The following data was collected to explore how the number of square feet in a house, the number of bedrooms, and the age of the house affect the selling price of the house. The dependent variable is the selling price of the house, the first independent variable (x) is the square footage, the second independent variable (x2) is the number of bedrooms, and the third independent variable (x3) is the age of the house. Effects on Selling Price of Houses Square Feet Number of Bedrooms Age Selling Price 2848 4 6 242100 1270 5 7 113600 1825 4 281700 2235 199100 2072 4 307500 2197 4 14 278800 2184 5. 275300 Prev 1764 4 7 107200 2276 4 14 103000 Copy Data Step 1 of 2: Find the p-value for the regression equation that fits the given data. Round your answer to four decimal places.
The following data was collected to explore how the number of square feet in a house, the number of bedrooms, and the age of the house affect the selling price of the house. The dependent variable is the selling price of the house, the first independent variable (x) is the square footage, the second independent variable (x2) is the number of bedrooms, and the third independent variable (x3) is the age of the house. Effects on Selling Price of Houses Square Feet Number of Bedrooms Age Selling Price 2848 4 6 242100 1270 5 7 113600 1825 4 281700 2235 199100 2072 4 307500 2197 4 14 278800 2184 5. 275300 Prev 1764 4 7 107200 2276 4 14 103000 Copy Data Step 1 of 2: Find the p-value for the regression equation that fits the given data. Round your answer to four decimal places.
MATLAB: An Introduction with Applications
6th Edition
ISBN:9781119256830
Author:Amos Gilat
Publisher:Amos Gilat
Chapter1: Starting With Matlab
Section: Chapter Questions
Problem 1P
Related questions
Question
![### Linear Regression Analysis on Housing Data
The table below presents data on house characteristics and their selling prices. The goal is to determine if there is a statistically significant linear relationship between the independent variables (Square Feet, Number of Bedrooms, Age) and the dependent variable (Selling Price) at the 0.05 level of significance. If significant, we will identify the multiple regression equation that best fits the data.
| Square Feet | Number of Bedrooms | Age | Selling Price |
|-------------|---------------------|-----|---------------|
| 2848 | 4 | 6 | 242100 |
| 1270 | 4 | 7 | 113600 |
| 1825 | 4 | 8 | 281700 |
| 2235 | 5 | 5 | 199100 |
| 2072 | 4 | 2 | 307500 |
| 2197 | 4 | 14 | 278800 |
| 2184 | 4 | 5 | 275300 |
| 1764 | 4 | 7 | 107200 |
| 2276 | 4 | 14 | 103000 |
#### Step 2 of 2
Determine whether there is a statistically significant linear relationship at the 0.05 significance level.
If the relationship is confirmed as statistically significant, you will need to compute the multiple regression equation that best fits the data, rounding the answers to three decimal places. Otherwise, indicate that there is not enough evidence for statistical significance.
Use the regression equation form:
\[ \hat{y} = b_0 + b_1 x_1 + b_2 x_2 + b_3 x_3 \]
- \( b_0 \): Intercept
- \( b_1 \), \( b_2 \), \( b_3 \): Coefficients for Square Feet, Number of Bedrooms, and Age respectively
To proceed, select the appropriate checkbox if there is enough evidence, otherwise note that there isn't.](/v2/_next/image?url=https%3A%2F%2Fcontent.bartleby.com%2Fqna-images%2Fquestion%2F0effa018-95f4-43af-8a60-b2be877a4826%2F9f2bf732-f729-45ee-8a3e-8123428f78d7%2Fufb45j_processed.jpeg&w=3840&q=75)
Transcribed Image Text:### Linear Regression Analysis on Housing Data
The table below presents data on house characteristics and their selling prices. The goal is to determine if there is a statistically significant linear relationship between the independent variables (Square Feet, Number of Bedrooms, Age) and the dependent variable (Selling Price) at the 0.05 level of significance. If significant, we will identify the multiple regression equation that best fits the data.
| Square Feet | Number of Bedrooms | Age | Selling Price |
|-------------|---------------------|-----|---------------|
| 2848 | 4 | 6 | 242100 |
| 1270 | 4 | 7 | 113600 |
| 1825 | 4 | 8 | 281700 |
| 2235 | 5 | 5 | 199100 |
| 2072 | 4 | 2 | 307500 |
| 2197 | 4 | 14 | 278800 |
| 2184 | 4 | 5 | 275300 |
| 1764 | 4 | 7 | 107200 |
| 2276 | 4 | 14 | 103000 |
#### Step 2 of 2
Determine whether there is a statistically significant linear relationship at the 0.05 significance level.
If the relationship is confirmed as statistically significant, you will need to compute the multiple regression equation that best fits the data, rounding the answers to three decimal places. Otherwise, indicate that there is not enough evidence for statistical significance.
Use the regression equation form:
\[ \hat{y} = b_0 + b_1 x_1 + b_2 x_2 + b_3 x_3 \]
- \( b_0 \): Intercept
- \( b_1 \), \( b_2 \), \( b_3 \): Coefficients for Square Feet, Number of Bedrooms, and Age respectively
To proceed, select the appropriate checkbox if there is enough evidence, otherwise note that there isn't.

