(a)
To explain:
The
(a)

Explanation of Solution
Given:
The table of the exposure and change.
Concept used:
Formula
The binomial distribution
Calculation:
Scatter plot is given as the table
The weak is negative relationship exists.
Because the value of
Where
Let us perform the regression
Regressing sub concussion exposure on white matter change with below mentioned steps in excel
SUMMARY OUTPUT
Regression Statistics
Multiple R
R Square
Adjusted R Square
Standard Error
Observations
Draw the table
Degree | SS | MS | F | Significant | |
Regression | |||||
Residual | |||||
Total |
Draw the second table
Coefficient | Standard Error | t Stat | P-value | Lower | Upper | Lower | Upper | |
Intercept | ||||||||
(b)
To find:
Theregression standard error of the given data.
(b)

Explanation of Solution
Given:
The table of the exposure and change.
Concept used:
Formula
The binomial distribution
Calculation:
Scatter plot is given as the table
The weak is negative relationship exists.
Because the value of
Where
Let us perform the regression
Regressing sub concussion exposure on white matter change with below mentioned steps in excel
SUMMARY OUTPUT
Regression Statistics
Multiple R
R Square
Adjusted R Square
Standard Error
Observations
Draw the table
Degree | SS | MS | F | Significant | |
Regression | |||||
Residual | |||||
Total |
Draw the second table
Coefficient | Standard Error | t Stat | P-value | Lower | Upper | Lower | Upper | |
Intercept | ||||||||
Here
So least squares regression line
Change (
Regression standard error
The low p-value of
Hence, the null hypothesis of no relationship between exposure and white matter change can be rejected in favor of the alternative hypothesis at a confidence level of
(c)
To explain:
Theequation for the least-squares regression line without data point and test null hypothesis of the given data.
(c)

Explanation of Solution
Given:
The table of the exposure and change.
Concept used:
Formula
The binomial distribution
Calculation:
Scatter plot is given as the table
The weak is negative relationship exists.
Because the value of
Where
Let us perform the regression
Regressing sub concussion exposure on white matter change with below mentioned steps in excel
SUMMARY OUTPUT
Regression Statistics
Multiple R
R Square
Adjusted R Square
Standard Error
Observations
Draw the table
Degree | SS | MS | F | Significant | |
Regression | |||||
Residual | |||||
Total |
Draw the second table
Coefficient | Standard Error | t Stat | P-value | Lower | Upper | Lower | Upper | |
Intercept | ||||||||
Here
So least squares regression line
Change (
Regression standard error
The low p-value of
Hence, the null hypothesis of no relationship between exposure and white matter change can be rejected in favor of the alternative hypothesis at a confidence level of
SUMMARY OUTPUT
Regression Statistics
Multiple R
R Square
Adjusted R Square
Standard Error
Observations
Draw the table
Degree | SS | MS | F | Significant | |
Regression | |||||
Residual | |||||
Total |
Draw the second table
Coefficient | Standard Error | t Stat | P-value | Lower | Upper | Lower | Upper | |
Intercept | ||||||||
Standard error is same
But the p value is
Exposure is not good predictor of Change in white matter.
Also, the Exposure coefficient has come down from
(d)
To explain:
Theimpact of the usual observation on the
(d)

