We have talked about the fact that the sample mean estimator X = X; is an unbiased estimator of the mean u for identically distributed X1, X2, ..., Xn: E[X] = variance estimator, on the other hand, is not an unbiased estimate of the true variance o²: for µ. The straightforward Vý = E1(X; – X)², we get that E[V½] = (1 – )o². Instead, the following bias-corrected sample variance estimator is unbiased: V = „4 E (X; – X)². This unbiased estimator is typically what is called the sample variance. Use the fact that E[V] = o² to show that E[V] = (1 – )0². Hint: The proof is short, it can be done in a few lines. (b) that Var[X] = 02, for iid variables with variance o?. We can similarly ask what the variance is We also discussed the variance of the sample mean estimator, and concluded
We have talked about the fact that the sample mean estimator X = X; is an unbiased estimator of the mean u for identically distributed X1, X2, ..., Xn: E[X] = variance estimator, on the other hand, is not an unbiased estimate of the true variance o²: for µ. The straightforward Vý = E1(X; – X)², we get that E[V½] = (1 – )o². Instead, the following bias-corrected sample variance estimator is unbiased: V = „4 E (X; – X)². This unbiased estimator is typically what is called the sample variance. Use the fact that E[V] = o² to show that E[V] = (1 – )0². Hint: The proof is short, it can be done in a few lines. (b) that Var[X] = 02, for iid variables with variance o?. We can similarly ask what the variance is We also discussed the variance of the sample mean estimator, and concluded
College Algebra
7th Edition
ISBN:9781305115545
Author:James Stewart, Lothar Redlin, Saleem Watson
Publisher:James Stewart, Lothar Redlin, Saleem Watson
Chapter1: Equations And Graphs
Section: Chapter Questions
Problem 10T: Olympic Pole Vault The graph in Figure 7 indicates that in recent years the winning Olympic men’s...
Related questions
Question
![We have talked about the fact that the sample mean estimator X = X; is an unbiased
estimator of the mean u for identically distributed X1, X2, ..., Xn: E[X] =
variance estimator, on the other hand, is not an unbiased estimate of the true variance o²: for
µ. The straightforward
Vý = E1(X; – X)², we get that E[V½] = (1 – )o². Instead, the following bias-corrected sample
variance estimator is unbiased: V = „4 E (X; – X)². This unbiased estimator is typically what
is called the sample variance.
Use the fact that E[V] = o² to show that E[V] = (1 – )0². Hint: The proof is
short, it can be done in a few lines.
(b)
that Var[X] = 02, for iid variables with variance o?. We can similarly ask what the variance is
We also discussed the variance of the sample mean estimator, and concluded](/v2/_next/image?url=https%3A%2F%2Fcontent.bartleby.com%2Fqna-images%2Fquestion%2F36a7a892-d2a2-4a6f-a8a2-55ad3cac8d8b%2F00f865a7-d8ca-4abf-8a5e-0242ed73e5b9%2Fescsv4_processed.png&w=3840&q=75)
Transcribed Image Text:We have talked about the fact that the sample mean estimator X = X; is an unbiased
estimator of the mean u for identically distributed X1, X2, ..., Xn: E[X] =
variance estimator, on the other hand, is not an unbiased estimate of the true variance o²: for
µ. The straightforward
Vý = E1(X; – X)², we get that E[V½] = (1 – )o². Instead, the following bias-corrected sample
variance estimator is unbiased: V = „4 E (X; – X)². This unbiased estimator is typically what
is called the sample variance.
Use the fact that E[V] = o² to show that E[V] = (1 – )0². Hint: The proof is
short, it can be done in a few lines.
(b)
that Var[X] = 02, for iid variables with variance o?. We can similarly ask what the variance is
We also discussed the variance of the sample mean estimator, and concluded
![of the sample variance estimator. Deriving the formula is a bit more complex for general random
variables, so let's assume the X; are zero-mean Gaussian. Note that for zero-mean Gaussian X;,
we can use V =:E1 X}, which is unbiased (i.e., E[V] = o²). Then we know that the following
is true (though we omit the derivation): Var[V] = 2(n-1),4.
This variance enables us to use Chebyshev's inequality, to get a confidence estimate. Recall
that Chebyshev's inequality states that for a random variable Y with known variance v, we know
that Pr(|Y – E[Y]| < e) > 1 – v/e². After seeing 10 samples from a distribution, do you think
you will have a tighter confidence estimate around the sample mean X or the sample variance V?
n2](/v2/_next/image?url=https%3A%2F%2Fcontent.bartleby.com%2Fqna-images%2Fquestion%2F36a7a892-d2a2-4a6f-a8a2-55ad3cac8d8b%2F00f865a7-d8ca-4abf-8a5e-0242ed73e5b9%2Fxsaxzol_processed.png&w=3840&q=75)
Transcribed Image Text:of the sample variance estimator. Deriving the formula is a bit more complex for general random
variables, so let's assume the X; are zero-mean Gaussian. Note that for zero-mean Gaussian X;,
we can use V =:E1 X}, which is unbiased (i.e., E[V] = o²). Then we know that the following
is true (though we omit the derivation): Var[V] = 2(n-1),4.
This variance enables us to use Chebyshev's inequality, to get a confidence estimate. Recall
that Chebyshev's inequality states that for a random variable Y with known variance v, we know
that Pr(|Y – E[Y]| < e) > 1 – v/e². After seeing 10 samples from a distribution, do you think
you will have a tighter confidence estimate around the sample mean X or the sample variance V?
n2
Expert Solution

This question has been solved!
Explore an expertly crafted, step-by-step solution for a thorough understanding of key concepts.
This is a popular solution!
Trending now
This is a popular solution!
Step by step
Solved in 2 steps with 2 images

Recommended textbooks for you
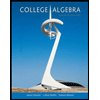
College Algebra
Algebra
ISBN:
9781305115545
Author:
James Stewart, Lothar Redlin, Saleem Watson
Publisher:
Cengage Learning
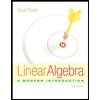
Linear Algebra: A Modern Introduction
Algebra
ISBN:
9781285463247
Author:
David Poole
Publisher:
Cengage Learning
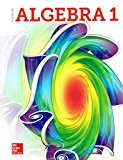
Glencoe Algebra 1, Student Edition, 9780079039897…
Algebra
ISBN:
9780079039897
Author:
Carter
Publisher:
McGraw Hill
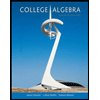
College Algebra
Algebra
ISBN:
9781305115545
Author:
James Stewart, Lothar Redlin, Saleem Watson
Publisher:
Cengage Learning
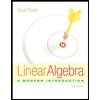
Linear Algebra: A Modern Introduction
Algebra
ISBN:
9781285463247
Author:
David Poole
Publisher:
Cengage Learning
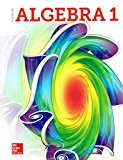
Glencoe Algebra 1, Student Edition, 9780079039897…
Algebra
ISBN:
9780079039897
Author:
Carter
Publisher:
McGraw Hill
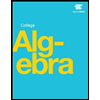
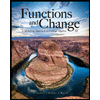
Functions and Change: A Modeling Approach to Coll…
Algebra
ISBN:
9781337111348
Author:
Bruce Crauder, Benny Evans, Alan Noell
Publisher:
Cengage Learning