QUESTION 6 Suppose we are to make a two-class classification decision (class label are w₁ and w2) based on observations of a scalar random variable x . Suppose we collect N such observations, x,, i = 1,, N and suppose they are independent and identically distributed (i.i.d.) with P(w1) and P(w2). What is the general expression, given in class, for the minimum-error-rate decision rule? O O ^(x)= A(x)= A(x)= ^(x)= p(x/w₂) > d₂ p(x/a)₂ 1 p(x/w₂) > d₂ P(W₁) p(x/w₁) <₂ P(W₂) p(x/w₂) > d₂ (112-122) P(W₁) p(x/w₁) ₂ (112) P(W₁) p(x/w₁)
QUESTION 6 Suppose we are to make a two-class classification decision (class label are w₁ and w2) based on observations of a scalar random variable x . Suppose we collect N such observations, x,, i = 1,, N and suppose they are independent and identically distributed (i.i.d.) with P(w1) and P(w2). What is the general expression, given in class, for the minimum-error-rate decision rule? O O ^(x)= A(x)= A(x)= ^(x)= p(x/w₂) > d₂ p(x/a)₂ 1 p(x/w₂) > d₂ P(W₁) p(x/w₁) <₂ P(W₂) p(x/w₂) > d₂ (112-122) P(W₁) p(x/w₁) ₂ (112) P(W₁) p(x/w₁)
Power System Analysis and Design (MindTap Course List)
6th Edition
ISBN:9781305632134
Author:J. Duncan Glover, Thomas Overbye, Mulukutla S. Sarma
Publisher:J. Duncan Glover, Thomas Overbye, Mulukutla S. Sarma
Chapter6: Power Flows
Section: Chapter Questions
Problem 6.22P
Related questions
Question
Show all work please!

Transcribed Image Text:**Question 6**
Suppose we are to make a two-class classification decision (class labels are \( w_1 \) and \( w_2 \)) based on observations of a scalar random variable \( x \). Suppose we collect \( N \) such observations, \( x_i, i = 1, \ldots, N \), and suppose they are independent and identically distributed (i.i.d.) with \( P(w_1) \) and \( P(w_2) \).
What is the general expression, given in class, for the minimum-error-rate decision rule?
Choices:
- \(\Lambda(x) = \frac{p(x/ w_2)}{p(x/ w_1)} \begin{cases} > \frac{d_2}{d_1} \\ < 1 \end{cases}\)
- \(\Lambda(x) = \frac{p(x/ w_2)}{p(x/ w_1)} \begin{cases} > \frac{d_2}{d_1} \frac{P(w_1)}{P(w_2)} \\ < \frac{P(w_1)}{P(w_2)} \end{cases}\)
- \(\Lambda(x) = \frac{p(x/ w_2)}{p(x/ w_1)} \begin{cases} > \frac{d_2}{d_1} ( \lambda_{12} - \lambda_{22} ) \frac{P(w_1)}{P(w_2)} \\ < ( \lambda_{21} - \lambda_{11} ) \frac{P(w_1)}{P(w_2)} \end{cases}\)
- \(\Lambda(x) = \frac{p(x/ w_2)}{p(x/ w_1)} \begin{cases} > \frac{d_2}{d_1} \frac{ \lambda_{12} }{ \lambda_{22} } \frac{P(w_1)}{P(w_2)} \\ < \frac{\lambda_{21}}{\lambda_{22}} \frac{P(w_1)}{P(w_2)} \end{cases}\)
**Correct answer: b)**
Expert Solution

Step 1
Trending now
This is a popular solution!
Step by step
Solved in 2 steps with 2 images

Knowledge Booster
Learn more about
Need a deep-dive on the concept behind this application? Look no further. Learn more about this topic, electrical-engineering and related others by exploring similar questions and additional content below.Recommended textbooks for you
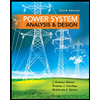
Power System Analysis and Design (MindTap Course …
Electrical Engineering
ISBN:
9781305632134
Author:
J. Duncan Glover, Thomas Overbye, Mulukutla S. Sarma
Publisher:
Cengage Learning
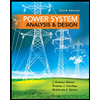
Power System Analysis and Design (MindTap Course …
Electrical Engineering
ISBN:
9781305632134
Author:
J. Duncan Glover, Thomas Overbye, Mulukutla S. Sarma
Publisher:
Cengage Learning