The basic convolutional block in GoogLeNet is called an Inception block, likely named due to a quote from the movie Inception ("We need to go deeper"), which launched a viral meme. Concatenation 3x3 Conv, pad 1 5x 5 Conv, pad 2 1x 1 Conv 1x 1 Conv 1x 1 Conv 1 x 1 Conv 3x 3 MaxPool, pad 1 Input Fig. 7.4.1: Structure of the Inception block. As depicted in Fig. 7.4.1, the inception block consists of four parallel paths. The first three paths use convolutional layers with window sizes of 1 x 1, 3 x 3, and 5 x 5 to extract information from different spatial sizes. The middle two paths perform a 1 x 1 convolution on the input to reduce the number of channels, reducing the model's complexity. The fourth path uses a 3 x 3 maximum pooling layer, followed by a 1 x 1 convolutional layer to change the number of channels. The four paths all use appropriate padding to give the input and output the same height and width. Finally, the outputs along each path are concatenated along the channel dimension and comprise the block's output. The commonly-tuned hyperparameters of the Inception block are the number of output channels per layer.
The basic convolutional block in GoogLeNet is called an Inception block, likely named due to a quote from the movie Inception ("We need to go deeper"), which launched a viral meme. Concatenation 3x3 Conv, pad 1 5x 5 Conv, pad 2 1x 1 Conv 1x 1 Conv 1x 1 Conv 1 x 1 Conv 3x 3 MaxPool, pad 1 Input Fig. 7.4.1: Structure of the Inception block. As depicted in Fig. 7.4.1, the inception block consists of four parallel paths. The first three paths use convolutional layers with window sizes of 1 x 1, 3 x 3, and 5 x 5 to extract information from different spatial sizes. The middle two paths perform a 1 x 1 convolution on the input to reduce the number of channels, reducing the model's complexity. The fourth path uses a 3 x 3 maximum pooling layer, followed by a 1 x 1 convolutional layer to change the number of channels. The four paths all use appropriate padding to give the input and output the same height and width. Finally, the outputs along each path are concatenated along the channel dimension and comprise the block's output. The commonly-tuned hyperparameters of the Inception block are the number of output channels per layer.
Computer Networking: A Top-Down Approach (7th Edition)
7th Edition
ISBN:9780133594140
Author:James Kurose, Keith Ross
Publisher:James Kurose, Keith Ross
Chapter1: Computer Networks And The Internet
Section: Chapter Questions
Problem R1RQ: What is the difference between a host and an end system? List several different types of end...
Related questions
Question
Matlab

Transcribed Image Text:The basic convolutional block in GoogLeNet is called an Inception block, likely named due to a quote
from the movie Inception ("We need to go deeper"), which launched a viral meme.
Concatenation
3x 3 Conv, pad 1
5x 5 Conv, pad 2
1x 1 Conv
1x 1 Conv
1x 1 Conv
1 x 1 Conv
3х 3 МаxРоo!, pad 1
Input
Fig. 7.4.1: Structure of the Inception block.
As depicted in Fig. 7.4.1, the inception block consists of four parallel paths. The first three paths
use convolutional layers with window sizes of 1 x 1, 3 x 3, and 5 x 5 to extract information from
different spatial sizes. The middle two paths perform a 1 x 1 convolution on the input to reduce
the number of channels, reducing the model's complexity. The fourth path uses a 3 x 3 maximum
pooling layer, followed by a 1 x 1 convolutional layer to change the number of channels. The
four paths all use appropriate padding to give the input and output the same height and width.
Finally, the outputs along each path are concatenated along the channel dimension and comprise
the block's output. The commonly-tuned hyperparameters of the Inception block are the number
of output channels per layer.
Expert Solution

This question has been solved!
Explore an expertly crafted, step-by-step solution for a thorough understanding of key concepts.
This is a popular solution!
Trending now
This is a popular solution!
Step by step
Solved in 2 steps with 1 images

Recommended textbooks for you
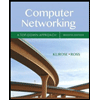
Computer Networking: A Top-Down Approach (7th Edi…
Computer Engineering
ISBN:
9780133594140
Author:
James Kurose, Keith Ross
Publisher:
PEARSON
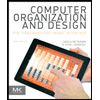
Computer Organization and Design MIPS Edition, Fi…
Computer Engineering
ISBN:
9780124077263
Author:
David A. Patterson, John L. Hennessy
Publisher:
Elsevier Science
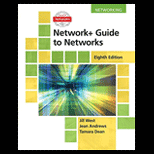
Network+ Guide to Networks (MindTap Course List)
Computer Engineering
ISBN:
9781337569330
Author:
Jill West, Tamara Dean, Jean Andrews
Publisher:
Cengage Learning
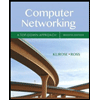
Computer Networking: A Top-Down Approach (7th Edi…
Computer Engineering
ISBN:
9780133594140
Author:
James Kurose, Keith Ross
Publisher:
PEARSON
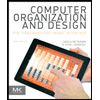
Computer Organization and Design MIPS Edition, Fi…
Computer Engineering
ISBN:
9780124077263
Author:
David A. Patterson, John L. Hennessy
Publisher:
Elsevier Science
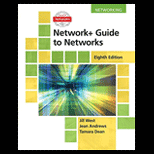
Network+ Guide to Networks (MindTap Course List)
Computer Engineering
ISBN:
9781337569330
Author:
Jill West, Tamara Dean, Jean Andrews
Publisher:
Cengage Learning
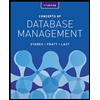
Concepts of Database Management
Computer Engineering
ISBN:
9781337093422
Author:
Joy L. Starks, Philip J. Pratt, Mary Z. Last
Publisher:
Cengage Learning
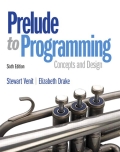
Prelude to Programming
Computer Engineering
ISBN:
9780133750423
Author:
VENIT, Stewart
Publisher:
Pearson Education
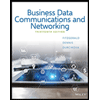
Sc Business Data Communications and Networking, T…
Computer Engineering
ISBN:
9781119368830
Author:
FITZGERALD
Publisher:
WILEY