This exercise derives the normalization constant of Beta(a, 3) in the case of integer parameters = 8+1,8=n-s+1 by exploring the connection between • Bayesian inference for a Bernoulli parameter using uniform prior, and • Order statistics of a uniform RV. Let p [0, 1] be the parameter of a Bernoulli (p) distribution (e.g. the probability of Heads for a coin). Suppose we have no prior information about p. In the Bayesian approach, we model our ignorance by considering the parameter p as a uniformly distributed random variable
This exercise derives the normalization constant of Beta(a, 3) in the case of integer parameters = 8+1,8=n-s+1 by exploring the connection between • Bayesian inference for a Bernoulli parameter using uniform prior, and • Order statistics of a uniform RV. Let p [0, 1] be the parameter of a Bernoulli (p) distribution (e.g. the probability of Heads for a coin). Suppose we have no prior information about p. In the Bayesian approach, we model our ignorance by considering the parameter p as a uniformly distributed random variable
A First Course in Probability (10th Edition)
10th Edition
ISBN:9780134753119
Author:Sheldon Ross
Publisher:Sheldon Ross
Chapter1: Combinatorial Analysis
Section: Chapter Questions
Problem 1.1P: a. How many different 7-place license plates are possible if the first 2 places are for letters and...
Related questions
Question
![Exercise 6
This exercise derives the normalization constant of Beta(a, 3) in the case of integer
parameters a = 8+1,ß=n-s+1 by exploring the connection between
Bayesian inference for a Bernoulli parameter using uniform prior, and
• Order statistics of a uniform RV.
Let p = [0, 1] be the parameter of a Bernoulli (p) distribution (e.g. the probability of Heads
for a coin). Suppose we have no prior information about p. In the Bayesian approach,
we model our ignorance by considering the parameter p as a uniformly distributed random
variable
p~ Uniform([0, 1]).
We can then model the observations X₁, X2,, X₁, in the following way: let U₁, U₂, ,‚ Un
be i.i.d. Uniform([0, 1]) that are independent from p, and define
where s = ₁ + ₂ + ..
(ii) Deduce that
X₁ = LUSP: ==
1
(i) Reason that conditioned on the value of p, X₁, X2, X₁ are i.i.d. Bernoulli(p).
Conclude that
and
1
P(X₁ = ₁, ¹, X = np) = (1-P)¹
+ In-
if U; ≤p,
if U₂ > p.
P(X₁ + X₂ +
i=1
=p²(1-p)" *
· + Xu = s[p) = (”) p² (1 − p)” •
P(X₁ + X₂ + -- - + Xm = s) = √" ("* )P^(1 − p)" "dp.
(Hint: for the second equation, use Law of Total Probability.)](/v2/_next/image?url=https%3A%2F%2Fcontent.bartleby.com%2Fqna-images%2Fquestion%2F04625ac1-43ff-4999-b93a-55388fc0c5e2%2F32cf1fbf-ca70-4629-b682-a82b1ce646b4%2F5a0wqdn_processed.png&w=3840&q=75)
Transcribed Image Text:Exercise 6
This exercise derives the normalization constant of Beta(a, 3) in the case of integer
parameters a = 8+1,ß=n-s+1 by exploring the connection between
Bayesian inference for a Bernoulli parameter using uniform prior, and
• Order statistics of a uniform RV.
Let p = [0, 1] be the parameter of a Bernoulli (p) distribution (e.g. the probability of Heads
for a coin). Suppose we have no prior information about p. In the Bayesian approach,
we model our ignorance by considering the parameter p as a uniformly distributed random
variable
p~ Uniform([0, 1]).
We can then model the observations X₁, X2,, X₁, in the following way: let U₁, U₂, ,‚ Un
be i.i.d. Uniform([0, 1]) that are independent from p, and define
where s = ₁ + ₂ + ..
(ii) Deduce that
X₁ = LUSP: ==
1
(i) Reason that conditioned on the value of p, X₁, X2, X₁ are i.i.d. Bernoulli(p).
Conclude that
and
1
P(X₁ = ₁, ¹, X = np) = (1-P)¹
+ In-
if U; ≤p,
if U₂ > p.
P(X₁ + X₂ +
i=1
=p²(1-p)" *
· + Xu = s[p) = (”) p² (1 − p)” •
P(X₁ + X₂ + -- - + Xm = s) = √" ("* )P^(1 − p)" "dp.
(Hint: for the second equation, use Law of Total Probability.)
Expert Solution

Step 1
Given information:
Let p be the parameter of a Bernoulli(p) distribution.
The prior distribution of p is .
Trending now
This is a popular solution!
Step by step
Solved in 3 steps

Recommended textbooks for you
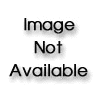
A First Course in Probability (10th Edition)
Probability
ISBN:
9780134753119
Author:
Sheldon Ross
Publisher:
PEARSON
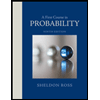
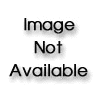
A First Course in Probability (10th Edition)
Probability
ISBN:
9780134753119
Author:
Sheldon Ross
Publisher:
PEARSON
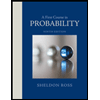