Use the k-means algorithm and Euclidean distance to cluster the following samples into 3 clusters: A1= (2,10), A2= (2,5), A3= (8,4), A4= (5,8), A5= (7,5), A6= (6,4), A7= (1,2), A8= (4,9). Suppose that the initial seeds (centers of each cluster) are A1, A4 and A7. Run the k-means algorithm for 1 epoch only. At the end of this epoch show: (i)The new clusters (i.e. the examples belonging to each cluster The centers of the new clusters Draw a 10 by 10 space with all the 8 points and show the clusters after the first epoch and the new centroids.
QUESTION ONE
Use the k-means
A1= (2,10), A2= (2,5), A3= (8,4), A4= (5,8), A5= (7,5), A6= (6,4), A7= (1,2), A8= (4,9).
Suppose that the initial seeds (centers of each cluster) are A1, A4 and A7. Run the k-means algorithm for 1 epoch only. At the end of this epoch show:
- (i)The new clusters (i.e. the examples belonging to each cluster
- The centers of the new clusters
- Draw a 10 by 10 space with all the 8 points and show the clusters after the first epoch and the new centroids.
- How many more iterations are needed to converge? Draw the result for each epoch.
- Use the Nearest Neighbor clustering algorithm and Euclidean distance to cluster the examples from the previous exercise: A1= (2,10), A2= (2,5), A3= (8,4), A4= (5,8), A5= (7,5), A6= (6,4), A7= (1,2), A8= (4,9). Suppose that the threshold t is 4.
- Explain the difference between feed forward neural networks and deep learning networks.
QUESTION THREE
- Consider the K-means cost function for clustering N d-dimensional data points into K Compute the optimal parameters when K = 1.
- Derive the optimal parameters of the model when K = N.
Now, we use Gaussian mixture models (GMM) to cluster the data, wherein the covariance matrices are fixed and given in advance. In addition, we aim to obtain the correct number of clusters via Akaike information criterion (AIC) and Bayesian information criterion (BIC).
Assume we know that all the estimated clusters should have the same size. Describe how you would apply AIC and BIC to compute the correct number of clusters. Discard the steps for calculating the log-likelihoods.



Trending now
This is a popular solution!
Step by step
Solved in 5 steps with 2 images

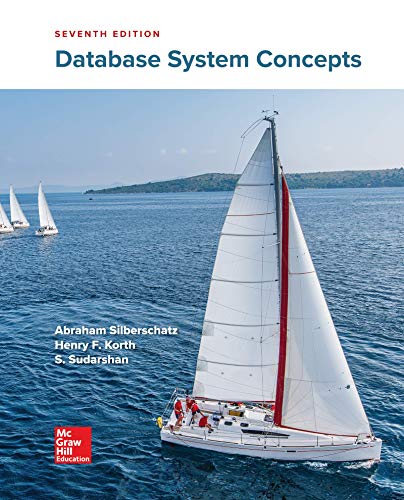
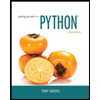
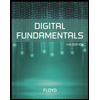
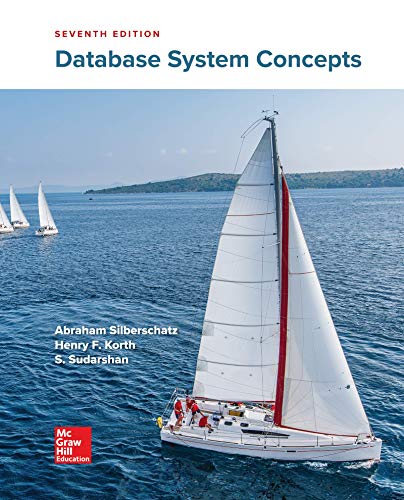
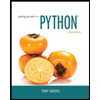
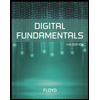
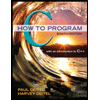
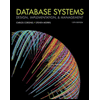
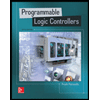