Question You can actually improve model fit by removing (a) predictor(s) in multiple regression. True False
Question
You can actually improve model fit by removing (a) predictor(s) in multiple regression.
True
False
Question 2
...................? are observations that fall far from the "cloud" of points
Question 3
What is the best way to describe the point in the yellow box?
a. An outlier fall along from the cloud that appears to influence the direction of the line
b. An outlier close to the least squares line, suggesting that it was likely not very influential
c. An outlier far from the other points that only slightly influences the line, if at all
d. None of these
Question 4
The proper estimate of variance explained in multiple regression is
a. >50% significant predictors
b. Adjusted R2
c. R2
d. All of these
Question 5
Adjusted R2 is actually a biased estimate, so we turn to R2
True
False
Question 6
In multiple regression, you can have nominal and continuous predictors
True
False
Question 7
In this regression, which predictor(s) is/are significant?
a. Both
b. 'pubs'
c. Neither
d. 'time'
Question 8
You run a regression for x predicting y and find the p-value to be .04. Which of the following can you say most comfortably?
a. Given the assumptions have been met, y significantly predicts x, but we can't be sure if it is important
b. None of these
c. Only 4% of the time would you expect to find a test statistic as extreme or more, assuming the null hypothesis is true
d. The finding indicates x strongly predicts y
Question 9
Which of the following describes the amount of variation in the response that is explained by the least squares line?
a. S
b. Line of best fit
c. Variability
d. R2
Question 10
Which of the following is true?
a. The t-value was obtained by dividing the estimate by standard error
b. The 'unemp' coefficient is significant
c. A t-value of -1.23 is significant
d. None of these
Question 11
Based on this table, which of the following statements is true?
a. adverts' estimate of .08 shows it is not significant
b. adverts' t-value of -1.53 shows it is a significant predictor
c. adverts' t-value and associated p-value indicate it is a significant predictor
d. None of these
Question 12
The line of best fit in linear regression will be the one that has the maximizes the sum of the squared residuals
True
False
Question 13
Which of the following are assumptions when fitting a least squares line in linear regression? (Select all that apply)
a. Linearity: The data should show a linear trend
b. Nearly normal residuals: generally the residuals must be nearly normal
c. Constant variability: Variability of points around the least squares line must remain roughly constant
d. Symmetry: Lines of best fit should fit symmetrically along the
Question 14
When fitting a line to data, the points will (almost) never perfectly fall on the line. The difference between the line of best fit and a point is called a(n)
a. error
b. residual
c. regressor
d.
Question 15 (2 points)
Just by looking at this
a. No relationship
b. Parabolic
c. Negative
d. Positive
Question 16
Logistic and multiple regression have the same type of outcome variables
True
False
Question 17
What transformation does this represent?
log (p)
(1-p)

Trending now
This is a popular solution!
Step by step
Solved in 2 steps

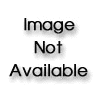
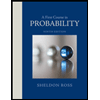
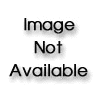
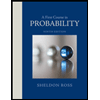