Concept explainers
a.
Identify and explain whichof the given modelscan be recommended.
a.

Answer to Problem 66SE
The model with 2 predictors and the model with 3 predictors can be recommended for predicting the pH before addition of dyes.
Explanation of Solution
Given info:
The MINITAB output shows the best regression option for the data predicted for pH before the addition of dyes using carpet density, carpet weight, dye weight, dye weight as a percentage of carpet and pH after addition of dyes.
Justification:
Mallows
It is used to assess the fit of regression model where the aim to find the best subset of predictors. A relatively small value of
By observing the mallows
By examining the models with three variables,
Hence, the model with two predictorsnamely dye weight and pH after addition of dyes could be considered as a best model subset for predicting pH before the addition of dyes.
Also, a second option would be the model with three predictorsnamely carpet weight, dye weight and pH after addition of dyes could be considered as a best model subset for predicting pH before the addition of dyes.
b.
Test whether the model suggests a useful linear relationship between pH before the addition of dyes and at least one of the predictors.
b.

Answer to Problem 66SE
There is sufficient evidence to conclude that the there is a use of linear relationship between pH before the addition of dyes and at least one of the predictors dye weight and pH after the addition of dyes.
Explanation of Solution
Given info:
The MINITAB output for predicting the pH before the addition of dyes using the dye weight
Calculation:
The test hypotheses are given below:
Null hypothesis:
That is, there is no use of linear relationship between pH before the addition of dyes and the predictors dye weightand pH after the addition of dyes.
Alternative hypothesis:
That is, there is a use of linear relationship between pH before the addition of dyes and at least one of the predictors dye weightand pH after the addition of dyes.
Conclusion:
The P-value is 0.000 and the level of significance is 0.001.
The P-value is lesser than the level of significance.
That is
Thus, the null hypothesis is rejected.
Hence, there is sufficient evidence to conclude that there is a use of linear relationship between pH before the addition of dyes and at least one of the predictors dye weight and pH after the addition of dyes.
c.
Explain whether either one of the predictors could be eliminated from the model given that the other predictor is retained.
c.

Answer to Problem 66SE
No, either one of the predictors could not be eliminated from the model given that the other predictor is retained.
Explanation of Solution
Calculation:
For variable
Testing the hypothesis:
Null hypothesis:
That is, there is no use of linear relationship between pH before the addition of dyes and dye weightgiven that pH after addition of dyes was retained in the model.
Alternative hypothesis:
That is, there is a use of linear relationship between pH before the addition of dyes and dye weightgiven that pH after addition of dyes was retained in the model.
From the MINITAB output it can be observed that the P-value corresponding to the t statistic of
Conclusion:
The P-value is 0.000 and the level of significance is 0.001.
The P-value is lesser than the level of significance.
That is
Thus, the null hypothesis is rejected.
Hence, there is sufficient evidence to conclude that there is a use of linear relationship between pH before the addition of dyes and dye weight given that pH after addition of dyes was retained in the model.
For variable
Testing the hypothesis:
Null hypothesis:
That is, there is no use of linear relationship between pH before the addition of dyes and pH after addition of dyes given that dye weight was retained in the model.
Alternative hypothesis:
That is, there is a use of linear relationship between pH before the addition of dyes and pH after addition of dyes given that dye weight was retained in the model.
From the MINITAB output it can be observed that the P-value corresponding to the t statistic of
Conclusion:
The P-value is 0.000 and the level of significance is 0.001.
The P-value is lesser than the level of significance.
That is
Thus, the null hypothesis is rejected.
Hence, there is sufficient evidence to conclude that there is a use of linear relationship between pH before the addition of dyes and pH after addition of dyes given that dye weight was retained in the model.
Justification:
From the analysis it can be concluded that none of the variables can be eliminated from the model given that the other variable is already present in the model.
d.
Calculate and interpret the 95% confidence interval for the two predictors.
d.

Answer to Problem 66SE
The 95% confidence interval for the estimated slope coefficient
(–0.0000684, –0.0000244).
The 95% confidence interval for the estimated slope coefficient
Explanation of Solution
Calculation:
The 95% confidence interval is calculated using the formula:
The confidence interval is calculated using the formula:
Where,
n is the total number of observations.
k is the total number of predictors in the model.
Critical value:
Software procedure:
Step-by-step procedure to find the critical value is given below:
- Click on Graph, select View Probability and click OK.
- Select t, enter 111 as Degrees of freedom, inShaded Area Tab select Probability under Define Shaded Area By and choose Both tails.
- Enter Probability value as 0.05.
- Click OK.
Output obtained from MINITAB is given below:
The 95% confidence interval for
Thus, the 95% confidence interval for the estimated slope coefficient
(–0.0000684, –0.0000244).
The 95% confidence interval for
Thus, the 95% confidence interval for the estimated slope coefficient
(0.6417,0.8325).
Interpretation:
For the variable
For one unit increase in the dye weight, it is 95% confident that the estimated value of pH before addition of dyes would decrease between–0.00000684 and–0.0000244 given that pH after addition of dyes is fixed constant.
For the variable
For one unit increase in the pH after the addition of dyes it is 95% confident that the estimated value of pH before addition of dyes would increase between 0.6417 and 0.8325 given that dye weight is fixed constant.
e.
Calculate and interpret the 95% confidence interval for the average value of pH before the addition of dyes when the dye weight and pH after the addition of dyes takes 1,000 and 6, respectively.
e.

