6 (nor-
mality).
(
mark)
©
LSE ST
/EC
R
Page
of
6
The test statistic is
V
=
(
SSR
0
-
SSR
1
)
/k
SSR
1
/
(
n
-
k
-
1)
,
where
n
is the number of observations,
SSR
0
and
SSR
1
are the restricted and unre-
stricted residual sums of squares respectively, and
k
is the number of non-intercept re-
gressors.
(
marks)
The distribution under the null is
F
(
k, n
-
k
-
1)
.
(
mark)
We reject if and only if
V > V
(1
-
α
)
, where
V
(1
-
α
)
is the
100(1
-
α
)
-th percentile on the
CDF of the
F
(
k, n
-
k
-
1)
distribution, and
α
∈
(0
,
1)
is a given signi cance level.
(
mark)
(b)
Let us consider the following system of simultaneous equations
y
1
=
α
1
y
2
+
α
2
x
1
+
α
3
x
2
+
u
1
( . )
y
2
=
β
1
y
1
+
β
2
x
1
+
β
3
x
3
+
β
4
x
4
+
u
2
,
( . )
where
(
u
1
, u
2
)
are i.i.d. errors with zero mean,
V ar
(
u
1
) =
σ
2
1
, V ar
(
u
2
) =
σ
2
2
, and
Cov
(
u
1
, u
2
) =
σ
12
.
The endogenous variables are
(
y
1
, y
2
)
and
(
x
1
, x
2
, x
3
, x
4
)
are exogenous variables.
(i)
Explain the simultaneity issue associated with the above simultaneous equation model
(SEM) intuitively (no derivations expected). Outline a real-world scenario for which the
given model may be useful.
[
marks]
Solution :
(rescale marks from
to
) The problem here is reverse causality: both equa-
tions have their own ceteris paribus interpretation, the simultaneity gives rise to a corre-
lation between the two endogenous variables that are jointly determined in this system
which makes it that when we estimate these structural equation in isolation by OLS we
get inconsistency. One example (discussed) is police&crime.
(ii)
You are told that in the above SEM there exists a perfect linear relation between
x
2
and
x
3
,
in particular,
x
3
= 2
x
2
.
Obtain the reduced form equations for
y
1
and
y
2
, recognising this exact linear relation
between
x
2
and
x
3
.
[
marks]
Solution :
(rescale marks from 6 to
) Let us
rst rewrite the SEM accounting for this
perfect linear relation:
y
1
=
α
1
y
2
+
α
2
x
1
+
α
3
x
2
+
u
1
( . )
y
2
=
β
1
y
1
+
β
2
x
1
+ 2
β
3
x
2
+
β
4
x
4
+
u
2
,
( . )
Substitute ( . ) into ( . ) we have:
y
1
=
α
1
(
β
1
y
1
+
β
2
x
1
+ 2
β
3
x
2
+
β
4
x
4
+
u
2
) +
α
2
x
1
+
α
3
x
2
+
u
1
(1
-
α
1
β
1
)
y
1
= (
α
1
β
2
+
α
2
)
x
1
+ (
α
3
+ 2
α
1
β
3
)
x
2
+
α
1
β
4
x
4
+
u
1
+
α
1
u
2
y
1
=
(
α
1
β
2
+
α
2
)
x
1
+ (
α
3
+ 2
α
1
β
3
)
x
2
+
α
1
β
4
x
4
+
u
1
+
α
1
u
2
(1
-
α
1
β
1
)
,
©
LSE ST
/EC
R
Page
of
6
which requires us to assume
α
1
β
1
6
= 1
. We write this reduced form as
y
1
=
π
11
x
1
+
π
12
x
2
+
π
13
x
4
+
v
1
Similarly, for
y
2
, substitute ( . ) into ( . ), we obtain:
y
2
=
β
1
(
α
1
y
2
+
α
2
x
1
+
α
3
x
2
+
u
1
) +
β
2
x
1
+ 2
β
3
x
2
+
β
4
x
4
+
u
2
y
2
=
(
β
1
α
2
+
α
2
)
x
1
+ (
β
1
α
3
+ 2
β
3
)
x
2
+
β
4
x
4
+
u
2
+
β
1
u
1
(1
-
α
1
β
1
)
.
We write this reduced form as
y
2
=
π
21
x
1
+
π
22
x
2
+
π
23
x
4
+
v
2
.
(iii) Use the result obtained in (b)ii to discuss the identi cation of the two structural equa-
tions. Clearly state whether the equations are over identi ed, exact (just) identi ed, or
under identi ed.
