Wait, what happened? Our training process literally blew up, leading to losses becom- ing inf. This is a clear sign that params is receiving updates that are too large, and their values start oscillating back and forth as each update overshoots and the next overcorrects even more. The optimization process is unstable: it diverges instead of converging to a minimum. We want to see smaller and smaller updates to params, not larger, as shown in figure 5.8. A 2 2 D E Figure 5.8 Top: Diverging optimization on a convex function (parabola-like) due to large steps. Bottom: Converging optimization with small steps. How can we limit the magnitude of learning_rate * grad? Well, that looks easy. We could simply choose a smaller learning_rate, and indeed, the learning rate is one of the things we typically change when training does not go as well as we would like. We usually change learning rates by orders of magnitude, so we might try with le-3 or le-4, which would decrease the magnitude of the updates by orders of magnitude. Let's go with le-4 and see how it works out:
Wait, what happened? Our training process literally blew up, leading to losses becom- ing inf. This is a clear sign that params is receiving updates that are too large, and their values start oscillating back and forth as each update overshoots and the next overcorrects even more. The optimization process is unstable: it diverges instead of converging to a minimum. We want to see smaller and smaller updates to params, not larger, as shown in figure 5.8. A 2 2 D E Figure 5.8 Top: Diverging optimization on a convex function (parabola-like) due to large steps. Bottom: Converging optimization with small steps. How can we limit the magnitude of learning_rate * grad? Well, that looks easy. We could simply choose a smaller learning_rate, and indeed, the learning rate is one of the things we typically change when training does not go as well as we would like. We usually change learning rates by orders of magnitude, so we might try with le-3 or le-4, which would decrease the magnitude of the updates by orders of magnitude. Let's go with le-4 and see how it works out:
Computer Networking: A Top-Down Approach (7th Edition)
7th Edition
ISBN:9780133594140
Author:James Kurose, Keith Ross
Publisher:James Kurose, Keith Ross
Chapter1: Computer Networks And The Internet
Section: Chapter Questions
Problem R1RQ: What is the difference between a host and an end system? List several different types of end...
Related questions
Question
machine learning coding

Transcribed Image Text:Wait, what happened? Our training process literally blew up, leading to losses becom-
ing inf. This is a clear sign that params is receiving updates that are too large, and
their values start oscillating back and forth as each update overshoots and the next
overcorrects even more. The optimization process is unstable: it diverges instead of
converging to a minimum. We want to see smaller and smaller updates to params, not
larger, as shown in figure 5.8.
A
2
E
Figure 5.8 Top: Diverging optimization on a convex function (parabola-like) due to large steps.
Bottom: Converging optimization with small steps.
How can we limit the magnitude of learning_rate * grad? Well, that looks easy. We
could simply choose a smaller learning_rate, and indeed, the learning rate is one of
the things we typically change when training does not go as well as we would like. We
usually change learning rates by orders of magnitude, so we might try with le-3 or
le-4, which would decrease the magnitude of the updates by orders of magnitude.
Let's go with le-4 and see how it works out:
Expert Solution

This question has been solved!
Explore an expertly crafted, step-by-step solution for a thorough understanding of key concepts.
Step by step
Solved in 2 steps with 1 images

Recommended textbooks for you
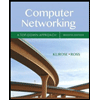
Computer Networking: A Top-Down Approach (7th Edi…
Computer Engineering
ISBN:
9780133594140
Author:
James Kurose, Keith Ross
Publisher:
PEARSON
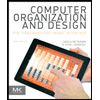
Computer Organization and Design MIPS Edition, Fi…
Computer Engineering
ISBN:
9780124077263
Author:
David A. Patterson, John L. Hennessy
Publisher:
Elsevier Science
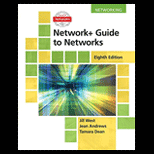
Network+ Guide to Networks (MindTap Course List)
Computer Engineering
ISBN:
9781337569330
Author:
Jill West, Tamara Dean, Jean Andrews
Publisher:
Cengage Learning
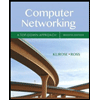
Computer Networking: A Top-Down Approach (7th Edi…
Computer Engineering
ISBN:
9780133594140
Author:
James Kurose, Keith Ross
Publisher:
PEARSON
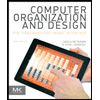
Computer Organization and Design MIPS Edition, Fi…
Computer Engineering
ISBN:
9780124077263
Author:
David A. Patterson, John L. Hennessy
Publisher:
Elsevier Science
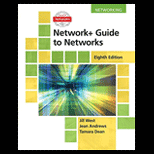
Network+ Guide to Networks (MindTap Course List)
Computer Engineering
ISBN:
9781337569330
Author:
Jill West, Tamara Dean, Jean Andrews
Publisher:
Cengage Learning
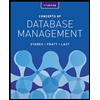
Concepts of Database Management
Computer Engineering
ISBN:
9781337093422
Author:
Joy L. Starks, Philip J. Pratt, Mary Z. Last
Publisher:
Cengage Learning
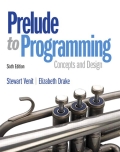
Prelude to Programming
Computer Engineering
ISBN:
9780133750423
Author:
VENIT, Stewart
Publisher:
Pearson Education
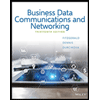
Sc Business Data Communications and Networking, T…
Computer Engineering
ISBN:
9781119368830
Author:
FITZGERALD
Publisher:
WILEY