Туре? Patrons? French Italian Thai Burger Nore Some 136 8 10 No Yes Hungry? No Yes 4 12 2 10 (a) (b) Figure 18.4 Splitting the examples by testing on attributes. At each node we show the positive (light boxes) and negative (dark boxes) examples remaining. (a) Splitting on Type brings us no nearer to distinguishing between positive and negative examples (b) Splitting on Patrons does a good job of separating positive and negative examples. After splitting on Patrons, Hungry is a fairly good second test. bination of attribute values, and we retum a default value calculated from the plurality classification of all the examples that were used in constructing the node's parent. These are passed along in the variable parent.ezamples. 4. If there are no attributes left, but both positive and negative examples, it means that these examples have exactly the same description, but different classifications. This can happen because there is an error or noise in the data; because the domain is nondeter- ministic; or because we can't obscrve an attribute that would distinguish the examples. The best we can do is retum the plurality classification of the remaining examples. The DECISION-TREE-LEARNING algorithm is shown in Figure 18.5. Note that the set of examples is crucial for constructing the tree, but nowhere do the examples appear in the tree itself. A tree consists of just tests on attributes in the interior nodes, values of attributes on the branches, and output values on the leaf nodes. The details of the IMPORTANCE function are given in Section 18.3.4. The output of the learning algorithm on our sample training set is shown in Figure 18.6. The tree is clearly different from the original tree shown in Figure 18.2. One might conclude that the leaming algorithm is not doing a very good job of learming the correct function. This would be the wrong conclusion to draw, however. The learning algorithm looks at the examples, not at the correct function, and in fact, its hypothesis (see Figure 18.6) not only is consistent with all the examples, but is considerably simpler than the original tree! The leaming algorithm has no reason to include tests for Raining and Reservation, because it can classify all the examples without them. It has also detected an interesting and previously unsuspected pattern: the first author will wait for Thai food on weekends. It is also bound to make some mistakes for cases where it has seen no examples. For example, it has never seen a case where the wait is 0-10 minutes but the restaurant is full.
Туре? Patrons? French Italian Thai Burger Nore Some 136 8 10 No Yes Hungry? No Yes 4 12 2 10 (a) (b) Figure 18.4 Splitting the examples by testing on attributes. At each node we show the positive (light boxes) and negative (dark boxes) examples remaining. (a) Splitting on Type brings us no nearer to distinguishing between positive and negative examples (b) Splitting on Patrons does a good job of separating positive and negative examples. After splitting on Patrons, Hungry is a fairly good second test. bination of attribute values, and we retum a default value calculated from the plurality classification of all the examples that were used in constructing the node's parent. These are passed along in the variable parent.ezamples. 4. If there are no attributes left, but both positive and negative examples, it means that these examples have exactly the same description, but different classifications. This can happen because there is an error or noise in the data; because the domain is nondeter- ministic; or because we can't obscrve an attribute that would distinguish the examples. The best we can do is retum the plurality classification of the remaining examples. The DECISION-TREE-LEARNING algorithm is shown in Figure 18.5. Note that the set of examples is crucial for constructing the tree, but nowhere do the examples appear in the tree itself. A tree consists of just tests on attributes in the interior nodes, values of attributes on the branches, and output values on the leaf nodes. The details of the IMPORTANCE function are given in Section 18.3.4. The output of the learning algorithm on our sample training set is shown in Figure 18.6. The tree is clearly different from the original tree shown in Figure 18.2. One might conclude that the leaming algorithm is not doing a very good job of learming the correct function. This would be the wrong conclusion to draw, however. The learning algorithm looks at the examples, not at the correct function, and in fact, its hypothesis (see Figure 18.6) not only is consistent with all the examples, but is considerably simpler than the original tree! The leaming algorithm has no reason to include tests for Raining and Reservation, because it can classify all the examples without them. It has also detected an interesting and previously unsuspected pattern: the first author will wait for Thai food on weekends. It is also bound to make some mistakes for cases where it has seen no examples. For example, it has never seen a case where the wait is 0-10 minutes but the restaurant is full.
Computer Networking: A Top-Down Approach (7th Edition)
7th Edition
ISBN:9780133594140
Author:James Kurose, Keith Ross
Publisher:James Kurose, Keith Ross
Chapter1: Computer Networks And The Internet
Section: Chapter Questions
Problem R1RQ: What is the difference between a host and an end system? List several different types of end...
Related questions
Question