Hint:
Your answer is expected to discuss what conditions need to be satis ed to ensure
that we can use the observable data to estimate the parameters consistently.
[
marks]
Solution :
(rescale marks from
to
) Equation( . ) is just identi ed as we have
x
4
as
the exogenous variable to work as an instrument for the only endogenous variable (
y
2
).
x
2
and
x
3
cannot be used as instruments as they appear in both the equations. The con-
ditions are:
β
4
6
= 0
(relevance) and the exogeneity of
x
4
holds (
cov
(
x
4
, u
1
) = 0
).
Equation ( . ) is underidenti ed because we cannot use any of the regressors as an in-
strument to identify
β
1
following the multicollinearity between
x
2
and
x
3
.
(iv) Brie y comment on the importance of recognizing the existence of exact linear relations
among regressors in simultaneous equation models.
[
mark]
Solution :
(rescale from
marks to
) Recognizing the existence of exact linear relation-
ships is important as without it we would have claimed that ( . ) was overidenti ed and
( . ) was exact identi ed (this is related to the rank condition of identi cation [not ex-
pected students will make this statement].
©
LSE ST
/EC
R
Page
of
6
Your preview ends here
Eager to read complete document? Join bartleby learn and gain access to the full version
- Access to all documents
- Unlimited textbook solutions
- 24/7 expert homework help
Question
[
marks]
Consider the following linear regression model
y
t
=
β
0
+
ρy
t
-
1
+
β
1
z
t
-
1
+
β
2
z
t
-
2
+
u
t
with
|
ρ
|
<
1
t
= 1
, ..., n
( . )
where
{
y
t
}
and
{
z
t
}
are stationary and weakly dependent processes. We have a sample of
n
ob-
servations we can use to estimate this equation which we label
t
= 1
, ..., n
for convenience (this
means we assume that
y
0
,
z
0
, z
-
1
are available). There is no perfect multicollinearity in the regres-
sors.
(a)
Stationarity and weak dependence are important concepts that will allow us to apply the usual
laws of large numbers and CLT. Explain what the concepts of stationarity and weak depen-
dence mean.
[
marks]
Solution :
(rescale marks from
to
)Text book discussion of stationarity and weak depen-
dence (happy to accept students discussing the concept of covariance stationarity as long at
it is clearly indicated)
(
mark each)
.
The importance of these concepts are that they ensure that we can apply the usual laws of
large numbers and CLT, that will allows us to use asymptotic inference as in the cross sec-
tional setting (no need for normality, or strict exogeneity which is important in time series
analysis.
(
marks)
(b)
What (reasonable) assumptions do you need to make about the errors
u
t
to ensure that OLS
on equation ( . ) provides consistent estimators of the parameters
(
β
0
, β
1
, β
2
, ρ
)
? Provide a
clear explanation of the assumptions you make.
[
marks]
Solution :
(rescale marks from
to
) To ensure consistency, we need to assume contempo-
raneous exogeneity :
E
(
u
t
|
y
t
-
1
, z
t
-
1
, z
t
-
2
) = 0
(
marks)
This assumption will ensure
Cov
(
u
t
, y
t
-
1
) = 0
,
Cov
(
u
t
, z
t
-
1
) = 0
and
Cov
(
u
t
, z
t
-
2
) = 0
needed for consistency of OLS (can show using LIE), so that the errors and the regressors
(the way they appear in the regression) are uncorrelated.
(
mark)
These assumptions are weaker than strict exogeneity, an assumption that we cannot
have given that we have
y
t
-
1
as regressor anyway. This restriction does permit, e.g.,
Cov
(
u
t
, z
t
+1
)
6
= 0
, so that future regressors may respond to shocks which is important in
time series analysis.
(
mark)
Given the assumptions on the stochastic processes
{
y
t
}
and
{
z
t
}
,
u
t
will be stationary and
weakly dependent automatically.
(c)
Discuss what the short run and long run effect of
z
on
y
are in terms of the parameters and
what they tell you. Under the assumptions given in (b), discuss how you can obtain a con-
sistent estimator of the long run impact. Prove your claim. In your answer you do not have
to prove the consistency of the OLS estimator of
(
β
0
, β
1
, β
2
, ρ
)
.
[
marks]
Solution :
(rescale marks from 6 to
) The short run effect of
z
on
y
here is zero as
z
t
does
not appear as a regressor, that is there is no instantaneous effect of
z
changing
(
marks)
.
