This problem is inspired by a study of the “gender gap” in earnings in top corporate jobs [Bertrand and Hallock (2001)]. The study compares total compensation among top executives in a large set of U.S. public corporations in the 1990s. (Each year these publicly traded corporations must report total compensation levels for their top five executives.) a. Let Female be an indicator variable that is equal to 1 for females and 0 for males. A regression of the logarithm of
Correlation
Correlation defines a relationship between two independent variables. It tells the degree to which variables move in relation to each other. When two sets of data are related to each other, there is a correlation between them.
Linear Correlation
A correlation is used to determine the relationships between numerical and categorical variables. In other words, it is an indicator of how things are connected to one another. The correlation analysis is the study of how variables are related.
Regression Analysis
Regression analysis is a statistical method in which it estimates the relationship between a dependent variable and one or more independent variable. In simple terms dependent variable is called as outcome variable and independent variable is called as predictors. Regression analysis is one of the methods to find the trends in data. The independent variable used in Regression analysis is named Predictor variable. It offers data of an associated dependent variable regarding a particular outcome.
This problem is inspired by a study of the “gender gap” in earnings in top
corporate jobs [Bertrand and Hallock (2001)]. The study compares total
compensation among top executives in a large set of U.S. public corporations in the 1990s. (Each year these publicly traded corporations must report total compensation levels for their top five executives.)
a. Let Female be an indicator variable that is equal to 1 for females and 0
for males. A regression of the logarithm of earnings onto Female yields
"ln (Earnings)" = 6.48 - 0.44 Female, SER = 2.65.
(0.01) (0.05)
i. The estimated coefficient on Female is -0.44. Explain what this
value means.
ii. The SER is 2.65. Explain what this value means.
iii. Does this regression suggest that female top executives earn less
than top male executives? Explain.
iv. Does this regression suggest that there is gender discrimination?
Explain.
b. Two new variables, the market value of the firm (a measure of firm
size, in millions of dollars) and stock return (a measure of firm
performance, in percentage points), are added to the regression:
''ln(Earnings)" = 3.86 - 0.28 Female + 0.37ln(MarketValue) + 0.004 Return,
(0.03) (0.04) (0.004) (0.003)
n = 46,670, (adjusted) R2= 0.345.
i. The coefficient on ln(MarketValue) is 0.37. Explain what this
value means.
ii. The coefficient on Female is now -0.28. Explain why it has changed from the regression in (a).
c. Are large firms more likely than small firms to have female top executives? Explain.

Trending now
This is a popular solution!
Step by step
Solved in 4 steps

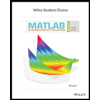
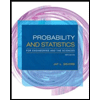
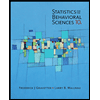
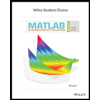
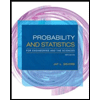
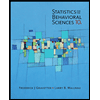
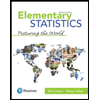
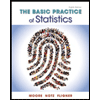
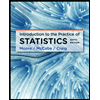