Lab Assignment_2_PartA&B_2024
docx
keyboard_arrow_up
School
Georgia Institute Of Technology *
*We aren’t endorsed by this school
Course
6570
Subject
Civil Engineering
Date
Feb 20, 2024
Type
docx
Pages
8
Uploaded by CountGrouseMaster920
Your preview ends here
Eager to read complete document? Join bartleby learn and gain access to the full version
- Access to all documents
- Unlimited textbook solutions
- 24/7 expert homework help
Your preview ends here
Eager to read complete document? Join bartleby learn and gain access to the full version
- Access to all documents
- Unlimited textbook solutions
- 24/7 expert homework help
Recommended textbooks for you
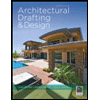
Architectural Drafting and Design (MindTap Course...
Civil Engineering
ISBN:9781285165738
Author:Alan Jefferis, David A. Madsen, David P. Madsen
Publisher:Cengage Learning
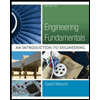
Engineering Fundamentals: An Introduction to Engi...
Civil Engineering
ISBN:9781305084766
Author:Saeed Moaveni
Publisher:Cengage Learning
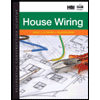
Residential Construction Academy: House Wiring (M...
Civil Engineering
ISBN:9781285852225
Author:Gregory W Fletcher
Publisher:Cengage Learning
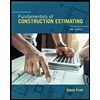
Fundamentals Of Construction Estimating
Civil Engineering
ISBN:9781337399395
Author:Pratt, David J.
Publisher:Cengage,
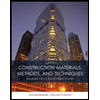
Construction Materials, Methods and Techniques (M...
Civil Engineering
ISBN:9781305086272
Author:William P. Spence, Eva Kultermann
Publisher:Cengage Learning
Recommended textbooks for you
- Architectural Drafting and Design (MindTap Course...Civil EngineeringISBN:9781285165738Author:Alan Jefferis, David A. Madsen, David P. MadsenPublisher:Cengage LearningEngineering Fundamentals: An Introduction to Engi...Civil EngineeringISBN:9781305084766Author:Saeed MoaveniPublisher:Cengage LearningResidential Construction Academy: House Wiring (M...Civil EngineeringISBN:9781285852225Author:Gregory W FletcherPublisher:Cengage Learning
- Fundamentals Of Construction EstimatingCivil EngineeringISBN:9781337399395Author:Pratt, David J.Publisher:Cengage,Construction Materials, Methods and Techniques (M...Civil EngineeringISBN:9781305086272Author:William P. Spence, Eva KultermannPublisher:Cengage Learning
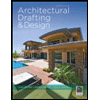
Architectural Drafting and Design (MindTap Course...
Civil Engineering
ISBN:9781285165738
Author:Alan Jefferis, David A. Madsen, David P. Madsen
Publisher:Cengage Learning
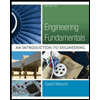
Engineering Fundamentals: An Introduction to Engi...
Civil Engineering
ISBN:9781305084766
Author:Saeed Moaveni
Publisher:Cengage Learning
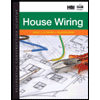
Residential Construction Academy: House Wiring (M...
Civil Engineering
ISBN:9781285852225
Author:Gregory W Fletcher
Publisher:Cengage Learning
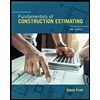
Fundamentals Of Construction Estimating
Civil Engineering
ISBN:9781337399395
Author:Pratt, David J.
Publisher:Cengage,
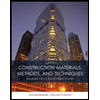
Construction Materials, Methods and Techniques (M...
Civil Engineering
ISBN:9781305086272
Author:William P. Spence, Eva Kultermann
Publisher:Cengage Learning