©
LSE ST
/EC
R
Page
of
6
To obtain the long run effect (requires
|
ρ
|
<
1
), let us de ne
y
e
and
z
e
as the equilibrium value
so that
y
*
=
β
0
+
ρy
*
+
β
1
z
*
+
β
2
z
*
The error term is taken to be its mean value zero in the long-run. Upon rewriting, we obtain:
y
*
=
β
0
1
-
ρ
+
β
1
+
β
2
1
-
ρ
z
*
The long run effect is
β
1
+
β
2
1
-
ρ
. It shows the effect a permanent unit change in
z
t
has on
y
t
in the
long run (after all lagged effects have been incorporated).
(
marks)
.
To obtain a consistent estimator, we plug in the OLS estimator. Using the properties of the
plim operator, we observe:
plim
ˆ
β
1
+
ˆ
β
2
1
-
ˆ
ρ
!
=
plim
ˆ
β
1
+
plim
ˆ
β
2
1
-
plim
ˆ
ρ
=
β
1
+
β
2
1
-
ρ
establishing its consistency.
(
marks)
.
(d)
You want to test the hypotheses that
u
t
in ( . ) exhibits serial correlation. Discuss how you
can test the hypothesis that there is no autocorrelation against the alternative that
u
t
follows
a stationary, weakly dependent AR( ) process, i.e.,
u
t
=
φu
t
-
1
+
v
t
,
|
φ
|
<
1
( . )
where
v
t
is iid
(
0
, σ
2
v
)
error that is independent of
y
t
-
1
, y
t
-
2
, ..
and
z
s
for all
s.
[
marks]
Solution :
(rescale marks from
to
) You want to test
H
0
:
φ
= 0
H
1
:
φ
6
= 0
To conduct this test, we
rst obtain the OLS residuals:
ˆ
u
t
=
y
t
-
ˆ
β
0
-
ˆ
ρy
t
-
1
ˆ
β
1
z
t
-
1
-
ˆ
β
2
z
t
-
2
Next we estimate the following regression:
ˆ
u
t
=
γ
0
+
ρ
ˆ
u
t
-
1
+
γ
1
y
t
-
1
+
γ
2
z
t
-
1
+
γ
3
z
t
-
2
+
e
t
Under TS. ’-TS. ’, our asymptotic t test is,
t
=
ˆ
ρ
se
(ˆ
ρ
)
a
∼
N
(0
,
1)
under
H
0
(we should assume TS. ’ as well if we do not use white robust standard errors). At the
%
level of signi cance we should reject if the realisation of our test statistic is larger (in abso-
lute value) than
. 6, or equivalently if its p-value is smaller than
%.
(e)
Say your test in (d) does not
nd evidence of autocorrelation. Discuss how you can test whether
β
1
+
β
2
= 0
.
1
against the alternative that
β
1
+
β
2
>
0
.
1
. In your answer discuss what regres-
sion you should estimate if you want to be able to obtain the test statistic directly using your
regression output.
[
marks]
©
LSE ST
/EC
R
Page
of
6
Solution :
(rescale marks from
to
) Under TS. ’-TS. ’ and TS. ’ we can use the asymptotic
t-test:
t
=
ˆ
β
1
+
ˆ
β
2
-
0
.
1
se
(
ˆ
β
1
+
ˆ
β
2
)
a
∼
N
(0
,
1)
under
H
0
.
(we should assume TS. ’ as well if we do not use white robust standard errors). At the
%
level of signi cance we should reject if the realisation of our test statistic is larger than
.6
(one sided).
(
marks)
We can obtain the test statistic directly if we estimate the following regression:
y
t
=
β
0
+
ρy
t
-
1
+
δz
t
-
1
+
β
2
(
z
t
-
2
-
z
t
-
1
) +
u
t
where
δ
=
β
1
+
β
2
. The test therefore becomes
H
0
:
δ
= 0
.
1
against
H
1
:
δ <
0
.
1
. Equally,
we could estimate the regression
y
t
-
.
1
z
t
=
β
0
+
ρy
t
-
1
+
δz
t
-
1
+
β
2
(
z
t
-
2
-
z
t
-
1
) +
u
t
where
δ
=
β
1
+
β
2
-
.
1
. The test therefore becomes
H
0
:
δ
= 0
against
H
1
:
δ <
0
.
(
marks)
(f)
Say your test in (d) detects the presence of autocorrelation. Discuss the following statement:
“In view of the evidence of autocorrelation in ( . ), you will need to make use of HAC robust
standard errors when conducting inference. This will render our test asymptotically valid.” In
your answer, clearly discuss what HAC robust standard errors are and what it means for a test
to be asymptotically valid.
