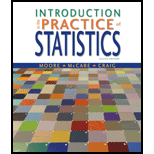
(a)
Section 1:
To find: The error degrees of freedom for Model 1.
(a)
Section 1:

Answer to Problem 19E
Solution: There are 200 error degrees of freedom in Model 1.
Explanation of Solution
Calculation: The two models have been provided in the problem. Model 1 discusses the effect of Gene expression
Here, the variable
The degrees of freedom for the model are calculated as
The total degrees of freedom are calculated as:
The error degrees of freedom are calculated as
Section 2:
To find: The error degrees of freedom for Model 2.
Section 2:

Answer to Problem 19E
Solution: There are 199 error degrees of freedom in Model 2.
Explanation of Solution
Calculation: The two models have been provided in the problem. Model 2 discusses the effect of Gene expression
Here, the variable
The degrees of freedom for the model are calculated as
The total degrees of freedom are calculated as
The error degrees of freedom are calculated as
(b)
To test: The null hypothesis that “the serotonin gene regression coefficient is equal to zero” against “it is not equal to zero,” and the corresponding test statistic and its P-value in Model 1.
(b)

Answer to Problem 19E
Solution: The result is significant, that is, the regression coefficient for gene expression
Explanation of Solution
Calculation: The null hypothesis to test whether the variable gene expression
The alternative hypothesis that the variable for gene expression
The level of significance is 0.05. It is provided that
The test statistic
The critical value of test statistic
The P-value for the test is calculated as
Conclusion: The P-value is less than the level of significance. Also, the test statistic results are
Hence, the null hypothesis gets rejected, that is, the result is significant.
(c)
Section 1:
To test: The null hypothesis that “the serotonin gene regression coefficient is equal to zero” against “it is not equal to zero,” and the corresponding test statistic and its P-value for Model 2.
(c)
Section 1:

Answer to Problem 19E
Solution: The result is significant, that is, the regression coefficient for gene expression
Explanation of Solution
Calculation: The null hypothesis to test whether the regression coefficient for gene expression
The alternative hypothesis that the regression coefficient for gene expression
The level of significance is 0.05. It is provided that
The test statistic
The critical value of test statistic
The P-value for the test is calculated as
Conclusion: The P-value is less than the level of significance. Also, the test statistic results are
Hence, the null hypothesis gets rejected, that is, the result is significant.
Section 2:
To test: The null hypothesis that “the rule-breaking composite coefficient is equal to zero” against “it is not equal to zero,” and the corresponding test statistic and its P-value for Model 2.
Section 2:

Answer to Problem 19E
Solution: The result is significant, that is, the coefficient for rule-breaking composite
Explanation of Solution
Calculation: The null hypothesis to test whether the coefficient of rule-breaking composite
The alternative hypothesis that the explanatory variable rule-breaking composite
The level of significance is 0.05. It is provided that
The test statistic
The critical value of test statistic
The P-value for the test is calculated as
Conclusion: The P-value is less than the level of significance. Also, the test statistic results are
Hence, the null hypothesis gets rejected, that is, the result is significant.
(d)
Section 1
Whether there lies a positive relationship between “the serotonin gene receptor expression level” and “popularity” after adjusting for rule-breaking.
(d)
Section 1

Answer to Problem 19E
Solution: The coefficients of the variables “gene expression” and “rule breaking” are positive. It highlights that there lies a positive relationship between the coefficients of the two variables in both the models.
Explanation of Solution
It is observed from the calculations in part (c) that there lies a relationship between the serotonin gene receptor expression and the popularity after adjusting for rule-breaking. Also, it is seen that the coefficients of gene expression and rule breaking for both the models are positive.
Section 2
To find: The comparison of upsurge in popularity when the gene expression increases by one unit and the rule breaking composite remains fixed.
Section 2

