Read the pages and make a very brief summary of them with your own words, please. It is what you understand. Don't make copy-paste, please. Mention important parts only. Also, you will put your comments and ideas about the topic. Please don't write item by item. Write the summary in paragraph form.
Read the pages and make a very brief summary of them with your own words, please. It is what you understand. Don't make copy-paste, please. Mention important parts only. Also, you will put your comments and ideas about the topic. Please don't write item by item. Write the summary in paragraph form.
Social Psychology (10th Edition)
10th Edition
ISBN:9780134641287
Author:Elliot Aronson, Timothy D. Wilson, Robin M. Akert, Samuel R. Sommers
Publisher:Elliot Aronson, Timothy D. Wilson, Robin M. Akert, Samuel R. Sommers
Chapter1: Introducing Social Psychology
Section: Chapter Questions
Problem 1RQ1
Related questions
Question
100%
Read the pages and make a very brief summary of them with your own words, please. It is what you understand. Don't make copy-paste, please. Mention important parts only. Also, you will put your comments and ideas about the topic. Please don't write item by item. Write the summary in paragraph form.

Transcribed Image Text:UCL or LCL, or there will be a "run" of several points in a
row above or below the average line. When a penetration or
a lengthy run appears, this is the control chart's signal that
other tools is that it does not help us understand whether something is wrong that requires immediate attention.
As long as the plots stay between the limits and don't
congregate on one side or the other of the process average
line, the process is in statistical control. If either of these con-
ditions is not met, then we can say that the process is not
in statistical control or simply is "out of control"-hence the
Control Charts
4
The problem with the run chart and, in fact, many of the
the variation is the result of special causes-things such as
changes in the materials used, machine problems, lack of
employee training-or common causes that are purely ran-
dom. Not until Dr. Walter Shewhart made that distinction
1.
7
9.
11
13 15
17 19 21
Workday
in the 1920s was there a real chance of improving processes
through the use of statistical techniques. Shewhart, then an
employee of Bell Laboratories, developed the control chart
to separate the special causes from the common causes.
In evaluating problems and finding solutions for them, it
is important to distinguish between special causes and com-
mon causes. Figure 15.27 shows a typical control chart. Data
are plotted over time, just as with a run chart; the difference
is that the data stay between the upper control limit (UCL)
and the lower control limit (LCL) while varying about the
centerline or average only so long as the variation is the re-
sult of common causes (i.e., statistical variation). Whenever a
special cause (nonstatistical cause) impacts the process, one
of two things will happen: Either a plot point will penetrate
name of the chart.
If you understand that it is the UCL, LCL, and process
average lines added to the run chart that make the differ-
ence, you may wonder how those lines are set. The position-
ing of the lines cannot be arbitrary. Nor can they merely
reflect what you want out of the process, for example, based
on a specification. Such an approach won't help separate
common causes from special causes, and it will only compli-
cate attempts at process improvement. UCL, LCL, and pro-
cess average must be determined by valid statistical means.
Determination of UCL, LCL, and process average is fully
covered in Chapter 18, which is dedicated to the use of con-
trol charts in statistical process control.
All processes have built-in variability. A process that
is in statistical control will still be affected by its natural
random variability. Such a process will exhibit the normal
distribution of the bell curve. The more finely tuned the
process, the less deviation from the process average and the
narrower the bell curve. (Refer to Figure 15.19, Histogram
A and Histogram B.) This is at the heart of the control chart
and is what makes it possible to define the limits and process
FIGURE 15.28 Chart of Operator Defects for November.
might include data relative to the environment, the people
involved, the machine(s) used in the process, materials, and
so on. Grouping data by common element or characteristic
makes it easier to understand the data and to pull insights
from them.
Consider an example from a factory floor. One of the
factory's products requires five assemblers, all doing the
same thing at the same rate. Their output flows together
for inspection. Inspection has found an unacceptably high
rate of defects in the products. Management forms a team
to investigate the problem with the objective of finding the
cause and correcting it. They plot the data taken over the last
month (see Figure 15.28).
The chart in Figure 15.28 plots all operator-induced de-
fects for the month. The team believes that for this product,
zero defects can be approached. If you were going to react to
this chart alone, how would you deal with the problem? You
have five assemblers. Do they all contribute defects equally?
This is hardly ever the case. The data can be stratified by the
operator to determine each individual's defect performance.
The charts in Figure 15.29 do this.
The five stratified charts in Figure 15.29 indicate that
one operator, Assembler B, is responsible for more defects
than the other four combined. Assembler A also makes more
UCL
average.
Control charts are the appropriate tool to monitor pro-
cesses. The properly used control chart will immediately
alert the operator to any change in the process. The appro-
priate response to that alert is to stop the process at once,
preventing the production of defective product. Only after
the special cause of the problem has been identified and cor-
rected should the process be restarted. Having eliminated
a problem's root cause, that problem should never recur.
(Anything less, however, and it is sure to return eventu-
ally.) Control charts also enable continual improvement of
processes. When a change is introduced to a process that is
operated under statistical process control charts, the effect
of the change will be immediately seen. You know when you
have made an improvement. You also know when the change
is ineffective or even detrimental. This validates effective
improvements, which you will retain. This is enormously
difficult when the process is not in statistical control because
the process instability masks
changes deliberately made.
To learn more about statistical process control and con-
trol charts, study Chapter 18.
Process Average
LCL
Samples
than twice as many errors as Assembler C or Assembler D
and eight times as many as Assembler E, the best performer
of the group.
FIGURE 15.27 Basic Control Chart.
The performance of Assembler A and Assembler B must
be brought up to the level of the others. Possible causes of the
operator-induced defects could be inherent skill, training,
vision, attitude, attentiveness, and environmental factors,
such as noise, lighting, and temperature in the operator's
workstation area. The charts provide an indication of the
place to start making changes.
The Pareto charts of Figure 15.6 also represent stratifi-
cation. Figure 15.6 started with a series of defect types that
results, good or bad, of any
were
most costly (the first chart). Then it took the worst
case, Miswires, and divided it into the kinds of miswires
(the second chart). Then the worst kind, Hand Wrap, was
split into several categories (the third chart). The dominant
Hand-Wrap defect category was operator induced. Finally,
the Operator category was stratified by individual operator
(the fourth chart).
The power of stratification lies in the fact that if you
stratify far enough, you will arrive at a root cause of the
STRATIFICATION
Stratification is a simple tool in spite of its name. It involves
investigating the cause of a problem by grouping data into
categories. This grouping is called stratification. The groups
Defects
Expert Solution

