What are the drawbacks of training a deep neural network with a small amount of labeled data ? The model does not converge because the training is not stable. O Training error becomes very high and, testing and validation errors become very low due to high bias (under fitting). O Because the data set is small and training is very quick, the inference during test time is also very quick. Training errors may be very low but test and validation errors may be very large due to over-fitting. Consider a deep neural network (fruit network) that has been trained to classify a labelled set of images of fruits based on their species (say C, categories). You are presented with a tiny labeled dataset of images of vegetables (a few thousand images with C categories). The goal is to train a model to classify images of vegetables. Which of the below approaches would work well? O Apply a feature extractor like SIFT or HoG on the vegetable images and use the features to train a Support Vector Machine classifier. O The last layer of the fruit network can be replaced with a classification layer based on the number of vegetable categories and the network can be fine-tuned with the tiny labeled set of images of vegetables. O Retrain the fruit network with images of vegetables. O The fruit network can be used without any modifications to classify images of vegetables since it has already
What are the drawbacks of training a deep neural network with a small amount of labeled data ? The model does not converge because the training is not stable. O Training error becomes very high and, testing and validation errors become very low due to high bias (under fitting). O Because the data set is small and training is very quick, the inference during test time is also very quick. Training errors may be very low but test and validation errors may be very large due to over-fitting. Consider a deep neural network (fruit network) that has been trained to classify a labelled set of images of fruits based on their species (say C, categories). You are presented with a tiny labeled dataset of images of vegetables (a few thousand images with C categories). The goal is to train a model to classify images of vegetables. Which of the below approaches would work well? O Apply a feature extractor like SIFT or HoG on the vegetable images and use the features to train a Support Vector Machine classifier. O The last layer of the fruit network can be replaced with a classification layer based on the number of vegetable categories and the network can be fine-tuned with the tiny labeled set of images of vegetables. O Retrain the fruit network with images of vegetables. O The fruit network can be used without any modifications to classify images of vegetables since it has already
Computer Networking: A Top-Down Approach (7th Edition)
7th Edition
ISBN:9780133594140
Author:James Kurose, Keith Ross
Publisher:James Kurose, Keith Ross
Chapter1: Computer Networks And The Internet
Section: Chapter Questions
Problem R1RQ: What is the difference between a host and an end system? List several different types of end...
Related questions
Question
PLEASE ENSURE ANSWERS ARE CORRECT AND DONT JUST GUESS

Transcribed Image Text:What are the drawbacks of training a deep neural network with a small amount of labeled data ?
O The model does not converge because the training is not stable.
O Training error becomes very high and, testing and validation errors become very low due to high bias (under
fitting).
Because the data set is small and training is very quick, the Inference during test time is also very quick.
Training errors may be very low but test and validation errors may be very large due to over-fitting,
Consider a deep neural network (fruit network) that has been trained to classify a labelled set of Images of fruits
based on their species (say C, categories). You are presented with a tiny labeled dataset of images of vegetables (a
few thousand images with C categories). The goal is to train a model to classify images of vegetables,. Which of the
below approaches would work well?
Apply a feature extractor like SIFT or HoG on the vegetable images and use the features to train a Support
Vector Machine classifier.
The last layer of the fruit network can be replaced with a classification layer based on the number of
vegetable categories and the network can be fine-tuned with the tiny labeled set of images of vegetables.
O Retrain the fruit network with images of vegetables.
O The fruit network can be used without any modifications to classify images of vegetables since it has already
been trained on the labelled images of fruits and fruits look similar to vegetables.
Expert Solution

This question has been solved!
Explore an expertly crafted, step-by-step solution for a thorough understanding of key concepts.
This is a popular solution!
Trending now
This is a popular solution!
Step by step
Solved in 2 steps

Recommended textbooks for you
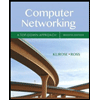
Computer Networking: A Top-Down Approach (7th Edi…
Computer Engineering
ISBN:
9780133594140
Author:
James Kurose, Keith Ross
Publisher:
PEARSON
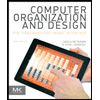
Computer Organization and Design MIPS Edition, Fi…
Computer Engineering
ISBN:
9780124077263
Author:
David A. Patterson, John L. Hennessy
Publisher:
Elsevier Science
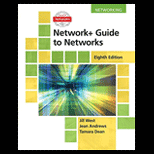
Network+ Guide to Networks (MindTap Course List)
Computer Engineering
ISBN:
9781337569330
Author:
Jill West, Tamara Dean, Jean Andrews
Publisher:
Cengage Learning
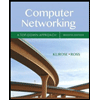
Computer Networking: A Top-Down Approach (7th Edi…
Computer Engineering
ISBN:
9780133594140
Author:
James Kurose, Keith Ross
Publisher:
PEARSON
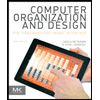
Computer Organization and Design MIPS Edition, Fi…
Computer Engineering
ISBN:
9780124077263
Author:
David A. Patterson, John L. Hennessy
Publisher:
Elsevier Science
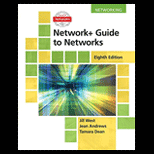
Network+ Guide to Networks (MindTap Course List)
Computer Engineering
ISBN:
9781337569330
Author:
Jill West, Tamara Dean, Jean Andrews
Publisher:
Cengage Learning
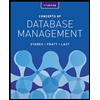
Concepts of Database Management
Computer Engineering
ISBN:
9781337093422
Author:
Joy L. Starks, Philip J. Pratt, Mary Z. Last
Publisher:
Cengage Learning
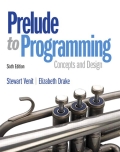
Prelude to Programming
Computer Engineering
ISBN:
9780133750423
Author:
VENIT, Stewart
Publisher:
Pearson Education
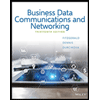
Sc Business Data Communications and Networking, T…
Computer Engineering
ISBN:
9781119368830
Author:
FITZGERALD
Publisher:
WILEY