Answer to Problem 66SE
The 95% confidence interval for the average value of pH before the addition of dyes when the dye weight and pH after the addition of dyes takes 1,000 and 6, respectively is (5.250, 5.383)
Explanation of Solution
Given info:
The estimated standard deviation for predicting the pH before the addition of dyes when the dye weight and pH after the addition of dyes takes 1,000 and 6 is 0.0336.
Calculation:
The average value of pH before the addition of dyes when the dye weight and pH after the addition of dyes takes 1,000 and 6 is calculated as follows:
Thus, the average value of pH before the addition of dyes when the dye weight and pH after the addition of dyes takes 1,000 and 6 is 5.316.
95% confidence interval for the true response:
The confidence interval is calculated using the formula:
Where,
n is the total number of observations.
k is the total number of predictors in the model.
Critical value:
Software procedure:
Step-by-step procedure to find the critical value is given below:
- Click on Graph, select View Probability and click OK.
- Select t, enter 111 as Degrees of freedom, in Shaded Area Tab select Probability under Define Shaded Area By and choose Both tails.
- Enter Probability value as 0.05.
- Click OK.
Output obtained from MINITAB is given below:
The 95% confidence interval is given below:
Thus, the 95% confidence interval for the average value of pH before the addition of dyes when the dye weight and pH after the addition of dyes takes 1,000 and 6 is (5.250,5.383).
Interpretation:
It is 95% confident that average value of pH before the addition of dyes when the dye weight and pH after the addition of dyes takes 1,000 and 6 would lie between 5.250 and 5.383.
Want to see more full solutions like this?
Chapter 13 Solutions
WEBASSIGN ACCESS FOR PROBABILITY & STATS
- Question 1 The data shown in Table 1 are and R values for 24 samples of size n = 5 taken from a process producing bearings. The measurements are made on the inside diameter of the bearing, with only the last three decimals recorded (i.e., 34.5 should be 0.50345). Table 1: Bearing Diameter Data Sample Number I R Sample Number I R 1 34.5 3 13 35.4 8 2 34.2 4 14 34.0 6 3 31.6 4 15 37.1 5 4 31.5 4 16 34.9 7 5 35.0 5 17 33.5 4 6 34.1 6 18 31.7 3 7 32.6 4 19 34.0 8 8 33.8 3 20 35.1 9 34.8 7 21 33.7 2 10 33.6 8 22 32.8 1 11 31.9 3 23 33.5 3 12 38.6 9 24 34.2 2 (a) Set up and R charts on this process. Does the process seem to be in statistical control? If necessary, revise the trial control limits. [15 pts] (b) If specifications on this diameter are 0.5030±0.0010, find the percentage of nonconforming bearings pro- duced by this process. Assume that diameter is normally distributed. [10 pts] 1arrow_forward4. (5 pts) Conduct a chi-square contingency test (test of independence) to assess whether there is an association between the behavior of the elderly person (did not stop to talk, did stop to talk) and their likelihood of falling. Below, please state your null and alternative hypotheses, calculate your expected values and write them in the table, compute the test statistic, test the null by comparing your test statistic to the critical value in Table A (p. 713-714) of your textbook and/or estimating the P-value, and provide your conclusions in written form. Make sure to show your work. Did not stop walking to talk Stopped walking to talk Suffered a fall 12 11 Totals 23 Did not suffer a fall | 2 Totals 35 37 14 46 60 Tarrow_forwardQuestion 2 Parts manufactured by an injection molding process are subjected to a compressive strength test. Twenty samples of five parts each are collected, and the compressive strengths (in psi) are shown in Table 2. Table 2: Strength Data for Question 2 Sample Number x1 x2 23 x4 x5 R 1 83.0 2 88.6 78.3 78.8 3 85.7 75.8 84.3 81.2 78.7 75.7 77.0 71.0 84.2 81.0 79.1 7.3 80.2 17.6 75.2 80.4 10.4 4 80.8 74.4 82.5 74.1 75.7 77.5 8.4 5 83.4 78.4 82.6 78.2 78.9 80.3 5.2 File Preview 6 75.3 79.9 87.3 89.7 81.8 82.8 14.5 7 74.5 78.0 80.8 73.4 79.7 77.3 7.4 8 79.2 84.4 81.5 86.0 74.5 81.1 11.4 9 80.5 86.2 76.2 64.1 80.2 81.4 9.9 10 75.7 75.2 71.1 82.1 74.3 75.7 10.9 11 80.0 81.5 78.4 73.8 78.1 78.4 7.7 12 80.6 81.8 79.3 73.8 81.7 79.4 8.0 13 82.7 81.3 79.1 82.0 79.5 80.9 3.6 14 79.2 74.9 78.6 77.7 75.3 77.1 4.3 15 85.5 82.1 82.8 73.4 71.7 79.1 13.8 16 78.8 79.6 80.2 79.1 80.8 79.7 2.0 17 82.1 78.2 18 84.5 76.9 75.5 83.5 81.2 19 79.0 77.8 20 84.5 73.1 78.2 82.1 79.2 81.1 7.6 81.2 84.4 81.6 80.8…arrow_forward
