What is the general linear test presented in the previous question Q1.1 doing with respect to the full model fitted in Q1 description? Pick only one O Testing to see if your fitted model is the best model. O Testing to see if your fitted model is better than a model with all the covariates in it. O Testing to see if your fitted model is better than a model with only a single covariate in it. O Testing to see if your fitted model is better than a model with only an intercept in it.
What is the general linear test presented in the previous question Q1.1 doing with respect to the full model fitted in Q1 description? Pick only one O Testing to see if your fitted model is the best model. O Testing to see if your fitted model is better than a model with all the covariates in it. O Testing to see if your fitted model is better than a model with only a single covariate in it. O Testing to see if your fitted model is better than a model with only an intercept in it.
MATLAB: An Introduction with Applications
6th Edition
ISBN:9781119256830
Author:Amos Gilat
Publisher:Amos Gilat
Chapter1: Starting With Matlab
Section: Chapter Questions
Problem 1P
Related questions
Question

Transcribed Image Text:**Question:**
What is the general linear test presented in the previous question Q11 doing with respect to the full model fitted in Q1 description? Pick only one.
- ○ Testing to see if your fitted model is the best model.
- ○ Testing to see if your fitted model is better than a model with all the covariates in it.
- ○ Testing to see if your fitted model is better than a model with only a single covariate in it.
- ○ Testing to see if your fitted model is better than a model with only an intercept in it.
![**Question 1:**
Below is the R output of a model where the response is resting heart rate (Rest) and the predictors are Weight (Wgt, in pounds) and Gender (0 for female and 1 for male), with an interaction term between Weight and Gender.
Our population model is:
\[ Rest_i = \beta_0 + \beta_1 Wgt_i + \beta_2 Gender_i + \beta_3 Wgt_i \cdot Gender_i + \epsilon \]
```
lm(formula = Rest ~ Wgt + Gender + Wgt * Gender)
Coefficients:
Estimate Std. Error t value Pr(>|t|)
(Intercept) 70.25970 5.67488 12.381 <2e-16 ***
Wgt -0.01948 0.03138 -0.621 0.535
Gender 16.19178 9.97017 1.624 0.106
Wgt:Gender -0.10178 0.06803 -1.496 0.136
---
Residual standard error: 9.78 on 228 degrees of freedom
Multiple R-squared: 0.04625, Adjusted R-squared: 0.0337
F-statistic: 3.685 on 3 and 228 DF, p-value: 0.01274
```
**Explanation:**
- **Coefficients:**
- **(Intercept):** 70.25970, significant at p < 2e-16.
- **Wgt (Weight):** Coefficient of -0.01948, not statistically significant (p = 0.535).
- **Gender:** Coefficient of 16.19178, not statistically significant (p = 0.106).
- **Wgt:Gender (Interaction):** Coefficient of -0.10178, not statistically significant (p = 0.136).
- **Model Summary:**
- **Residual standard error:** 9.78, based on 228 degrees of freedom.
- **Multiple R-squared:** 0.04625, indicating the proportion of variance explained by the model.
- **Adjusted R-squared:** 0.0337, adjusted for the number of predictors.
-](/v2/_next/image?url=https%3A%2F%2Fcontent.bartleby.com%2Fqna-images%2Fquestion%2Fe5f558a7-14fc-4024-84d6-4debb1adc6f6%2F021713ea-d603-4014-8e60-c7f7316ff60c%2Ftz9jv28_processed.jpeg&w=3840&q=75)
Transcribed Image Text:**Question 1:**
Below is the R output of a model where the response is resting heart rate (Rest) and the predictors are Weight (Wgt, in pounds) and Gender (0 for female and 1 for male), with an interaction term between Weight and Gender.
Our population model is:
\[ Rest_i = \beta_0 + \beta_1 Wgt_i + \beta_2 Gender_i + \beta_3 Wgt_i \cdot Gender_i + \epsilon \]
```
lm(formula = Rest ~ Wgt + Gender + Wgt * Gender)
Coefficients:
Estimate Std. Error t value Pr(>|t|)
(Intercept) 70.25970 5.67488 12.381 <2e-16 ***
Wgt -0.01948 0.03138 -0.621 0.535
Gender 16.19178 9.97017 1.624 0.106
Wgt:Gender -0.10178 0.06803 -1.496 0.136
---
Residual standard error: 9.78 on 228 degrees of freedom
Multiple R-squared: 0.04625, Adjusted R-squared: 0.0337
F-statistic: 3.685 on 3 and 228 DF, p-value: 0.01274
```
**Explanation:**
- **Coefficients:**
- **(Intercept):** 70.25970, significant at p < 2e-16.
- **Wgt (Weight):** Coefficient of -0.01948, not statistically significant (p = 0.535).
- **Gender:** Coefficient of 16.19178, not statistically significant (p = 0.106).
- **Wgt:Gender (Interaction):** Coefficient of -0.10178, not statistically significant (p = 0.136).
- **Model Summary:**
- **Residual standard error:** 9.78, based on 228 degrees of freedom.
- **Multiple R-squared:** 0.04625, indicating the proportion of variance explained by the model.
- **Adjusted R-squared:** 0.0337, adjusted for the number of predictors.
-
Expert Solution

This question has been solved!
Explore an expertly crafted, step-by-step solution for a thorough understanding of key concepts.
This is a popular solution!
Trending now
This is a popular solution!
Step by step
Solved in 2 steps

Recommended textbooks for you
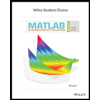
MATLAB: An Introduction with Applications
Statistics
ISBN:
9781119256830
Author:
Amos Gilat
Publisher:
John Wiley & Sons Inc
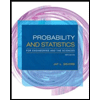
Probability and Statistics for Engineering and th…
Statistics
ISBN:
9781305251809
Author:
Jay L. Devore
Publisher:
Cengage Learning
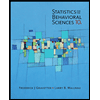
Statistics for The Behavioral Sciences (MindTap C…
Statistics
ISBN:
9781305504912
Author:
Frederick J Gravetter, Larry B. Wallnau
Publisher:
Cengage Learning
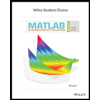
MATLAB: An Introduction with Applications
Statistics
ISBN:
9781119256830
Author:
Amos Gilat
Publisher:
John Wiley & Sons Inc
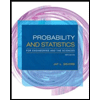
Probability and Statistics for Engineering and th…
Statistics
ISBN:
9781305251809
Author:
Jay L. Devore
Publisher:
Cengage Learning
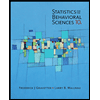
Statistics for The Behavioral Sciences (MindTap C…
Statistics
ISBN:
9781305504912
Author:
Frederick J Gravetter, Larry B. Wallnau
Publisher:
Cengage Learning
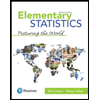
Elementary Statistics: Picturing the World (7th E…
Statistics
ISBN:
9780134683416
Author:
Ron Larson, Betsy Farber
Publisher:
PEARSON
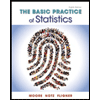
The Basic Practice of Statistics
Statistics
ISBN:
9781319042578
Author:
David S. Moore, William I. Notz, Michael A. Fligner
Publisher:
W. H. Freeman
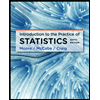
Introduction to the Practice of Statistics
Statistics
ISBN:
9781319013387
Author:
David S. Moore, George P. McCabe, Bruce A. Craig
Publisher:
W. H. Freeman