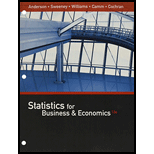
Bundle: Statistics for Business & Economics, Loose-Leaf Version, 13th + MindTap Business Statistics with XLSTAT, 1 term (6 months) Printed Access Card
13th Edition
ISBN: 9781337148092
Author: David R. Anderson, Dennis J. Sweeney, Thomas A. Williams, Jeffrey D. Camm, James J. Cochran
Publisher: Cengage Learning
expand_more
expand_more
format_list_bulleted
Concept explainers
Textbook Question
Chapter 14.6, Problem 39E
In exercise 12, the following data on x = average daily hotel room rate and y = amount spent on entertainment (The Wall street Journal, August 18, 2011) lead to the estimated regression equation ŷ = 17.49 + 1.0334x. For these data SSE = 1541.4.
City | Room Rate ($) |
Entertainment ($) |
Boston | 148 | 161 |
Denver | 96 | 105 |
Nashville | 91 | 101 |
New Orleans | 110 | 142 |
Phoenix | 90 | 100 |
San Diego | 102 | 120 |
San Francisco | 136 | 167 |
San Jose | 90 | 140 |
Tampa | 82 | 98 |
- a. Predict the amount spent on entertainment for a particular city that has a daily room rate of $89.
- b. Develop a 95% confidence interval for the
mean amount spent on entertainment for all cities that have a daily room rate of $89. - c. The average room rate in Chicago is $128. Develop a 95% prediction interval for the amount spent on entertainment in Chicago.
Expert Solution & Answer

Trending nowThis is a popular solution!

Students have asked these similar questions
The following ordered data list shows the data speeds for cell phones used by a
telephone company at an airport:
A. Calculate the Measures of Central Tendency from the ungrouped data list.
B. Group the data in an appropriate frequency table.
C. Calculate the Measures of Central Tendency using the table in point B.
0.8
1.4
1.8
1.9
3.2
3.6
4.5
4.5
4.6
6.2
6.5
7.7
7.9
9.9
10.2
10.3
10.9
11.1
11.1
11.6
11.8
12.0
13.1
13.5
13.7
14.1
14.2
14.7
15.0
15.1
15.5
15.8
16.0
17.5
18.2
20.2
21.1
21.5
22.2
22.4
23.1
24.5
25.7
28.5
34.6
38.5
43.0
55.6
71.3
77.8
II Consider the following data matrix X:
X1
X2
0.5
0.4
0.2 0.5
0.5
0.5
10.3 10
10.1 10.4
10.1
10.5
What will the resulting clusters be when using the k-Means method with k = 2. In your own words, explain why this result is
indeed expected, i.e. why this clustering minimises the ESS map.
why the answer is 3 and 10?
Chapter 14 Solutions
Bundle: Statistics for Business & Economics, Loose-Leaf Version, 13th + MindTap Business Statistics with XLSTAT, 1 term (6 months) Printed Access Card
Ch. 14.2 - Given are five observations for two variables, x...Ch. 14.2 - Given are five observations for two variables, x...Ch. 14.2 - Given are five observations collected in a...Ch. 14.2 - The following data give the percentage of women...Ch. 14.2 - Brawdy Plastics, Inc., produces plastic seat belt...Ch. 14.2 - The National Football League (NFL) records a...Ch. 14.2 - A sales manager collected the following data on...Ch. 14.2 - The American Association of Individual Investors...Ch. 14.2 - Prob. 9ECh. 14.2 - On March 31, 2009, Ford Motor Companys shares were...
