The director of the MBA program at Salterdine University wants to develop a procedure to determine which applicants to admit to the MBA program. The director believes that an applicant’s undergraduate grade point average (GPA) and score on the GMAT exam are helpful in predicting which applicants will be good students. To assist in this endeavor, the director asked a committee of faculty members to classify 70 of the recent students in the MBA program into two groups: (1) good students and (2) weak students. The file MBAStudents.xlsm summarizes these ratings, along with the GPA and GMAT scores for the 70 students. What are the coordinates of the centroids for the good students and the weak students? Use XLMiner’s standard data partition command to partition the data into a training set (with 60% of the observations) and validation set (with 40% of the observations) using the default seed of 12345. Use discriminant analysis to create a classifier for this data. How accurate is this procedure on the training and validation data sets? Suppose that the MBA director received applications for admission to the MBA program from the following individuals. According to your recommended classifier, which of these individuals do you expect to be good students and which do you expect to be weak? Name GPA GMAT Mike Dimoupolous 3.02 450 Scott Frazier 2.97 587 Paula Curry 3.95 551 Terry Freeman 2.45 484 Dana Simmons 3.
The director of the MBA program at Salterdine University wants to develop a procedure to determine which applicants to admit to the MBA program. The director believes that an applicant’s undergraduate grade point average (GPA) and score on the GMAT exam are helpful in predicting which applicants will be good students. To assist in this endeavor, the director asked a committee of faculty members to classify 70 of the recent students in the MBA program into two groups: (1) good students and (2) weak students. The file MBAStudents.xlsm summarizes these ratings, along with the GPA and GMAT scores for the 70 students.
- What are the coordinates of the centroids for the good students and the weak students?
- Use XLMiner’s standard data partition command to partition the data into a training set (with 60% of the observations) and validation set (with 40% of the observations) using the default seed of 12345.
- Use discriminant analysis to create a classifier for this data. How accurate is this procedure on the training and validation data sets?
- Suppose that the MBA director received applications for admission to the MBA program from the following individuals. According to your recommended classifier, which of these individuals do you expect to be good students and which do you expect to be weak?
Name GPA GMAT
Mike Dimoupolous 3.02 450
Scott Frazier 2.97 587
Paula Curry 3.95 551
Terry Freeman 2.45 484
Dana Simmons 3.26 524
DATA
Student | Rating | GPA | GMAT |
1 | 1 | 2.96 | 671 |
2 | 1 | 3.14 | 548 |
3 | 1 | 3.22 | 557 |
4 | 1 | 3.29 | 602 |
5 | 1 | 3.69 | 580 |
6 | 1 | 3.46 | 768 |
7 | 1 | 3.03 | 701 |
8 | 1 | 3.19 | 738 |
9 | 1 | 3.63 | 522 |
10 | 1 | 3.59 | 663 |
11 | 1 | 2.86 | 569 |
12 | 1 | 2.85 | 571 |
13 | 1 | 3.14 | 494 |
14 | 1 | 3.28 | 446 |
15 | 1 | 2.89 | 522 |
16 | 1 | 3.37 | 590 |
17 | 1 | 2.53 | 564 |
18 | 1 | 3.24 | 612 |
19 | 1 | 3.04 | 596 |
20 | 1 | 3.44 | 685 |
21 | 1 | 3.34 | 583 |
22 | 1 | 3.52 | 574 |
23 | 1 | 3.84 | 658 |
24 | 1 | 3.07 | 618 |
25 | 1 | 2.83 | 541 |
26 | 1 | 2.26 | 583 |
27 | 1 | 2.75 | 573 |
28 | 1 | 3.55 | 602 |
29 | 1 | 5.76 | 622 |
30 | 1 | 3.48 | 655 |
31 | 1 | 3.36 | 588 |
32 | 1 | 3.12 | 534 |
33 | 1 | 3.22 | 580 |
34 | 1 | 3.28 | 602 |
35 | 1 | 3.26 | 626 |
36 | 2 | 3.33 | 588 |
37 | 2 | 3.01 | 555 |
38 | 2 | 3.57 | 541 |
39 | 2 | 2.8 | 566 |
40 | 2 | 3.06 | 569 |
41 | 2 | 1.98 | 562 |
42 | 2 | 2.69 | 610 |
43 | 2 | 3.33 | 548 |
44 | 2 | 2.47 | 599 |
45 | 2 | 1.85 | 464 |
46 | 2 | 2.47 | 478 |
47 | 2 | 2.38 | 648 |
48 | 2 | 2.36 | 548 |
49 | 2 | 2.73 | 477 |
50 | 2 | 2.56 | 490 |
51 | 2 | 2.3 | 410 |
53 | 2 | 3.41 | 434 |
54 | 2 | 2.56 | 563 |
55 | 2 | 2.25 | 466 |
56 | 2 | 3.15 | 388 |
57 | 2 | 3.5 | 477 |
58 | 2 | 2.89 | 560 |
59 | 2 | 2.8 | 519 |
60 | 2 | 3.13 | 491 |
61 | 2 | 2.54 | 521 |
62 | 2 | 2.43 | 500 |
63 | 2 | 2.2 | 549 |
64 | 2 | 2.36 | 606 |
65 | 2 | 2.57 | 617 |
66 | 2 | 2.35 | 481 |
67 | 2 | 2.72 | 487 |
68 | 2 | 2.51 | 533 |
69 | 2 | 2.36 | 474 |
70 | 2 | 2.19 | 557 |

Trending now
This is a popular solution!
Step by step
Solved in 6 steps with 17 images

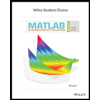
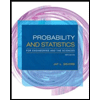
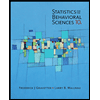
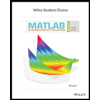
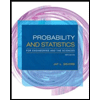
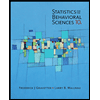
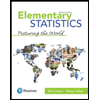
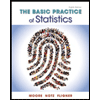
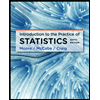