Answer to Problem 19E
Solution: When the variable gene expression is increased by one unit, the popularity increases by 0.204 units in Model 1, and there is an increase in popularity by 0.161 units in Model 2.
Explanation of Solution
Calculation: The regression model for Model 1 can be written as
When the gene expression is increased by 1 unit, the increase in the popularity is calculated as follows:
Also, the regression model for Model 2 can be written as
When the gene expression is increased by 1 unit, keeping all other variables constant, the increase in popularity is calculated as follows:
Want to see more full solutions like this?
Chapter 11 Solutions
Introduction to the Practice of Statistics: w/CrunchIt/EESEE Access Card
- Discuss and explain in the picturearrow_forwardBob and Teresa each collect their own samples to test the same hypothesis. Bob’s p-value turns out to be 0.05, and Teresa’s turns out to be 0.01. Why don’t Bob and Teresa get the same p-values? Who has stronger evidence against the null hypothesis: Bob or Teresa?arrow_forwardReview a classmate's Main Post. 1. State if you agree or disagree with the choices made for additional analysis that can be done beyond the frequency table. 2. Choose a measure of central tendency (mean, median, mode) that you would like to compute with the data beyond the frequency table. Complete either a or b below. a. Explain how that analysis can help you understand the data better. b. If you are currently unable to do that analysis, what do you think you could do to make it possible? If you do not think you can do anything, explain why it is not possible.arrow_forward
- 0|0|0|0 - Consider the time series X₁ and Y₁ = (I – B)² (I – B³)Xt. What transformations were performed on Xt to obtain Yt? seasonal difference of order 2 simple difference of order 5 seasonal difference of order 1 seasonal difference of order 5 simple difference of order 2arrow_forwardCalculate the 90% confidence interval for the population mean difference using the data in the attached image. I need to see where I went wrong.arrow_forwardMicrosoft Excel snapshot for random sampling: Also note the formula used for the last column 02 x✓ fx =INDEX(5852:58551, RANK(C2, $C$2:$C$51)) A B 1 No. States 2 1 ALABAMA Rand No. 0.925957526 3 2 ALASKA 0.372999976 4 3 ARIZONA 0.941323044 5 4 ARKANSAS 0.071266381 Random Sample CALIFORNIA NORTH CAROLINA ARKANSAS WASHINGTON G7 Microsoft Excel snapshot for systematic sampling: xfx INDEX(SD52:50551, F7) A B E F G 1 No. States Rand No. Random Sample population 50 2 1 ALABAMA 0.5296685 NEW HAMPSHIRE sample 10 3 2 ALASKA 0.4493186 OKLAHOMA k 5 4 3 ARIZONA 0.707914 KANSAS 5 4 ARKANSAS 0.4831379 NORTH DAKOTA 6 5 CALIFORNIA 0.7277162 INDIANA Random Sample Sample Name 7 6 COLORADO 0.5865002 MISSISSIPPI 8 7:ONNECTICU 0.7640596 ILLINOIS 9 8 DELAWARE 0.5783029 MISSOURI 525 10 15 INDIANA MARYLAND COLORADOarrow_forward
- Suppose the Internal Revenue Service reported that the mean tax refund for the year 2022 was $3401. Assume the standard deviation is $82.5 and that the amounts refunded follow a normal probability distribution. Solve the following three parts? (For the answer to question 14, 15, and 16, start with making a bell curve. Identify on the bell curve where is mean, X, and area(s) to be determined. 1.What percent of the refunds are more than $3,500? 2. What percent of the refunds are more than $3500 but less than $3579? 3. What percent of the refunds are more than $3325 but less than $3579?arrow_forwardA normal distribution has a mean of 50 and a standard deviation of 4. Solve the following three parts? 1. Compute the probability of a value between 44.0 and 55.0. (The question requires finding probability value between 44 and 55. Solve it in 3 steps. In the first step, use the above formula and x = 44, calculate probability value. In the second step repeat the first step with the only difference that x=55. In the third step, subtract the answer of the first part from the answer of the second part.) 2. Compute the probability of a value greater than 55.0. Use the same formula, x=55 and subtract the answer from 1. 3. Compute the probability of a value between 52.0 and 55.0. (The question requires finding probability value between 52 and 55. Solve it in 3 steps. In the first step, use the above formula and x = 52, calculate probability value. In the second step repeat the first step with the only difference that x=55. In the third step, subtract the answer of the first part from the…arrow_forwardIf a uniform distribution is defined over the interval from 6 to 10, then answer the followings: What is the mean of this uniform distribution? Show that the probability of any value between 6 and 10 is equal to 1.0 Find the probability of a value more than 7. Find the probability of a value between 7 and 9. The closing price of Schnur Sporting Goods Inc. common stock is uniformly distributed between $20 and $30 per share. What is the probability that the stock price will be: More than $27? Less than or equal to $24? The April rainfall in Flagstaff, Arizona, follows a uniform distribution between 0.5 and 3.00 inches. What is the mean amount of rainfall for the month? What is the probability of less than an inch of rain for the month? What is the probability of exactly 1.00 inch of rain? What is the probability of more than 1.50 inches of rain for the month? The best way to solve this problem is begin by a step by step creating a chart. Clearly mark the range, identifying the…arrow_forward
- Client 1 Weight before diet (pounds) Weight after diet (pounds) 128 120 2 131 123 3 140 141 4 178 170 5 121 118 6 136 136 7 118 121 8 136 127arrow_forwardClient 1 Weight before diet (pounds) Weight after diet (pounds) 128 120 2 131 123 3 140 141 4 178 170 5 121 118 6 136 136 7 118 121 8 136 127 a) Determine the mean change in patient weight from before to after the diet (after – before). What is the 95% confidence interval of this mean difference?arrow_forwardIn order to find probability, you can use this formula in Microsoft Excel: The best way to understand and solve these problems is by first drawing a bell curve and marking key points such as x, the mean, and the areas of interest. Once marked on the bell curve, figure out what calculations are needed to find the area of interest. =NORM.DIST(x, Mean, Standard Dev., TRUE). When the question mentions “greater than” you may have to subtract your answer from 1. When the question mentions “between (two values)”, you need to do separate calculation for both values and then subtract their results to get the answer. 1. Compute the probability of a value between 44.0 and 55.0. (The question requires finding probability value between 44 and 55. Solve it in 3 steps. In the first step, use the above formula and x = 44, calculate probability value. In the second step repeat the first step with the only difference that x=55. In the third step, subtract the answer of the first part from the…arrow_forward
- Big Ideas Math A Bridge To Success Algebra 1: Stu...AlgebraISBN:9781680331141Author:HOUGHTON MIFFLIN HARCOURTPublisher:Houghton Mifflin HarcourtGlencoe Algebra 1, Student Edition, 9780079039897...AlgebraISBN:9780079039897Author:CarterPublisher:McGraw HillHolt Mcdougal Larson Pre-algebra: Student Edition...AlgebraISBN:9780547587776Author:HOLT MCDOUGALPublisher:HOLT MCDOUGAL
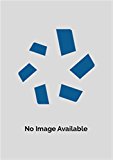
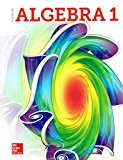
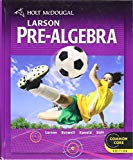