What are the various ways of tree trimming (in data mining)? Explain in detail?
What are the various ways of tree trimming (in data mining)? Explain in detail?
Computer Networking: A Top-Down Approach (7th Edition)
7th Edition
ISBN:9780133594140
Author:James Kurose, Keith Ross
Publisher:James Kurose, Keith Ross
Chapter1: Computer Networks And The Internet
Section: Chapter Questions
Problem R1RQ: What is the difference between a host and an end system? List several different types of end...
Related questions
Question

Transcribed Image Text:**Question: What are the various ways of tree trimming (in data mining)? Explain in detail?**
This appears to be a prompt related to data mining techniques. There are various methodologies for tree trimming or pruning in data mining to enhance decision tree performance by removing sections that may be noisy or less relevant.
### Detailed Explanation of Tree Trimming in Data Mining
1. **Pre-pruning (Early Stopping):**
- Pre-pruning involves halting the tree-building process before it fully reflects the training data. Criteria for stopping could include setting a maximum tree depth, minimum number of samples in a node, or a threshold for splitting nodes.
- **Benefits:** Helps in reducing overfitting by stopping before the tree becomes overly specific to the training data.
2. **Post-pruning:**
- Post-pruning involves building the full decision tree first and then removing nodes that add little predictive power. Techniques include reduced-error pruning and cost complexity pruning.
- **Benefits:** Generally leads to a more precise final tree as it evaluates the entire structure before making modifications.
3. **Reduced-Error Pruning:**
- In this method, nodes are removed (pruned) if their removal does not deteriorate the decision tree’s accuracy on a validation set. The idea is to replace a subtree with a leaf node if it increases or does not change the accuracy.
- **Steps:**
* Build the decision tree on the training data.
* Evaluate the tree’s performance on a validation set.
* Prune nodes and re-evaluate until an optimal tree size is obtained.
4. **Cost Complexity Pruning (CCP):**
- CCP prunes the tree by weighing the trade-off between the tree's complexity (number of nodes) and its fit to the training data. It uses a regularization parameter to balance this trade-off.
- **Steps:**
* Build the complete tree.
* Calculate the cost complexity for each subtree.
* Prune the subtree whose removal results in the smallest increase in error until an ideal tree size is reached.
5. **Minimum Error Pruning:**
- This approach prunes the tree to minimize classification error rates. Nodes are pruned if their removal results in a lower or equal error rate on the validation set.
6. **Pessimistic Pruning:**
- Adjusts for the potential over-optimism of the training error rate by adding a correction factor
Expert Solution

This question has been solved!
Explore an expertly crafted, step-by-step solution for a thorough understanding of key concepts.
Step by step
Solved in 2 steps

Recommended textbooks for you
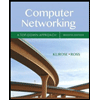
Computer Networking: A Top-Down Approach (7th Edi…
Computer Engineering
ISBN:
9780133594140
Author:
James Kurose, Keith Ross
Publisher:
PEARSON
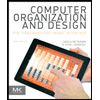
Computer Organization and Design MIPS Edition, Fi…
Computer Engineering
ISBN:
9780124077263
Author:
David A. Patterson, John L. Hennessy
Publisher:
Elsevier Science
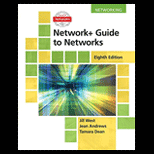
Network+ Guide to Networks (MindTap Course List)
Computer Engineering
ISBN:
9781337569330
Author:
Jill West, Tamara Dean, Jean Andrews
Publisher:
Cengage Learning
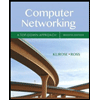
Computer Networking: A Top-Down Approach (7th Edi…
Computer Engineering
ISBN:
9780133594140
Author:
James Kurose, Keith Ross
Publisher:
PEARSON
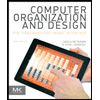
Computer Organization and Design MIPS Edition, Fi…
Computer Engineering
ISBN:
9780124077263
Author:
David A. Patterson, John L. Hennessy
Publisher:
Elsevier Science
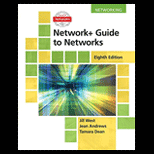
Network+ Guide to Networks (MindTap Course List)
Computer Engineering
ISBN:
9781337569330
Author:
Jill West, Tamara Dean, Jean Andrews
Publisher:
Cengage Learning
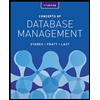
Concepts of Database Management
Computer Engineering
ISBN:
9781337093422
Author:
Joy L. Starks, Philip J. Pratt, Mary Z. Last
Publisher:
Cengage Learning
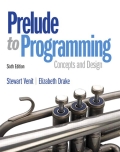
Prelude to Programming
Computer Engineering
ISBN:
9780133750423
Author:
VENIT, Stewart
Publisher:
Pearson Education
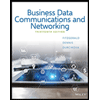
Sc Business Data Communications and Networking, T…
Computer Engineering
ISBN:
9781119368830
Author:
FITZGERALD
Publisher:
WILEY