Explanation of Solution
Given:
The table of the exposure and change.
Concept used:
Formula
The binomial distribution
Calculation:
Scatter plot is given as the table
The weak is negative relationship exists.
Because the value of
Where
Let us perform the regression
Regressing sub concussion exposure on white matter change with below mentioned steps in excel
SUMMARY OUTPUT
Regression Statistics
Multiple R
R Square
Adjusted R Square
Standard Error
Observations
Draw the table
Degree | SS | MS | F | Significant | |
Regression | |||||
Residual | |||||
Total |
Draw the second table
Coefficient | Standard Error | t Stat | P-value | Lower | Upper | Lower | Upper | |
Intercept | ||||||||
Here
So least squares regression line
Change (
Regression standard error
The low p-value of
Hence, the null hypothesis of no relationship between exposure and white matter change can be rejected in favor of the alternative hypothesis at a confidence level of
SUMMARY OUTPUT
Regression Statistics
Multiple R
R Square
Adjusted R Square
Standard Error
Observations
Draw the table
Degree | SS | MS | F | Significant | |
Regression | |||||
Residual | |||||
Total |
Draw the second table
Coefficient | Standard Error | t Stat | P-value | Lower | Upper | Lower | Upper | |
Intercept | ||||||||
Standard error is same
But the p value is
Exposure is not good predictor of Change in white matter.
Also, the Exposure coefficient has come down from
The Change in white matter will not actually change as fast
So,eliminate of the outlier data points.
The Outlier data points tend to increase the correlation between the two variables.
Want to see more full solutions like this?
Chapter 23 Solutions
ACHIEVE F/PRACT OF STAT IN LIFE-ACCESS
- A television news channel samples 25 gas stations from its local area and uses the results to estimate the average gas price for the state. What’s wrong with its margin of error?arrow_forwardYou’re fed up with keeping Fido locked inside, so you conduct a mail survey to find out people’s opinions on the new dog barking ordinance in a certain city. Of the 10,000 people who receive surveys, 1,000 respond, and only 80 are in favor of it. You calculate the margin of error to be 1.2 percent. Explain why this reported margin of error is misleading.arrow_forwardYou find out that the dietary scale you use each day is off by a factor of 2 ounces (over — at least that’s what you say!). The margin of error for your scale was plus or minus 0.5 ounces before you found this out. What’s the margin of error now?arrow_forward
- Suppose that Sue and Bill each make a confidence interval out of the same data set, but Sue wants a confidence level of 80 percent compared to Bill’s 90 percent. How do their margins of error compare?arrow_forwardSuppose that you conduct a study twice, and the second time you use four times as many people as you did the first time. How does the change affect your margin of error? (Assume the other components remain constant.)arrow_forwardOut of a sample of 200 babysitters, 70 percent are girls, and 30 percent are guys. What’s the margin of error for the percentage of female babysitters? Assume 95 percent confidence.What’s the margin of error for the percentage of male babysitters? Assume 95 percent confidence.arrow_forward
- You sample 100 fish in Pond A at the fish hatchery and find that they average 5.5 inches with a standard deviation of 1 inch. Your sample of 100 fish from Pond B has the same mean, but the standard deviation is 2 inches. How do the margins of error compare? (Assume the confidence levels are the same.)arrow_forwardA survey of 1,000 dental patients produces 450 people who floss their teeth adequately. What’s the margin of error for this result? Assume 90 percent confidence.arrow_forwardThe annual aggregate claim amount of an insurer follows a compound Poisson distribution with parameter 1,000. Individual claim amounts follow a Gamma distribution with shape parameter a = 750 and rate parameter λ = 0.25. 1. Generate 20,000 simulated aggregate claim values for the insurer, using a random number generator seed of 955.Display the first five simulated claim values in your answer script using the R function head(). 2. Plot the empirical density function of the simulated aggregate claim values from Question 1, setting the x-axis range from 2,600,000 to 3,300,000 and the y-axis range from 0 to 0.0000045. 3. Suggest a suitable distribution, including its parameters, that approximates the simulated aggregate claim values from Question 1. 4. Generate 20,000 values from your suggested distribution in Question 3 using a random number generator seed of 955. Use the R function head() to display the first five generated values in your answer script. 5. Plot the empirical density…arrow_forward
- Find binomial probability if: x = 8, n = 10, p = 0.7 x= 3, n=5, p = 0.3 x = 4, n=7, p = 0.6 Quality Control: A factory produces light bulbs with a 2% defect rate. If a random sample of 20 bulbs is tested, what is the probability that exactly 2 bulbs are defective? (hint: p=2% or 0.02; x =2, n=20; use the same logic for the following problems) Marketing Campaign: A marketing company sends out 1,000 promotional emails. The probability of any email being opened is 0.15. What is the probability that exactly 150 emails will be opened? (hint: total emails or n=1000, x =150) Customer Satisfaction: A survey shows that 70% of customers are satisfied with a new product. Out of 10 randomly selected customers, what is the probability that at least 8 are satisfied? (hint: One of the keyword in this question is “at least 8”, it is not “exactly 8”, the correct formula for this should be = 1- (binom.dist(7, 10, 0.7, TRUE)). The part in the princess will give you the probability of seven and less than…arrow_forwardplease answer these questionsarrow_forwardSelon une économiste d’une société financière, les dépenses moyennes pour « meubles et appareils de maison » ont été moins importantes pour les ménages de la région de Montréal, que celles de la région de Québec. Un échantillon aléatoire de 14 ménages pour la région de Montréal et de 16 ménages pour la région Québec est tiré et donne les données suivantes, en ce qui a trait aux dépenses pour ce secteur d’activité économique. On suppose que les données de chaque population sont distribuées selon une loi normale. Nous sommes intéressé à connaitre si les variances des populations sont égales.a) Faites le test d’hypothèse sur deux variances approprié au seuil de signification de 1 %. Inclure les informations suivantes : i. Hypothèse / Identification des populationsii. Valeur(s) critique(s) de Fiii. Règle de décisioniv. Valeur du rapport Fv. Décision et conclusion b) A partir des résultats obtenus en a), est-ce que l’hypothèse d’égalité des variances pour cette…arrow_forward
- MATLAB: An Introduction with ApplicationsStatisticsISBN:9781119256830Author:Amos GilatPublisher:John Wiley & Sons IncProbability and Statistics for Engineering and th...StatisticsISBN:9781305251809Author:Jay L. DevorePublisher:Cengage LearningStatistics for The Behavioral Sciences (MindTap C...StatisticsISBN:9781305504912Author:Frederick J Gravetter, Larry B. WallnauPublisher:Cengage Learning
- Elementary Statistics: Picturing the World (7th E...StatisticsISBN:9780134683416Author:Ron Larson, Betsy FarberPublisher:PEARSONThe Basic Practice of StatisticsStatisticsISBN:9781319042578Author:David S. Moore, William I. Notz, Michael A. FlignerPublisher:W. H. FreemanIntroduction to the Practice of StatisticsStatisticsISBN:9781319013387Author:David S. Moore, George P. McCabe, Bruce A. CraigPublisher:W. H. Freeman
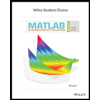
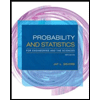
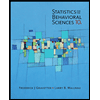
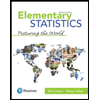
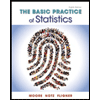
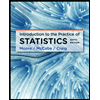