Ch. 14.2 - To help consumers in purchasing a laptop computer,...Ch. 14.2 - Concur Technologies, Inc., is a large...Ch. 14.2 - To the Internal Revenue Service, the...Ch. 14.2 - A large city hospital conducted a study to...Ch. 14.3 - The data from exercise 1 follow. xi 1 2 3 4 5 yi 3...Ch. 14.3 - The data from exercise 2 follow. xi 3 12 6 20 14...Ch. 14.3 - The data from exercise 3 follow. xi 2 6 9 13 20 yi...Ch. 14.3 - The following data show the brand, price (), and...Ch. 14.3 - In exercise 7 a sales manager collected the...Ch. 14.3 - Bicycling, the worlds leading cycling magazine,...Ch. 14.3 - An important application of regression analysis in...Ch. 14.3 - Refer to exercise 9, where the following data were...Ch. 14.5 - The data from exercise 1 follow. xi 1 2 3 4 5 yi 3...Ch. 14.5 - The data from exercise 2 follow. xi 3 12 6 20 14...Ch. 14.5 - The data from exercise 3 follow. xi 2 6 9 13 20 yi...Ch. 14.5 - In exercise 18 the data on price () and the...Ch. 14.5 - To identify high-paying jobs for people who do not...Ch. 14.5 - In exercise 8 ratings data on x = the quality of...Ch. 14.5 - Refer to exercise 21, where data on production...Ch. 14.5 - Refer to exercise 9, where the following data were...Ch. 14.5 - In exercise 20, data on x = weight (pounds) and y...Ch. 14.6 - The data from exercise 1 follow. xi 1 2 3 4 5 yi 3...Ch. 14.6 - The data from exercise 2 follow. xi 3 12 6 20 14...Ch. 14.6 - The data from exercise 3 follow. xi 2 6 9 13 20 yi...Ch. 14.6 - The following data are the monthly salaries y and...Ch. 14.6 - In exercise 7, the data on y = annual sales (...Ch. 14.6 - In exercise 13, data were given on the adjusted...Ch. 14.6 - Refer to exercise 21, where data on the production...Ch. 14.6 - In exercise 12, the following data on x = average...Ch. 14.7 - The commercial division of a real estate firm is...Ch. 14.7 - Following is a portion of the computer output for...Ch. 14.7 - A regression model relating x, number of...Ch. 14.7 - A 2012 suvey conducted by Idea Works provided data...Ch. 14.7 - Automobile racing, high-performance driving...Ch. 14.8 - Given are data for two variables, x and y. xi 6 11...Ch. 14.8 - The following data were used in a regression...Ch. 14.8 - Data on advertising expenditures and revenue (in...Ch. 14.8 - Refer to exercise 7, where an estimated regression...Ch. 14.8 - In 2011 home prices and mortgage rates dropped so...Ch. 14.9 - Consider the following data for two variables, x...Ch. 14.9 - Consider the following data for two variables, x...Ch. 14.9 - Charity Navigator is Americas leading independent...Ch. 14.9 - Many countries, especially those in Europe, have...Ch. 14.9 - Prob. 54ECh. 14 - Does a high value of r2 imply that two variables...Ch. 14 - In your own words, explain the difference between...Ch. 14 - What is the purpose of testing whether 1 = 0? If...Ch. 14 - The Dow Jones Industrial Average (DJIA) and the...Ch. 14 - Is the number of square feet of living space a...Ch. 14 - One of the biggest changes in higher education in...Ch. 14 - Jensen Tire Auto is in the process of deciding...Ch. 14 - In a manufacturing process the assembly line speed...Ch. 14 - A sociologist was hired by a large city hospital...Ch. 14 - The regional transit authority for a major...Ch. 14 - A marketing professor at Givens College is...Ch. 14 - The Transactional Records Access Clearinghouse at...Ch. 14 - The Toyota Camry is one of the best-selling cars...Ch. 14 - You have been assigned to analyze the risk...Ch. 14 - As part of a study on transportation safety, the...Ch. 14 - Consumer Reports tested 166 different...Ch. 14 - Finding the Best Car Value When trying to decide...Ch. 14 - Buckeye Creek Amusement Park is open from the...