Transcribed Image Text:### Exploring the Factors Affecting the Selling Price of Houses
The following data was collected to explore how the number of square feet in a house, the number of bedrooms, and the age of the house affect the selling price of the house. The dependent variable is the selling price of the house, the first independent variable (\(x_1\)) is the square footage, the second independent variable (\(x_2\)) is the number of bedrooms, and the third independent variable (\(x_3\)) is the age of the house.
#### Effects on Selling Price of Houses
| Square Feet | Number of Bedrooms | Age | Selling Price |
|-------------|---------------------|-----|---------------|
| 2848 | 4 | 6 | $242,100 |
| 1270 | 5 | 7 | $113,600 |
| 1825 | 4 | 8 | $281,700 |
| 2235 | 5 | 5 | $199,100 |
| 2072 | 4 | 2 | $307,500 |
| 2197 | 4 | 14 | $278,800 |
| 2184 | 4 | 5 | $275,300 |
| 1764 | 4 | 7 | $107,200 |
| 2276 | 4 | 14 | $103,000 |
**Instructions:**
Step 1 of 2: Find the \(p\)-value for the regression equation that fits the given data. Round your answer to four decimal places.
Expert Solution

This question has been solved!
Explore an expertly crafted, step-by-step solution for a thorough understanding of key concepts.
This is a popular solution!
Trending now
This is a popular solution!
Step by step
Solved in 3 steps with 2 images

Recommended textbooks for you
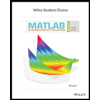
MATLAB: An Introduction with Applications
Statistics
ISBN:
9781119256830
Author:
Amos Gilat
Publisher:
John Wiley & Sons Inc
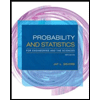
Probability and Statistics for Engineering and th…
Statistics
ISBN:
9781305251809
Author:
Jay L. Devore
Publisher:
Cengage Learning
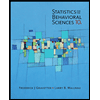
Statistics for The Behavioral Sciences (MindTap C…
Statistics
ISBN:
9781305504912
Author:
Frederick J Gravetter, Larry B. Wallnau
Publisher:
Cengage Learning
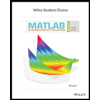
MATLAB: An Introduction with Applications
Statistics
ISBN:
9781119256830
Author:
Amos Gilat
Publisher:
John Wiley & Sons Inc
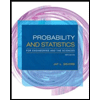
Probability and Statistics for Engineering and th…
Statistics
ISBN:
9781305251809
Author:
Jay L. Devore
Publisher:
Cengage Learning
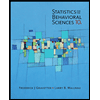
Statistics for The Behavioral Sciences (MindTap C…
Statistics
ISBN:
9781305504912
Author:
Frederick J Gravetter, Larry B. Wallnau
Publisher:
Cengage Learning
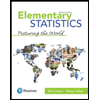
Elementary Statistics: Picturing the World (7th E…
Statistics
ISBN:
9780134683416
Author:
Ron Larson, Betsy Farber
Publisher:
PEARSON
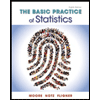
The Basic Practice of Statistics
Statistics
ISBN:
9781319042578
Author:
David S. Moore, William I. Notz, Michael A. Fligner
Publisher:
W. H. Freeman
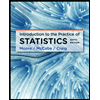
Introduction to the Practice of Statistics
Statistics
ISBN:
9781319013387
Author:
David S. Moore, George P. McCabe, Bruce A. Craig
Publisher:
W. H. Freeman