Suppose that, for some N, the continuous random variables X₁, X2,..., XN are independently drawn from the uniform distribution on [-1,1]. That is, each X₂ Uniform([-1,1]). Let S = X₁ + X2 + ... + XN denote the sum of these uniform random variables. The Central Limit Theorem allows us to approximate the true sum Sy as a Gaussian random variable we'll call G. (It is up to you to find the mean and variance of G.) Notice that since each X; is bounded, Sy is also bounded. In particular, the true sum Sy can only take values between -N and N. However, the Gaussian approximation to Sy is not bounded: G has some small probability of taking values greater than N (or less than -N). Find an expression for P(|G| > N). Your answer should be in terms of N, and it should involve the Gaussian cdf function Þ(.). Hint: for large values of N, P(|G| > N) goes to 0.
Suppose that, for some N, the continuous random variables X₁, X2,..., XN are independently drawn from the uniform distribution on [-1,1]. That is, each X₂ Uniform([-1,1]). Let S = X₁ + X2 + ... + XN denote the sum of these uniform random variables. The Central Limit Theorem allows us to approximate the true sum Sy as a Gaussian random variable we'll call G. (It is up to you to find the mean and variance of G.) Notice that since each X; is bounded, Sy is also bounded. In particular, the true sum Sy can only take values between -N and N. However, the Gaussian approximation to Sy is not bounded: G has some small probability of taking values greater than N (or less than -N). Find an expression for P(|G| > N). Your answer should be in terms of N, and it should involve the Gaussian cdf function Þ(.). Hint: for large values of N, P(|G| > N) goes to 0.
A First Course in Probability (10th Edition)
10th Edition
ISBN:9780134753119
Author:Sheldon Ross
Publisher:Sheldon Ross
Chapter1: Combinatorial Analysis
Section: Chapter Questions
Problem 1.1P: a. How many different 7-place license plates are possible if the first 2 places are for letters and...
Related questions
Question
Please assist with this practice problem
![Suppose that, for some \( N \), the continuous random variables \( X_1, X_2, \ldots, X_N \) are independently drawn from the uniform distribution on \([-1,1]\). That is, each \( X_i \sim \text{Uniform}([-1,1]) \). Let \( S_N = X_1 + X_2 + \cdots + X_N \) denote the sum of these uniform random variables.
The Central Limit Theorem allows us to approximate the true sum \( S_N \) as a Gaussian random variable we’ll call \( G \). (It is up to you to find the mean and variance of \( G \).)
Notice that since each \( X_i \) is bounded, \( S_N \) is also bounded. In particular, the true sum \( S_N \) can only take values between \(-N\) and \( N \). However, the Gaussian approximation to \( S_N \) is not bounded: \( G \) has some small probability of taking values greater than \( N \) (or less than \(-N\)).
Find an expression for \( P(|G| > N) \). Your answer should be in terms of \( N \), and it should involve the Gaussian cdf function \( \Phi(\cdot) \). Hint: for large values of \( N \), \( P(|G| > N) \) goes to 0.](/v2/_next/image?url=https%3A%2F%2Fcontent.bartleby.com%2Fqna-images%2Fquestion%2Fc012e98d-11f3-4ebf-9e91-31a87ace6c91%2Fe6b29708-5ded-498e-909c-d72971f5bc70%2Fcr6m4_processed.png&w=3840&q=75)
Transcribed Image Text:Suppose that, for some \( N \), the continuous random variables \( X_1, X_2, \ldots, X_N \) are independently drawn from the uniform distribution on \([-1,1]\). That is, each \( X_i \sim \text{Uniform}([-1,1]) \). Let \( S_N = X_1 + X_2 + \cdots + X_N \) denote the sum of these uniform random variables.
The Central Limit Theorem allows us to approximate the true sum \( S_N \) as a Gaussian random variable we’ll call \( G \). (It is up to you to find the mean and variance of \( G \).)
Notice that since each \( X_i \) is bounded, \( S_N \) is also bounded. In particular, the true sum \( S_N \) can only take values between \(-N\) and \( N \). However, the Gaussian approximation to \( S_N \) is not bounded: \( G \) has some small probability of taking values greater than \( N \) (or less than \(-N\)).
Find an expression for \( P(|G| > N) \). Your answer should be in terms of \( N \), and it should involve the Gaussian cdf function \( \Phi(\cdot) \). Hint: for large values of \( N \), \( P(|G| > N) \) goes to 0.
Expert Solution

This question has been solved!
Explore an expertly crafted, step-by-step solution for a thorough understanding of key concepts.
Step by step
Solved in 3 steps with 13 images

Recommended textbooks for you
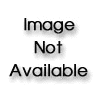
A First Course in Probability (10th Edition)
Probability
ISBN:
9780134753119
Author:
Sheldon Ross
Publisher:
PEARSON
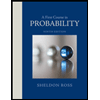
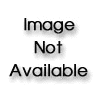
A First Course in Probability (10th Edition)
Probability
ISBN:
9780134753119
Author:
Sheldon Ross
Publisher:
PEARSON
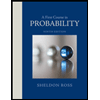