Transcribed Image Text:12
9 10 11
25
11
| Туре?
Patrons?
French
Italian
Thai
Burger
None
Some
Full
1
4 8
3 12
13 E 8
10
2 11
10
Hungry?
No
Yes
No
Yes
4 12
2 10
(a)
(b)
Figure 18.4 Splitting the examples by testing on attributes. At each node we show the
positive (light boxes) and negative (dark boxes) examples remaining. (a) Splitting on Type
brings us no nearer to distinguishing between positive and negative examples. (b) Splitting
on Patrons does a good job of separating positive and negative examples. After splitting on
Patrons, Hungry is a fairly good second test.
bination of attribute values, and we retum a default value calculated from the plurality
classification of all the examples that were used in constructing the node's parent. These
are passed along in the variable parenteramples.
4. If there are no attributes left, but both positive and negative examples, it means that
these examples have exactly the same description, but different classifications. This can
happen because there is an error or noise in the data; because the domain is nondeter-
ministic; or because we can't observe an attribute that would distinguish the examples.
The best we can do is retum the plurality classification of the remaining examples.
The DECISION-TREE-LEARNING algorithm is shown in Figure 18.5. Note that the set of
examples is crucial for constructing the tree, but nowhere do the examples appear in the tree
itself. A tree consists of just tests on attributes in the interior nodes, values of attributes on
the branches, and output values on the leaf nodes. The details of the IMPORTANCE function
are given in Section 18.3.4. The output of the learning algorithm on our sample training
set is shown in Figure 18.6. The tree is clearly different from the original tree shown in
Figure 18.2. One might conclude that the leaming algorithm is not doing a very good job
of learning the correct function. This would be the wrong conclusion to draw, however. The
learning algorithm looks at the examples, not at the correct function, and in fact, its hypothesis
(see Figure 18.6) not only is consistent with all the examples, but is considerably simpler
than the original tree! The learning algorithm has no reason to include tests for Raining and
Reservation, because it can classify all the examples without them. It has also detected an
interesting and previously unsuspected pattern: the first author will wait for Thai food on
weekends. It is also bound to make some mistakes for cases where it has seen no examples.
For example, it has never seen a case where the wait is 0-10 minutes but the restaurant is full.
Expert Solution

This question has been solved!
Explore an expertly crafted, step-by-step solution for a thorough understanding of key concepts.
Step by step
Solved in 2 steps with 1 images

Recommended textbooks for you
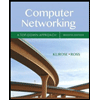
Computer Networking: A Top-Down Approach (7th Edi…
Computer Engineering
ISBN:
9780133594140
Author:
James Kurose, Keith Ross
Publisher:
PEARSON
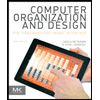
Computer Organization and Design MIPS Edition, Fi…
Computer Engineering
ISBN:
9780124077263
Author:
David A. Patterson, John L. Hennessy
Publisher:
Elsevier Science
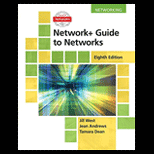
Network+ Guide to Networks (MindTap Course List)
Computer Engineering
ISBN:
9781337569330
Author:
Jill West, Tamara Dean, Jean Andrews
Publisher:
Cengage Learning
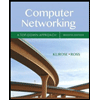
Computer Networking: A Top-Down Approach (7th Edi…
Computer Engineering
ISBN:
9780133594140
Author:
James Kurose, Keith Ross
Publisher:
PEARSON
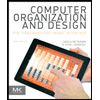
Computer Organization and Design MIPS Edition, Fi…
Computer Engineering
ISBN:
9780124077263
Author:
David A. Patterson, John L. Hennessy
Publisher:
Elsevier Science
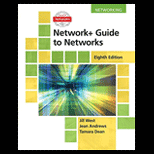
Network+ Guide to Networks (MindTap Course List)
Computer Engineering
ISBN:
9781337569330
Author:
Jill West, Tamara Dean, Jean Andrews
Publisher:
Cengage Learning
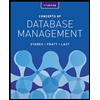
Concepts of Database Management
Computer Engineering
ISBN:
9781337093422
Author:
Joy L. Starks, Philip J. Pratt, Mary Z. Last
Publisher:
Cengage Learning
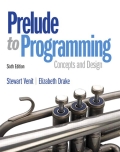
Prelude to Programming
Computer Engineering
ISBN:
9780133750423
Author:
VENIT, Stewart
Publisher:
Pearson Education
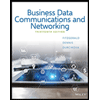
Sc Business Data Communications and Networking, T…
Computer Engineering
ISBN:
9781119368830
Author:
FITZGERALD
Publisher:
WILEY