The number of connections to a wrong phone number is often modeled by a Poisson distribution. To estimate the unknown parameter λ of that distribution (the mean number of wrong connections) one observes a sample ₁,..., n of wrong connections on n different days. Assum- ing that σ = x₁ + ... + în > 0, (i)give the likelihood function of the sample; (ii) find the m.l.e. and MLE of X. Hint. (i) If f(x|X) = e, then show that the likelihood function is 2 fn(Xn|X) = en\X° /[[2j!(o= 21+ .tin) j=1 22 (ii) L(X) = lnfn (Xñ |A) = get it. After finding L'(X), show that L'(X) = 0 iff λ = o/n = πn. Now, σ/n is a critical point of L(A). But we can rewrite L' as L'(X) = (n − X) showing that Thus, î= L' is positive for > < xn, L' is equal zero when λ = n, L'is negative when > > n. It proves that în is a local and hence the global maximum point of L and therefore of f(x₂|X). ... = n is the m.l.e. of X and  = Xn is the MLE of X.
The number of connections to a wrong phone number is often modeled by a Poisson distribution. To estimate the unknown parameter λ of that distribution (the mean number of wrong connections) one observes a sample ₁,..., n of wrong connections on n different days. Assum- ing that σ = x₁ + ... + în > 0, (i)give the likelihood function of the sample; (ii) find the m.l.e. and MLE of X. Hint. (i) If f(x|X) = e, then show that the likelihood function is 2 fn(Xn|X) = en\X° /[[2j!(o= 21+ .tin) j=1 22 (ii) L(X) = lnfn (Xñ |A) = get it. After finding L'(X), show that L'(X) = 0 iff λ = o/n = πn. Now, σ/n is a critical point of L(A). But we can rewrite L' as L'(X) = (n − X) showing that Thus, î= L' is positive for > < xn, L' is equal zero when λ = n, L'is negative when > > n. It proves that în is a local and hence the global maximum point of L and therefore of f(x₂|X). ... = n is the m.l.e. of X and  = Xn is the MLE of X.
MATLAB: An Introduction with Applications
6th Edition
ISBN:9781119256830
Author:Amos Gilat
Publisher:Amos Gilat
Chapter1: Starting With Matlab
Section: Chapter Questions
Problem 1P
Related questions
Question
For the image above, a question and process for solving it are included. Please show work and explain how the answer is found for anything in the image that states "explain." The desired answer should be shown at the end of the work please.
![2. The number of connections to a wrong phone number is often modeled by a Poisson distribution. To estimate the unknown parameter \(\lambda\) of that distribution (the mean number of wrong connections) one observes a sample \(x_1, \ldots, x_n\) of wrong connections on \(n\) different days. Assuming that \(\sigma = x_1 + \ldots + x_n > 0\), (i) give the likelihood function of the sample; (ii) find the m.l.e. and MLE of \(\lambda\).
**Hint**. (i) If \(f(x|\lambda) = e^{-\lambda} \frac{\lambda^x}{x!}\), then show that the likelihood function is
\[
f_n(x_n|\lambda) = e^{-n\lambda} \frac{\lambda^\sigma}{\prod_{j=1}^n x_j!}(\sigma = x_1 + \ldots + x_n)
\]
(ii) \(L(\lambda) = \ln f_n(x_n|\lambda) = \text{get it.}\)
After finding \(L'(\lambda)\), show that \(L'(\lambda) = 0\) iff \(\lambda = \sigma/n = \bar{x}_n\).
Now, \(\sigma/n\) is a critical point of \(L(\lambda)\). But we can rewrite \(L'\) as \(L'(\lambda) = \frac{1}{\lambda_n}(\bar{x}_n - \lambda)\) showing that
\[
\begin{align*}
L' \text{ is positive for } \lambda < \bar{x}_n, \\
L' \text{ is equal to zero when } \lambda = \bar{x}_n, \\
L' \text{ is negative when } \lambda > \bar{x}_n.
\end{align*}
\]
It proves that \(\bar{x}_n\) is a local and hence the global maximum point of \(L\) and therefore of \(f_n(x_n|\lambda)\).
