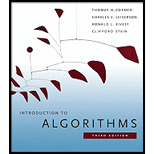
a
To prove the problem of determining the minimum number of bins required is NP-HARD.
a

Explanation of Solution
Suppose and are the same (indeed if the answer is yes for. Then there exists . On the other hand if the answer is yes for , so if
Note that
So, the answer of derived bin-packing problem can-not be less than 2 . First assume that the answer to the given instance of sub set -sum problem is “yes”.
Now, there is
Now
b
To argue that the optimal number of bins required is at least
b

Explanation of Solution
Consider the packing ofn objects with sizes
Let
Therefore,
Since bis an integer number,
c
To argue that the first-fit heuristic leaves at most one bin less than half full.
c

Explanation of Solution
The first- fit heuristic places each object in the first available bin. Suppose by contradiction that two bins
d
To prove that the number of bins used by the first-fit heuristic is never more than
d

Explanation of Solution
Consider a bin- packing solution provided by the first-fit HEURISTIC.
Using similar notation as in (b) (let
It can be assumed without loss of generality that
e
To prove an approximation ratio of 2 for the first-fit heuristic.
e

Explanation of Solution
Letb be the number of the bins used the first-fit HEURISTIC and let
e
To give an efficient implementation of the first-fit heuristic, and analyze its running time.
e

Explanation of Solution
1.
2. initialize data structures
3. fori = 1 to ndo
4. letjbe the first bin that can fit objecti with size
5. ifjexists then
6. inserti at the list (list
7.update data structures
8.else
9.
10. insertiat the list
11.update data structures II
12.endif
13. endfor
Each of the above steps can be done in
Want to see more full solutions like this?
Chapter 35 Solutions
Introduction to Algorithms
- Can you please solve this. Thanksarrow_forwardcan you solve this pleasearrow_forwardIn the previous homework scenario problem below: You have been hired by TechCo to create and manage their employee training portal. Your first task is to develop a program that will create and track different training sessions in the portal. Each training session has the following properties: • A session ID (e.g., "TECH101", "TECH205") • A session title (e.g., "Machine learning", "Advanced Java Programming") • A total duration in hours (e.g., 5.0, 8.0) • Current number of participants (e.g., 25) Each session must have at least a session ID and a total duration and must met the following requirements: • The maximum participant for each session is 30. • The total duration of a session must not exceed 10 hours. • The current number of participants should never exceed the maximum number of participants. Design an object-oriented solution to create a data definition class(DDC) and an implementation class for the session object. In the DDC, a session class must include: • Constructors to…arrow_forward
- In the previous homework scenario problem below: You have been hired by TechCo to create and manage their employee training portal. Your first task is to develop a program that will create and track different training sessions in the portal. Each training session has the following properties: • A session ID (e.g., "TECH101", "TECH205") • A session title (e.g., "Machine learning", "Advanced Java Programming") • A total duration in hours (e.g., 5.0, 8.0) • Current number of participants (e.g., 25) Each session must have at least a session ID and a total duration and must met the following requirements: • The maximum participant for each session is 30. • The total duration of a session must not exceed 10 hours. • The current number of participants should never exceed the maximum number of participants. Design an object-oriented solution to create a data definition class(DDC) and an implementation class for the session object. In the DDC, a session class must include: • Constructors to…arrow_forwardSend me the lexer and parserarrow_forwardHere is my code please draw a transition diagram and nfa on paper public class Lexer { private static final char EOF = 0; private static final int BUFFER_SIZE = 10; private Parser yyparser; // parent parser object private java.io.Reader reader; // input stream public int lineno; // line number public int column; // column // Double buffering implementation private char[] buffer1; private char[] buffer2; private boolean usingBuffer1; private int currentPos; private int bufferLength; private boolean endReached; // Keywords private static final String[] keywords = { "int", "print", "if", "else", "while", "void" }; public Lexer(java.io.Reader reader, Parser yyparser) throws Exception { this.reader = reader; this.yyparser = yyparser; this.lineno = 1; this.column = 0; // Initialize double buffering buffer1 = new char[BUFFER_SIZE]; buffer2 = new char[BUFFER_SIZE]; usingBuffer1 = true; currentPos = 0; bufferLength = 0; endReached = false; // Initial buffer fill fillBuffer(); } private…arrow_forward
- Create 2 charts using this data. One without using wind speed and one including max speed in mph. Write a Report and a short report explaining your visualizations and design decisions. Include the following: Lead Story: Identify the key story or insight based on your visualizations. Shaffer’s 4C Framework: Describe how you applied Shaffer’s 4C principles in the design of your charts. External Data Integration: Explain the second data and how you integrated it with the Halloween dataset. Compare the two datasets. Attach screenshots of the two charts (Bar graph or Line graph) The Shaffer 4 C’s of Data Visualization Clear - easily seen; sharply defined• who's the audience? what's the message? clarity more important than aestheticsClean - thorough; complete; unadulterated, labels, axis, gridlines, formatting, right chart type, colorchoice, etc.Concise - brief but comprehensive. not minimalist but not verboseCaptivating - to attract and hold by beauty or excellence does it capture…arrow_forwardHow can I resolve the following issue?arrow_forwardI need help to resolve, thank you.arrow_forward
- Operations Research : Applications and AlgorithmsComputer ScienceISBN:9780534380588Author:Wayne L. WinstonPublisher:Brooks ColeC++ Programming: From Problem Analysis to Program...Computer ScienceISBN:9781337102087Author:D. S. MalikPublisher:Cengage LearningC++ for Engineers and ScientistsComputer ScienceISBN:9781133187844Author:Bronson, Gary J.Publisher:Course Technology Ptr
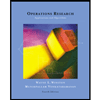
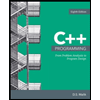
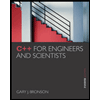