Knowledge Booster
Learn more about
Need a deep-dive on the concept behind this application? Look no further. Learn more about this topic, statistics and related others by exploring similar questions and additional content below.Similar questions
- PS 9 Two films are shown on screen A and screen B at a cinema each evening. The numbers of people viewing the films on 12 consecutive evenings are shown in the back-to-back stem-and-leaf diagram. Screen A (12) Screen B (12) 8 037 34 7 6 4 0 534 74 1645678 92 71689 Key: 116|4 represents 61 viewers for A and 64 viewers for B A second stem-and-leaf diagram (with rows of the same width as the previous diagram) is drawn showing the total number of people viewing films at the cinema on each of these 12 evenings. Find the least and greatest possible number of rows that this second diagram could have. TIP On the evening when 30 people viewed films on screen A, there could have been as few as 37 or as many as 79 people viewing films on screen B.arrow_forwardQ.2.4 There are twelve (12) teams participating in a pub quiz. What is the probability of correctly predicting the top three teams at the end of the competition, in the correct order? Give your final answer as a fraction in its simplest form.arrow_forwardThe table below indicates the number of years of experience of a sample of employees who work on a particular production line and the corresponding number of units of a good that each employee produced last month. Years of Experience (x) Number of Goods (y) 11 63 5 57 1 48 4 54 5 45 3 51 Q.1.1 By completing the table below and then applying the relevant formulae, determine the line of best fit for this bivariate data set. Do NOT change the units for the variables. X y X2 xy Ex= Ey= EX2 EXY= Q.1.2 Estimate the number of units of the good that would have been produced last month by an employee with 8 years of experience. Q.1.3 Using your calculator, determine the coefficient of correlation for the data set. Interpret your answer. Q.1.4 Compute the coefficient of determination for the data set. Interpret your answer.arrow_forward
- Can you answer this question for mearrow_forwardTechniques QUAT6221 2025 PT B... TM Tabudi Maphoru Activities Assessments Class Progress lIE Library • Help v The table below shows the prices (R) and quantities (kg) of rice, meat and potatoes items bought during 2013 and 2014: 2013 2014 P1Qo PoQo Q1Po P1Q1 Price Ро Quantity Qo Price P1 Quantity Q1 Rice 7 80 6 70 480 560 490 420 Meat 30 50 35 60 1 750 1 500 1 800 2 100 Potatoes 3 100 3 100 300 300 300 300 TOTAL 40 230 44 230 2 530 2 360 2 590 2 820 Instructions: 1 Corall dawn to tha bottom of thir ceraan urina se se tha haca nariad in archerca antarand cubmit Q Search ENG US 口X 2025/05arrow_forwardThe table below indicates the number of years of experience of a sample of employees who work on a particular production line and the corresponding number of units of a good that each employee produced last month. Years of Experience (x) Number of Goods (y) 11 63 5 57 1 48 4 54 45 3 51 Q.1.1 By completing the table below and then applying the relevant formulae, determine the line of best fit for this bivariate data set. Do NOT change the units for the variables. X y X2 xy Ex= Ey= EX2 EXY= Q.1.2 Estimate the number of units of the good that would have been produced last month by an employee with 8 years of experience. Q.1.3 Using your calculator, determine the coefficient of correlation for the data set. Interpret your answer. Q.1.4 Compute the coefficient of determination for the data set. Interpret your answer.arrow_forward
- Q.3.2 A sample of consumers was asked to name their favourite fruit. The results regarding the popularity of the different fruits are given in the following table. Type of Fruit Number of Consumers Banana 25 Apple 20 Orange 5 TOTAL 50 Draw a bar chart to graphically illustrate the results given in the table.arrow_forwardQ.2.3 The probability that a randomly selected employee of Company Z is female is 0.75. The probability that an employee of the same company works in the Production department, given that the employee is female, is 0.25. What is the probability that a randomly selected employee of the company will be female and will work in the Production department? Q.2.4 There are twelve (12) teams participating in a pub quiz. What is the probability of correctly predicting the top three teams at the end of the competition, in the correct order? Give your final answer as a fraction in its simplest form.arrow_forwardQ.2.1 A bag contains 13 red and 