[
marks]
Solution :
(rescale marks from
to
) The problem with this statement is that in the pres-
ence of autocorrelation in the errors OLS on ( . ) no longer provides us with a consistent esti-
mator due to fact that this creates a correlation between
y
t
-
1
and
u
t
, i.e.,
Cov
(
y
t
-
1
, u
t
)
6
= 0
,
a violation of TS. ’. To understand this we observe that both
y
t
-
1
and
u
t
will then depend on
u
t
-
1
and hence they will be correlated. We would not be interested in conducting inference
with such an estimator, as you wouldn’t even be guaranteed to under the null the distribution
of the test will be centered around zero to begin with.
(
marks)
HAC robust standard errors are needed to make tests asymptotically valid in the presence of
autocorrelation (and heteroskedasticity) provided the estimator itself is consistent in the
rst
place. If the above model did not have
y
t
-
1
as regressor, then the presence of autocorrelation
would not necessarily cause a violation of TS. ’. In that case, in order to perform our test, we
would need to adjust the standard errors as the usual standard errors assume TS. ’ (contem-
poraneous homoskedasticity) and TS. ’ (no serial correlation).
(
marks)
(g)
Assume now that
u
t
in ( . ) follows an MA( ) process, i.e.,
u
t
=
v
t
+
θv
t
-
1
,
( . )
where
v
t
is an iid
(
0
, σ
2
v
)
error independent of
y
t
-
1
, y
t
-
2
, ..
;
v
t
is also independent of
z
s
for all
s.
Describe in detail how you would proceed when you want to obtain a consistent estimator
for the parameters
(
β
0
, β
1
, β
2
, ρ
)
.
[
marks]
Solution :
(rescale marks from
to
) Because of the endogeneity of
y
t
-
1
we will need to
propose an IV estimator to estimate the parameters. The instrument(s) we seek need to be:
exogenous (uncorrelated with the error term), relevant (correlated with
y
t
-
1
, and not have a
©
LSE ST
/EC
R
Page
of
6
Your preview ends here
Eager to read complete document? Join bartleby learn and gain access to the full version
- Access to all documents
- Unlimited textbook solutions
- 24/7 expert homework help
direct effect on
y
t
(exclusion). Let us lag ( . ) one period:
y
t
-
1
=
β
0
+
ρy
t
-
2
+
β
1
z
t
-
2
+
β
2
z
t
-
3
+
u
t
-
1
This shows that
z
t
-
3
can be used as an instrument for
y
t
-
1
. In fact, we can also propose to
use
y
t
-
2
here as an instrument as the MA( ) dependence structure ensures that
y
t
-
2
will not
exhibit any correlation with
u
t
(exogeneity).
(
marks)
We can proceed using
SLS (or IV), where
(
marks)
•
Step
: Obtain the
tted values for
y
t
-
1
from running the following regression:
y
t
-
1
=
π
0
+
π
1
z
t
-
1
+
π
2
z
t
-
2
+
π
3
z
t
-
3
+
e
t
The
tted values
ˆ
y
t
-
1
= ˆ
π
0
+ ˆ
π
1
z
t
-
1
+ ˆ
π
2
z
t
-
2
+ ˆ
π
3
z
t
-
3
•
Step
: Run the following regression
y
t
=
β
0
+
ρ
ˆ
y
t
-
1
+
β
1
z
t
-
1
+
β
2
z
t
-
2
+
v
t
END OF PAPER
©
LSE ST
/EC
R
Page
6 of
6
Related Documents
Recommended textbooks for you
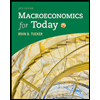
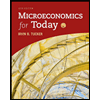
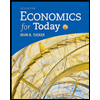
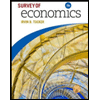
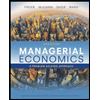
Managerial Economics: A Problem Solving Approach
Economics
ISBN:9781337106665
Author:Luke M. Froeb, Brian T. McCann, Michael R. Ward, Mike Shor
Publisher:Cengage Learning
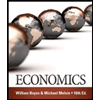
Recommended textbooks for you
- Managerial Economics: A Problem Solving ApproachEconomicsISBN:9781337106665Author:Luke M. Froeb, Brian T. McCann, Michael R. Ward, Mike ShorPublisher:Cengage Learning
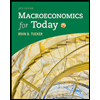
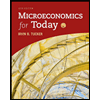
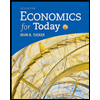
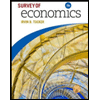
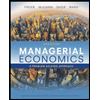
Managerial Economics: A Problem Solving Approach
Economics
ISBN:9781337106665
Author:Luke M. Froeb, Brian T. McCann, Michael R. Ward, Mike Shor
Publisher:Cengage Learning
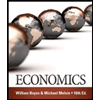