Task 1: Feed Forward Neural Network Here is a problem consisting of inputs P and targets T to be solved with a network. P=[0 1 2 3 4 5 6 7 8 9 10]; T = [0 1 2 3 4 3 2 1 2 3 4]; Here a network is created with one hidden layer of five neurons. net = newff(P.T,5); The network is simulated and its output plotted against the targets. = sim(net,P); plot(P.T.P.Y,'o') The network is trained for 50 epochs. Again the network's output is plotted. net.trainParam.epochs = 50; met = train(net,P,T); Incim/not Pl
Task 1: Feed Forward Neural Network Here is a problem consisting of inputs P and targets T to be solved with a network. P=[0 1 2 3 4 5 6 7 8 9 10]; T = [0 1 2 3 4 3 2 1 2 3 4]; Here a network is created with one hidden layer of five neurons. net = newff(P.T,5); The network is simulated and its output plotted against the targets. = sim(net,P); plot(P.T.P.Y,'o') The network is trained for 50 epochs. Again the network's output is plotted. net.trainParam.epochs = 50; met = train(net,P,T); Incim/not Pl
Database System Concepts
7th Edition
ISBN:9780078022159
Author:Abraham Silberschatz Professor, Henry F. Korth, S. Sudarshan
Publisher:Abraham Silberschatz Professor, Henry F. Korth, S. Sudarshan
Chapter1: Introduction
Section: Chapter Questions
Problem 1PE
Related questions
Question
![Task 1: Feed Forward Neural Network
Here is a problem consisting of inputs P and targets T to be solved with a network.
P = [012345678910];
T = [01234321 2 3 4];
Here a network is created with one hidden layer of five neurons.
net = newff(P,T,5);
The network is simulated and its output plotted against the targets.
Y = sim(net,P);
plot(P,T,P,Y,'o')
The network is trained for 50 epochs. Again the network's output is plotted.
net.trainParam.epochs = 50;
net = train(net,P,T);
Y = sim(net,P);
plot(P,T,P,Y,'o')
Change the training epochs to 100 and 1000, and plot again. Copy the plotted figures (4
figures) and prepare a one-page report including your comments under each figure.
Task 2: Repeat task 1 for
a) simplefit_dataset (use the command load simplefit_dataset)
b) simplecluster dataset (use the command load simplecluster_dataset)
Using 50 and 1000 epochs in the training, each with 5 and 20 hidden layers and report the
figures (8 figures in total) obtained with your comments.
▪](/v2/_next/image?url=https%3A%2F%2Fcontent.bartleby.com%2Fqna-images%2Fquestion%2F385c6f29-1f1e-40e3-b8f8-8d3f84f564c8%2F9b634378-dea4-4b1b-a218-b37417c919e6%2Fgbpizvt_processed.jpeg&w=3840&q=75)
Transcribed Image Text:Task 1: Feed Forward Neural Network
Here is a problem consisting of inputs P and targets T to be solved with a network.
P = [012345678910];
T = [01234321 2 3 4];
Here a network is created with one hidden layer of five neurons.
net = newff(P,T,5);
The network is simulated and its output plotted against the targets.
Y = sim(net,P);
plot(P,T,P,Y,'o')
The network is trained for 50 epochs. Again the network's output is plotted.
net.trainParam.epochs = 50;
net = train(net,P,T);
Y = sim(net,P);
plot(P,T,P,Y,'o')
Change the training epochs to 100 and 1000, and plot again. Copy the plotted figures (4
figures) and prepare a one-page report including your comments under each figure.
Task 2: Repeat task 1 for
a) simplefit_dataset (use the command load simplefit_dataset)
b) simplecluster dataset (use the command load simplecluster_dataset)
Using 50 and 1000 epochs in the training, each with 5 and 20 hidden layers and report the
figures (8 figures in total) obtained with your comments.
▪
![Description
newff(P,T,[S1 S2...S(N-1)],{TF1 TF2...TFNI), BTF,BLF, PF,IPF,OPF,DDF) takes several arguments
PR x Q1 matrix of Q1 sample R-element input vectorsTSN x Q2 matrix of Q2 sample SN-
element target vectorsSiSize of ith layer, for N-1 layers, default = [].
(Output layer size SN is determined from T.)TFiTransfer function of ith layer. (Default=
'tansig' for
hidden layers and 'purelin' for output layer.)BTFBackpropagation network training function
(default = 'trainlm')BLFBackpropagation weight/bias learning function (default=
'learngdm')PFPerformance function. (Default = 'mse')IPFRow cell array of input processing
functions. (Default = {'fixunknowns','removeconstantrows','mapminmax'})OPFRow cell array
of output processing functions. (Default = {'removeconstantrows','mapminmax'}) DDFData
divison function (default = 'dividerand'),
and returns an N-layer feed-forward backpropagation network.
The transfer functions TFi can be any differentiable transfer function such as tansig, logsig,
or purelin.
