Using the fact that r (k, 2) = x₂ for k € Z., i.e., A Camma distribution with parameters (k, 2) is a Chi-squared distribution with 2k degrees of freedom, express the sampling distribution for LE in terms of Xan Show that the confidence interval in part (6) can be equivalently expressed as CI(0; a)- 2nX €₂x1-a/2 where x is the a quantile of the x distribution. " 2nX X₂m/a/2 X²/2]
Using the fact that r (k, 2) = x₂ for k € Z., i.e., A Camma distribution with parameters (k, 2) is a Chi-squared distribution with 2k degrees of freedom, express the sampling distribution for LE in terms of Xan Show that the confidence interval in part (6) can be equivalently expressed as CI(0; a)- 2nX €₂x1-a/2 where x is the a quantile of the x distribution. " 2nX X₂m/a/2 X²/2]
MATLAB: An Introduction with Applications
6th Edition
ISBN:9781119256830
Author:Amos Gilat
Publisher:Amos Gilat
Chapter1: Starting With Matlab
Section: Chapter Questions
Problem 1P
Related questions
Question
I need answers and details step by step of question 7, 8, and 9
Question 7, 8 and 9
![7.
Using the fact that
T (k, 2) = Xak
for k € Z+, i.e., A Gamma distribution with parameters (k, 2) is a Chi-squared distribution with 2k
degrees of freedom, express the sampling distribution for MLE in terms of Xan
Show that the confidence interval in part (6) can be equivalently expressed as
CI(0; a)
- [2
2n.X
2n.X
[X₂1-a/2 X²/2]
where X is the a quantile of the x distribution.
8.
Using the central limit theorem for maximum likelihood estimators we know that the large sample
sampling distribution for MILE is approximately
P(-20/255
1
OMLEN(0, Var(@MLE))
We also know that Var(0) = f(0), where f is some function of which you obtained in part 3.
Therefore,
0 - ⓇMLE
√1(0)
A (1-a) large sample confidence confidence interval using this approximation is given by
0 - ⓇMLE
√1(0)
CI(0; a) =
≈N(0, 1).
≤a/2=1-a.
Using your answer from part (3) in the expression above and without approximating f(0), with
f(0), derive the large sample confidence interval for 0, i.e., find and such that
CI(0; a) [lasua].
9. (
In class, we saw that when Var(8) = f(0), i.e., when the variance of your sample estimator
depends on the unknown parameter 0, it is reasonable to use f() in place of f(0) to obtain an
estimator for the variance, i.e.,
Var(ÔMLE) = f(ÔMLE),
where, f is again a placeholder for the answer you obtained in part (3). Using this method, show
that the approximate large sample confidence interval you obtain for is given by
− [x (1 – ²00), X (1 + ³07 )]](/v2/_next/image?url=https%3A%2F%2Fcontent.bartleby.com%2Fqna-images%2Fquestion%2Fd7c2748e-5ba1-4f2f-bc7d-c6e1ab8bba12%2F8bb6ab93-74cd-4f00-8f6f-6278fc23c785%2Ffhssfab_processed.png&w=3840&q=75)
Transcribed Image Text:7.
Using the fact that
T (k, 2) = Xak
for k € Z+, i.e., A Gamma distribution with parameters (k, 2) is a Chi-squared distribution with 2k
degrees of freedom, express the sampling distribution for MLE in terms of Xan
Show that the confidence interval in part (6) can be equivalently expressed as
CI(0; a)
- [2
2n.X
2n.X
[X₂1-a/2 X²/2]
where X is the a quantile of the x distribution.
8.
Using the central limit theorem for maximum likelihood estimators we know that the large sample
sampling distribution for MILE is approximately
P(-20/255
1
OMLEN(0, Var(@MLE))
We also know that Var(0) = f(0), where f is some function of which you obtained in part 3.
Therefore,
0 - ⓇMLE
√1(0)
A (1-a) large sample confidence confidence interval using this approximation is given by
0 - ⓇMLE
√1(0)
CI(0; a) =
≈N(0, 1).
≤a/2=1-a.
Using your answer from part (3) in the expression above and without approximating f(0), with
f(0), derive the large sample confidence interval for 0, i.e., find and such that
CI(0; a) [lasua].
9. (
In class, we saw that when Var(8) = f(0), i.e., when the variance of your sample estimator
depends on the unknown parameter 0, it is reasonable to use f() in place of f(0) to obtain an
estimator for the variance, i.e.,
Var(ÔMLE) = f(ÔMLE),
where, f is again a placeholder for the answer you obtained in part (3). Using this method, show
that the approximate large sample confidence interval you obtain for is given by
− [x (1 – ²00), X (1 + ³07 )]

