QUESTION: (PYTHON PROGRAMMING) Edit the code to reduce the black points ( black noise ).
import numpy as np
import matplotlib.pyplot as plt
from sklearn.cluster import KMeans
from sklearn.cluster import DBSCAN
from sklearn.datasets import make_blobs
n_samples = [750,750,750]
cluster_std = 1
random_state = 200
X, Y_true = make_blobs(n_samples=n_samples, random_state=random_state, cluster_std=cluster_std)
plt.scatter(X[:, 0], X[:, 1], marker='.', c=Y_true)
#DBSCAN model, setting up required parameters
eps = 0.4
min_Samples = 10
db = DBSCAN(eps=eps, min_samples=min_Samples).fit(X)
labels = db.labels_
labels
# To count number of clusters in labels, ignoring noise if present.
n_clusters_ = len(set(labels)) - (1 if -1 in labels else 0)
n_noise_ = list(labels).count(-1)
print('Estimated number of clusters: %d' % n_clusters_)
print('Estimated number of noise points: %d' % n_noise_)
# Plot result
# Use black to label noise points.
unique_labels = set(labels)
colors = plt.cm.Spectral(np.linspace(0, 1, len(unique_labels)))
core_samples_mask = np.zeros_like(db.labels_, dtype=bool)
core_samples_mask[db.core_sample_indices_] = True
# Plot the points with colors
for k, col in zip(unique_labels, colors):
if k == -1:
# Black used for noise points.
col = 'k'
class_member_mask = (labels == k)
# Plot the datapoints that are clustered
xy = X[class_member_mask & core_samples_mask]
plt.scatter(xy[:, 0], xy[:, 1], c=[col], marker=u'o', alpha=0.5)
# Plot the border points and noise points
xy = X[class_member_mask & ~core_samples_mask]
plt.scatter(xy[:, 0], xy[:, 1], c=[col], marker=u'x', alpha=0.5)'
QUESTION: (PYTHON PROGRAMMING)
Edit the code to reduce the black points ( black noise ).
TIP: adjust eps and min_Samples
https://ibb.co/b2jwzSy

Trending now
This is a popular solution!
Step by step
Solved in 2 steps with 1 images

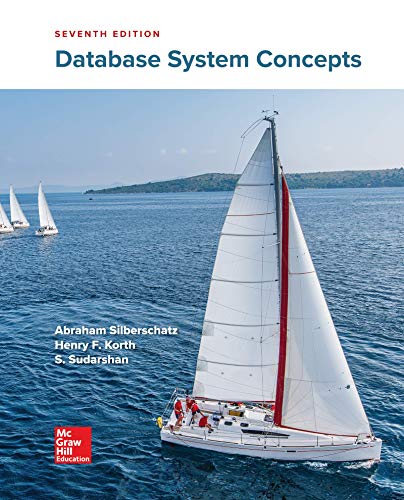
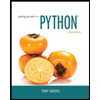
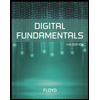
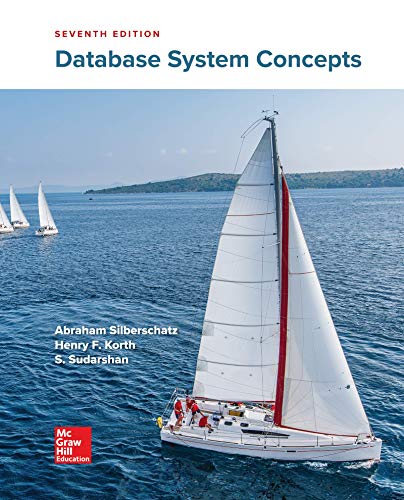
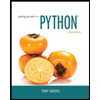
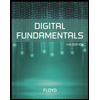
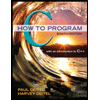
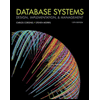
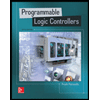