The training function BTF can be any of the backpropagation training functions such as
trainim, trainbfg, trainrp, traingd, etc.](/v2/_next/image?url=https%3A%2F%2Fcontent.bartleby.com%2Fqna-images%2Fquestion%2F385c6f29-1f1e-40e3-b8f8-8d3f84f564c8%2F9b634378-dea4-4b1b-a218-b37417c919e6%2Foj5pd15_processed.jpeg&w=3840&q=75)
Transcribed Image Text:Description
newff(P,T,[S1 S2...S(N-1)],{TF1 TF2...TFNI), BTF,BLF, PF,IPF,OPF,DDF) takes several arguments
PR x Q1 matrix of Q1 sample R-element input vectorsTSN x Q2 matrix of Q2 sample SN-
element target vectorsSiSize of ith layer, for N-1 layers, default = [].
(Output layer size SN is determined from T.)TFiTransfer function of ith layer. (Default=
'tansig' for
hidden layers and 'purelin' for output layer.)BTFBackpropagation network training function
(default = 'trainlm')BLFBackpropagation weight/bias learning function (default=
'learngdm')PFPerformance function. (Default = 'mse')IPFRow cell array of input processing
functions. (Default = {'fixunknowns','removeconstantrows','mapminmax'})OPFRow cell array
of output processing functions. (Default = {'removeconstantrows','mapminmax'}) DDFData
divison function (default = 'dividerand'),
and returns an N-layer feed-forward backpropagation network.
The transfer functions TFi can be any differentiable transfer function such as tansig, logsig,
or purelin.
The training function BTF can be any of the backpropagation training functions such as
trainim, trainbfg, trainrp, traingd, etc.
Expert Solution

This question has been solved!
Explore an expertly crafted, step-by-step solution for a thorough understanding of key concepts.
Step by step
Solved in 4 steps with 1 images

Knowledge Booster
Learn more about
Need a deep-dive on the concept behind this application? Look no further. Learn more about this topic, computer-science and related others by exploring similar questions and additional content below.Recommended textbooks for you
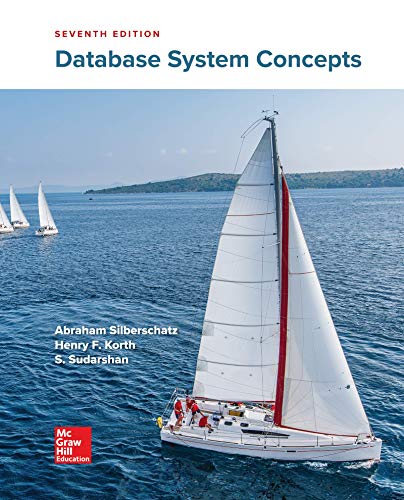
Database System Concepts
Computer Science
ISBN:
9780078022159
Author:
Abraham Silberschatz Professor, Henry F. Korth, S. Sudarshan
Publisher:
McGraw-Hill Education
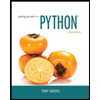
Starting Out with Python (4th Edition)
Computer Science
ISBN:
9780134444321
Author:
Tony Gaddis
Publisher:
PEARSON
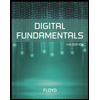
Digital Fundamentals (11th Edition)
Computer Science
ISBN:
9780132737968
Author:
Thomas L. Floyd
Publisher:
PEARSON
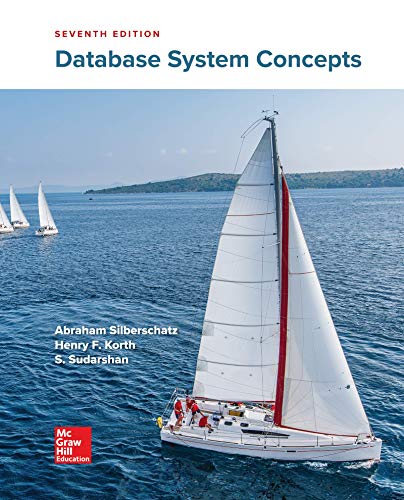
Database System Concepts
Computer Science
ISBN:
9780078022159
Author:
Abraham Silberschatz Professor, Henry F. Korth, S. Sudarshan
Publisher:
McGraw-Hill Education
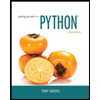
Starting Out with Python (4th Edition)
Computer Science
ISBN:
9780134444321
Author:
Tony Gaddis
Publisher:
PEARSON
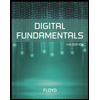
Digital Fundamentals (11th Edition)
Computer Science
ISBN:
9780132737968
Author:
Thomas L. Floyd
Publisher:
PEARSON
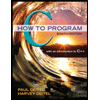
C How to Program (8th Edition)
Computer Science
ISBN:
9780133976892
Author:
Paul J. Deitel, Harvey Deitel
Publisher:
PEARSON
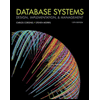
Database Systems: Design, Implementation, & Manag…
Computer Science
ISBN:
9781337627900
Author:
Carlos Coronel, Steven Morris
Publisher:
Cengage Learning
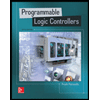
Programmable Logic Controllers
Computer Science
ISBN:
9780073373843
Author:
Frank D. Petruzella
Publisher:
McGraw-Hill Education