Transcribed Image Text:iid
Let X₁, X2,..., X, * Exp(0) be a sample from an exponential distribution with (unknown)
parameter and pdf given by
1
0
(Note that this is the "scale" parametrization for the Exponential distribution)
1.
2.
fx(x; 0)
Derive the method of moments estimator MM. Is it biased or unbiased?
3.
=
Derive the method of maximum likelihood estimator MLE. Is it biased or unbiased?
Compute Var (ÔMLE), the variance of the maximum likelihood estimator.
4. (8
Using the fact that Exp(0) = [(1, 0), i.e. an Exponential distribution is just a Gamma distribution
with shape parameter 1, derive the exact sampling distribution for ÔMLE-
-x/0.
5.
Using your answer to part (4.), use the properties for transformations of random variables to find
the distribution of the new random variable
Y = H (ÔMLE, 0)
Does the distribution of Y depend on 0?
=
CI(0; a) =
ÔMLE
0
6.
Using your answer from part (5.) show that the exact two-sided confidence interval for with
confidence level (1-x) is given by
nX
nX
In,1; (1-a/2) In,1;0/2
where I'n,1,a denotes the a quantile of the Gamma distribution I'(n, 1)
7
Expert Solution

This question has been solved!
Explore an expertly crafted, step-by-step solution for a thorough understanding of key concepts.
Step by step
Solved in 2 steps

Recommended textbooks for you
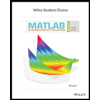
MATLAB: An Introduction with Applications
Statistics
ISBN:
9781119256830
Author:
Amos Gilat
Publisher:
John Wiley & Sons Inc
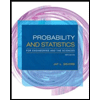
Probability and Statistics for Engineering and th…
Statistics
ISBN:
9781305251809
Author:
Jay L. Devore
Publisher:
Cengage Learning
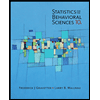
Statistics for The Behavioral Sciences (MindTap C…
Statistics
ISBN:
9781305504912
Author:
Frederick J Gravetter, Larry B. Wallnau
Publisher:
Cengage Learning
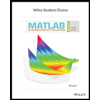
MATLAB: An Introduction with Applications
Statistics
ISBN:
9781119256830
Author:
Amos Gilat
Publisher:
John Wiley & Sons Inc
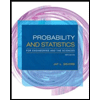
Probability and Statistics for Engineering and th…
Statistics
ISBN:
9781305251809
Author:
Jay L. Devore
Publisher:
Cengage Learning
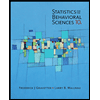
Statistics for The Behavioral Sciences (MindTap C…
Statistics
ISBN:
9781305504912
Author:
Frederick J Gravetter, Larry B. Wallnau
Publisher:
Cengage Learning
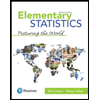
Elementary Statistics: Picturing the World (7th E…
Statistics
ISBN:
9780134683416
Author:
Ron Larson, Betsy Farber
Publisher:
PEARSON
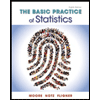
The Basic Practice of Statistics
Statistics
ISBN:
9781319042578
Author:
David S. Moore, William I. Notz, Michael A. Fligner
Publisher:
W. H. Freeman
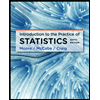
Introduction to the Practice of Statistics
Statistics
ISBN:
9781319013387
Author:
David S. Moore, George P. McCabe, Bruce A. Craig
Publisher:
W. H. Freeman