- Name: Lab Time: Quiz 7 & 8 (Take Home) - due Wednesday, Feb. 26 Contingency Analysis (Ch. 9) In lab 5, part 3, you will create a mosaic plot and conducted a chi-square contingency test to evaluate whether elderly patients who did not stop walking to talk (vs. those who did stop) were more likely to suffer a fall in the next six months. I have tabulated the data below. Answer the questions below. Please show your calculations on this or a separate sheet. Did not stop walking to talk Stopped walking to talk Totals Suffered a fall Did not suffer a fall Totals 12 11 23 2 35 37 14 14 46 60 Quiz 7: 1. (2 pts) Compute the odds of falling for each group. Compute the odds ratio for those who did not stop walking vs. those who did stop walking. Interpret your result verbally.arrow_forwardSolve please and thank you!arrow_forward7. In a 2011 article, M. Radelet and G. Pierce reported a logistic prediction equation for the death penalty verdicts in North Carolina. Let Y denote whether a subject convicted of murder received the death penalty (1=yes), for the defendant's race h (h1, black; h = 2, white), victim's race i (i = 1, black; i = 2, white), and number of additional factors j (j = 0, 1, 2). For the model logit[P(Y = 1)] = a + ß₁₂ + By + B²², they reported = -5.26, D â BD = 0, BD = 0.17, BY = 0, BY = 0.91, B = 0, B = 2.02, B = 3.98. (a) Estimate the probability of receiving the death penalty for the group most likely to receive it. [4 pts] (b) If, instead, parameters used constraints 3D = BY = 35 = 0, report the esti- mates. [3 pts] h (c) If, instead, parameters used constraints Σ₁ = Σ₁ BY = Σ; B = 0, report the estimates. [3 pts] Hint the probabilities, odds and odds ratios do not change with constraints.arrow_forward
- Solve please and thank you!arrow_forwardSolve please and thank you!arrow_forwardQuestion 1:We want to evaluate the impact on the monetary economy for a company of two types of strategy (competitive strategy, cooperative strategy) adopted by buyers.Competitive strategy: strategy characterized by firm behavior aimed at obtaining concessions from the buyer.Cooperative strategy: a strategy based on a problem-solving negotiating attitude, with a high level of trust and cooperation.A random sample of 17 buyers took part in a negotiation experiment in which 9 buyers adopted the competitive strategy, and the other 8 the cooperative strategy. The savings obtained for each group of buyers are presented in the pdf that i sent: For this problem, we assume that the samples are random and come from two normal populations of unknown but equal variances.According to the theory, the average saving of buyers adopting a competitive strategy will be lower than that of buyers adopting a cooperative strategy.a) Specify the population identifications and the hypotheses H0 and H1…arrow_forward
- You assume that the annual incomes for certain workers are normal with a mean of $28,500 and a standard deviation of $2,400. What’s the chance that a randomly selected employee makes more than $30,000?What’s the chance that 36 randomly selected employees make more than $30,000, on average?arrow_forwardWhat’s the chance that a fair coin comes up heads more than 60 times when you toss it 100 times?arrow_forwardSuppose that you have a normal population of quiz scores with mean 40 and standard deviation 10. Select a random sample of 40. What’s the chance that the mean of the quiz scores won’t exceed 45?Select one individual from the population. What’s the chance that his/her quiz score won’t exceed 45?arrow_forward
- Big Ideas Math A Bridge To Success Algebra 1: Stu...AlgebraISBN:9781680331141Author:HOUGHTON MIFFLIN HARCOURTPublisher:Houghton Mifflin Harcourt
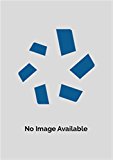