This question has been solved!
Explore an expertly crafted, step-by-step solution for a thorough understanding of key concepts.
Step by step
Solved in 2 steps

Recommended textbooks for you
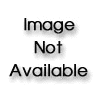
Social Psychology (10th Edition)
Sociology
ISBN:
9780134641287
Author:
Elliot Aronson, Timothy D. Wilson, Robin M. Akert, Samuel R. Sommers
Publisher:
Pearson College Div
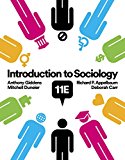
Introduction to Sociology (Eleventh Edition)
Sociology
ISBN:
9780393639407
Author:
Deborah Carr, Anthony Giddens, Mitchell Duneier, Richard P. Appelbaum
Publisher:
W. W. Norton & Company
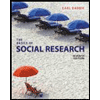
The Basics of Social Research (MindTap Course Lis…
Sociology
ISBN:
9781305503076
Author:
Earl R. Babbie
Publisher:
Cengage Learning
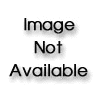
Social Psychology (10th Edition)
Sociology
ISBN:
9780134641287
Author:
Elliot Aronson, Timothy D. Wilson, Robin M. Akert, Samuel R. Sommers
Publisher:
Pearson College Div
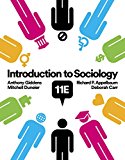
Introduction to Sociology (Eleventh Edition)
Sociology
ISBN:
9780393639407
Author:
Deborah Carr, Anthony Giddens, Mitchell Duneier, Richard P. Appelbaum
Publisher:
W. W. Norton & Company
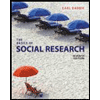
The Basics of Social Research (MindTap Course Lis…
Sociology
ISBN:
9781305503076
Author:
Earl R. Babbie
Publisher:
Cengage Learning
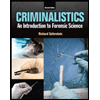
Criminalistics: An Introduction to Forensic Scien…
Sociology
ISBN:
9780134477596
Author:
Saferstein, Richard
Publisher:
PEARSON
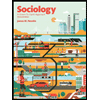
Sociology: A Down-to-Earth Approach (13th Edition)
Sociology
ISBN:
9780134205571
Author:
James M. Henslin
Publisher:
PEARSON
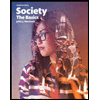
Society: The Basics (14th Edition)
Sociology
ISBN:
9780134206325
Author:
John J. Macionis
Publisher:
PEARSON