9 green marbles. You are asked to select two (2) marbles from the bag. The first marble selected will not be placed back into the bag. Q.2.1.1 Construct a probability tree to indicate the various possible outcomes and their probabilities (as fractions). Q.2.1.2 What is the probability that the two selected marbles will be the same colour? Q.2.2 The following contingency table gives the results of a sample survey of South African male and female respondents with regard to their preferred brand of sports watch: PREFERRED BRAND OF SPORTS WATCH Samsung Apple Garmin TOTAL No. of Females 30 100 40 170 No. of Males 75 125 80 280 TOTAL 105 225 120 450 Q.2.2.1 What is the probability of randomly selecting a respondent from the sample who prefers Garmin? Q.2.2.2 What is the probability of randomly selecting a respondent from the sample who is not female? Q.2.2.3 What is the probability of randomly…arrow_forward
- Test the claim that a student's pulse rate is different when taking a quiz than attending a regular class. The mean pulse rate difference is 2.7 with 10 students. Use a significance level of 0.005. Pulse rate difference(Quiz - Lecture) 2 -1 5 -8 1 20 15 -4 9 -12arrow_forwardThe following ordered data list shows the data speeds for cell phones used by a telephone company at an airport: A. Calculate the Measures of Central Tendency from the ungrouped data list. B. Group the data in an appropriate frequency table. C. Calculate the Measures of Central Tendency using the table in point B. D. Are there differences in the measurements obtained in A and C? Why (give at least one justified reason)? I leave the answers to A and B to resolve the remaining two. 0.8 1.4 1.8 1.9 3.2 3.6 4.5 4.5 4.6 6.2 6.5 7.7 7.9 9.9 10.2 10.3 10.9 11.1 11.1 11.6 11.8 12.0 13.1 13.5 13.7 14.1 14.2 14.7 15.0 15.1 15.5 15.8 16.0 17.5 18.2 20.2 21.1 21.5 22.2 22.4 23.1 24.5 25.7 28.5 34.6 38.5 43.0 55.6 71.3 77.8 A. Measures of Central Tendency We are to calculate: Mean, Median, Mode The data (already ordered) is: 0.8, 1.4, 1.8, 1.9, 3.2, 3.6, 4.5, 4.5, 4.6, 6.2, 6.5, 7.7, 7.9, 9.9, 10.2, 10.3, 10.9, 11.1, 11.1, 11.6, 11.8, 12.0, 13.1, 13.5, 13.7, 14.1, 14.2, 14.7, 15.0, 15.1, 15.5,…arrow_forwardPEER REPLY 1: Choose a classmate's Main Post. 1. Indicate a range of values for the independent variable (x) that is reasonable based on the data provided. 2. Explain what the predicted range of dependent values should be based on the range of independent values.arrow_forward
arrow_back_ios
SEE MORE QUESTIONS
arrow_forward_ios
Recommended textbooks for you
- Functions and Change: A Modeling Approach to Coll...AlgebraISBN:9781337111348Author:Bruce Crauder, Benny Evans, Alan NoellPublisher:Cengage LearningBig Ideas Math A Bridge To Success Algebra 1: Stu...AlgebraISBN:9781680331141Author:HOUGHTON MIFFLIN HARCOURTPublisher:Houghton Mifflin Harcourt
- Glencoe Algebra 1, Student Edition, 9780079039897...AlgebraISBN:9780079039897Author:CarterPublisher:McGraw HillAlgebra and Trigonometry (MindTap Course List)AlgebraISBN:9781305071742Author:James Stewart, Lothar Redlin, Saleem WatsonPublisher:Cengage LearningCollege AlgebraAlgebraISBN:9781305115545Author:James Stewart, Lothar Redlin, Saleem WatsonPublisher:Cengage Learning
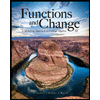
Functions and Change: A Modeling Approach to Coll...
Algebra
ISBN:9781337111348
Author:Bruce Crauder, Benny Evans, Alan Noell
Publisher:Cengage Learning
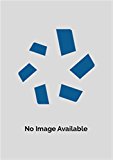
Big Ideas Math A Bridge To Success Algebra 1: Stu...
Algebra
ISBN:9781680331141
Author:HOUGHTON MIFFLIN HARCOURT
Publisher:Houghton Mifflin Harcourt
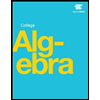
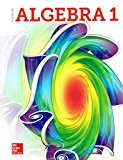
Glencoe Algebra 1, Student Edition, 9780079039897...
Algebra
ISBN:9780079039897
Author:Carter
Publisher:McGraw Hill
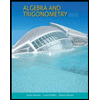
Algebra and Trigonometry (MindTap Course List)
Algebra
ISBN:9781305071742
Author:James Stewart, Lothar Redlin, Saleem Watson
Publisher:Cengage Learning
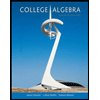
College Algebra
Algebra
ISBN:9781305115545
Author:James Stewart, Lothar Redlin, Saleem Watson
Publisher:Cengage Learning
Correlation Vs Regression: Difference Between them with definition & Comparison Chart; Author: Key Differences;https://www.youtube.com/watch?v=Ou2QGSJVd0U;License: Standard YouTube License, CC-BY
Correlation and Regression: Concepts with Illustrative examples; Author: LEARN & APPLY : Lean and Six Sigma;https://www.youtube.com/watch?v=xTpHD5WLuoA;License: Standard YouTube License, CC-BY