Thus, \(\hat{\lambda} = \bar{x}_n\) is the m.l.e. of \(\lambda\) and
\[
\hat{\Lambda} = X_n
\]
is the MLE of \(\lambda\).](/v2/_next/image?url=https%3A%2F%2Fcontent.bartleby.com%2Fqna-images%2Fquestion%2Fdb82eee3-c597-43b7-b98f-cffc18fbca72%2F64eae050-45c6-4437-89f4-80b8ed083e71%2Fxisyy8a_processed.png&w=3840&q=75)
Transcribed Image Text:2. The number of connections to a wrong phone number is often modeled by a Poisson distribution. To estimate the unknown parameter \(\lambda\) of that distribution (the mean number of wrong connections) one observes a sample \(x_1, \ldots, x_n\) of wrong connections on \(n\) different days. Assuming that \(\sigma = x_1 + \ldots + x_n > 0\), (i) give the likelihood function of the sample; (ii) find the m.l.e. and MLE of \(\lambda\).
**Hint**. (i) If \(f(x|\lambda) = e^{-\lambda} \frac{\lambda^x}{x!}\), then show that the likelihood function is
\[
f_n(x_n|\lambda) = e^{-n\lambda} \frac{\lambda^\sigma}{\prod_{j=1}^n x_j!}(\sigma = x_1 + \ldots + x_n)
\]
(ii) \(L(\lambda) = \ln f_n(x_n|\lambda) = \text{get it.}\)
After finding \(L'(\lambda)\), show that \(L'(\lambda) = 0\) iff \(\lambda = \sigma/n = \bar{x}_n\).
Now, \(\sigma/n\) is a critical point of \(L(\lambda)\). But we can rewrite \(L'\) as \(L'(\lambda) = \frac{1}{\lambda_n}(\bar{x}_n - \lambda)\) showing that
\[
\begin{align*}
L' \text{ is positive for } \lambda < \bar{x}_n, \\
L' \text{ is equal to zero when } \lambda = \bar{x}_n, \\
L' \text{ is negative when } \lambda > \bar{x}_n.
\end{align*}
\]
It proves that \(\bar{x}_n\) is a local and hence the global maximum point of \(L\) and therefore of \(f_n(x_n|\lambda)\).
Thus, \(\hat{\lambda} = \bar{x}_n\) is the m.l.e. of \(\lambda\) and
\[
\hat{\Lambda} = X_n
\]
is the MLE of \(\lambda\).
Expert Solution

This question has been solved!
Explore an expertly crafted, step-by-step solution for a thorough understanding of key concepts.
Step by step
Solved in 3 steps with 3 images

Recommended textbooks for you
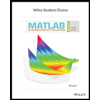
MATLAB: An Introduction with Applications
Statistics
ISBN:
9781119256830
Author:
Amos Gilat
Publisher:
John Wiley & Sons Inc
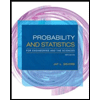
Probability and Statistics for Engineering and th…
Statistics
ISBN:
9781305251809
Author:
Jay L. Devore
Publisher:
Cengage Learning
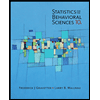
Statistics for The Behavioral Sciences (MindTap C…
Statistics
ISBN:
9781305504912
Author:
Frederick J Gravetter, Larry B. Wallnau
Publisher:
Cengage Learning
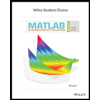
MATLAB: An Introduction with Applications
Statistics
ISBN:
9781119256830
Author:
Amos Gilat
Publisher:
John Wiley & Sons Inc
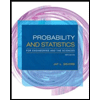
Probability and Statistics for Engineering and th…
Statistics
ISBN:
9781305251809
Author:
Jay L. Devore
Publisher:
Cengage Learning
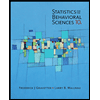
Statistics for The Behavioral Sciences (MindTap C…
Statistics
ISBN:
9781305504912
Author:
Frederick J Gravetter, Larry B. Wallnau
Publisher:
Cengage Learning
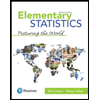
Elementary Statistics: Picturing the World (7th E…
Statistics
ISBN:
9780134683416
Author:
Ron Larson, Betsy Farber
Publisher:
PEARSON
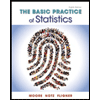
The Basic Practice of Statistics
Statistics
ISBN:
9781319042578
Author:
David S. Moore, William I. Notz, Michael A. Fligner
Publisher:
W. H. Freeman
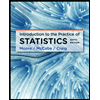
Introduction to the Practice of Statistics
Statistics
ISBN:
9781319013387
Author:
David S. Moore, George P. McCabe, Bruce A. Craig
Publisher:
W. H. Freeman