The graph below shows different function growth complexities (Big-O orders). Label each line with the appropriate Big-O growth function from the table on the chart.
The graph below shows different function growth complexities (Big-O orders). Label each line with the appropriate Big-O growth function from the table on the chart.
Database System Concepts
7th Edition
ISBN:9780078022159
Author:Abraham Silberschatz Professor, Henry F. Korth, S. Sudarshan
Publisher:Abraham Silberschatz Professor, Henry F. Korth, S. Sudarshan
Chapter1: Introduction
Section: Chapter Questions
Problem 1PE
Related questions
Question
The graph below shows different function growth complexities (Big-O
orders). Label each line with the appropriate Big-O growth function from the
table on the chart.

Transcribed Image Text:The image is a graph illustrating the growth of different computational complexities based on input size, \( N \). The graph plots various Big O notations, demonstrating their relative running times as \( N \) increases. Each line represents a different complexity class:
1. **O(1):** A constant time complexity, shown as a horizontal line near the x-axis, indicating it does not change regardless of input size.
2. **O(log N):** This curve grows slowly and represents algorithms whose time complexity grows logarithmically. It's depicted with a very gradual increase.
3. **O(N):** A linear time complexity, shown as a diagonal line. The running time increases linearly with the size of the input.
4. **O(N log N):** Typically associated with more efficient sorting algorithms, this line rises more steeply than O(N) but less than quadratic time complexities.
5. **O(N^2):** A quadratic time complexity, depicted as a steep curve, showing how the running time increases exponentially with the size of the input.
6. **O(N!):** Representing factorial time complexity, this line shoots up sharply, indicating an extremely rapid increase in running time, typical for algorithms with very poor scalability.
The graph provides a visual representation of how different algorithmic complexities affect the running time relative to the input size, helping learners understand the impact of algorithm efficiency on performance.
Expert Solution

This question has been solved!
Explore an expertly crafted, step-by-step solution for a thorough understanding of key concepts.
This is a popular solution!
Trending now
This is a popular solution!
Step by step
Solved in 2 steps with 2 images

Knowledge Booster
Learn more about
Need a deep-dive on the concept behind this application? Look no further. Learn more about this topic, computer-science and related others by exploring similar questions and additional content below.Recommended textbooks for you
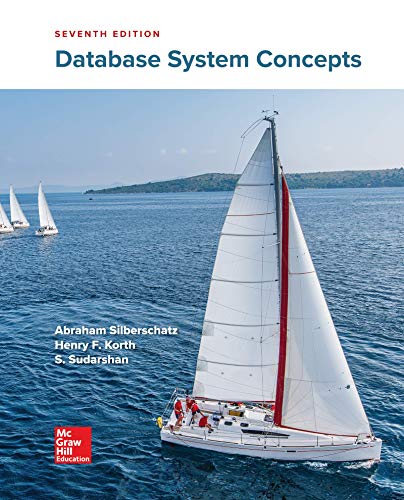
Database System Concepts
Computer Science
ISBN:
9780078022159
Author:
Abraham Silberschatz Professor, Henry F. Korth, S. Sudarshan
Publisher:
McGraw-Hill Education
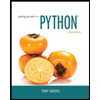
Starting Out with Python (4th Edition)
Computer Science
ISBN:
9780134444321
Author:
Tony Gaddis
Publisher:
PEARSON
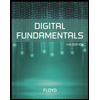
Digital Fundamentals (11th Edition)
Computer Science
ISBN:
9780132737968
Author:
Thomas L. Floyd
Publisher:
PEARSON
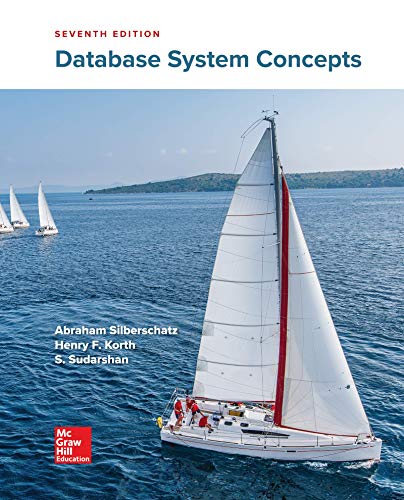
Database System Concepts
Computer Science
ISBN:
9780078022159
Author:
Abraham Silberschatz Professor, Henry F. Korth, S. Sudarshan
Publisher:
McGraw-Hill Education
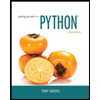
Starting Out with Python (4th Edition)
Computer Science
ISBN:
9780134444321
Author:
Tony Gaddis
Publisher:
PEARSON
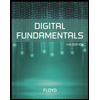
Digital Fundamentals (11th Edition)
Computer Science
ISBN:
9780132737968
Author:
Thomas L. Floyd
Publisher:
PEARSON
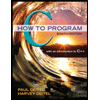
C How to Program (8th Edition)
Computer Science
ISBN:
9780133976892
Author:
Paul J. Deitel, Harvey Deitel
Publisher:
PEARSON
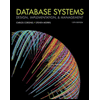
Database Systems: Design, Implementation, & Manag…
Computer Science
ISBN:
9781337627900
Author:
Carlos Coronel, Steven Morris
Publisher:
Cengage Learning
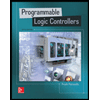
Programmable Logic Controllers
Computer Science
ISBN:
9780073373843
Author:
Frank D. Petruzella
Publisher:
McGraw-Hill Education