Question 5 We have observed data y = {yij: i= 1,...,n, j = 1,...,m;}. Each yi; is the number of times a certain type of machine needs to be repaired during length of time Tij, where j = 1,...,m; are the machines in factory i, for i = 1,...,n, with n ≥ 2. A hierarchical model is used to model the data. We assume that Yij Poisson (TijMi). μi is the repair rate for factory i, which varies between factories according to a gamma distribution Mi~ Gamma (a,ß), i = 1,..., n. The parameters a and 3 are given prior distributions, p(a) and p(B). Suppose that we have generated a sample of size M from the joint posterior distribution p1,...,n, a, ß y). (a) Explain how to estimate the following using the joint posterior sample: (i) The posterior mean of a. a (ii) The posterior median of v = B (iii) A 95% equal tail credible interval for v. (b) Explain how to generate a sample from the posterior predictive distribution of the number of repairs during time U for a machine not in our dataset, in each of the following two cases: (i) If the factory containing this machine is in our dataset. (ii) If the factory is not in our dataset. Also explain how to estimate the posterior predictive probability that such a machine will not need any repairs during time U.
Question 5 We have observed data y = {yij: i= 1,...,n, j = 1,...,m;}. Each yi; is the number of times a certain type of machine needs to be repaired during length of time Tij, where j = 1,...,m; are the machines in factory i, for i = 1,...,n, with n ≥ 2. A hierarchical model is used to model the data. We assume that Yij Poisson (TijMi). μi is the repair rate for factory i, which varies between factories according to a gamma distribution Mi~ Gamma (a,ß), i = 1,..., n. The parameters a and 3 are given prior distributions, p(a) and p(B). Suppose that we have generated a sample of size M from the joint posterior distribution p1,...,n, a, ß y). (a) Explain how to estimate the following using the joint posterior sample: (i) The posterior mean of a. a (ii) The posterior median of v = B (iii) A 95% equal tail credible interval for v. (b) Explain how to generate a sample from the posterior predictive distribution of the number of repairs during time U for a machine not in our dataset, in each of the following two cases: (i) If the factory containing this machine is in our dataset. (ii) If the factory is not in our dataset. Also explain how to estimate the posterior predictive probability that such a machine will not need any repairs during time U.
MATLAB: An Introduction with Applications
6th Edition
ISBN:9781119256830
Author:Amos Gilat
Publisher:Amos Gilat
Chapter1: Starting With Matlab
Section: Chapter Questions
Problem 1P
Related questions
Question
Can you answer all parts please ?

Transcribed Image Text:Question 5
We have observed data
y = {yij: i= 1,...,n, j = 1,...,m;}.
Each yi; is the number of times a certain type of machine needs to be repaired during length of
time Tij, where j = 1,...,m; are the machines in factory i, for i = 1,...,n, with n ≥ 2.
A hierarchical model is used to model the data. We assume that
Yij Poisson (TijMi).
μi is the repair rate for factory i, which varies between factories according to a gamma
distribution
Mi~ Gamma (a,ß), i = 1,..., n.
The parameters a and 3 are given prior distributions, p(a) and p(B).
Suppose that we have generated a sample of size M from the joint posterior distribution
p1,...,n, a, ß y).
(a) Explain how to estimate the following using the joint posterior sample:
(i) The posterior mean of a.
a
(ii) The posterior median of v =
B
(iii) A 95% equal tail credible interval for v.
(b) Explain how to generate a sample from the posterior predictive distribution of the
number of repairs during time U for a machine not in our dataset, in each of the
following two cases:
(i) If the factory containing this machine is in our dataset.
(ii) If the factory is not in our dataset. Also explain how to estimate the posterior
predictive probability that such a machine will not need any repairs during time U.
AI-Generated Solution
Unlock instant AI solutions
Tap the button
to generate a solution
Recommended textbooks for you
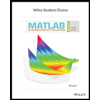
MATLAB: An Introduction with Applications
Statistics
ISBN:
9781119256830
Author:
Amos Gilat
Publisher:
John Wiley & Sons Inc
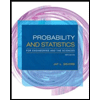
Probability and Statistics for Engineering and th…
Statistics
ISBN:
9781305251809
Author:
Jay L. Devore
Publisher:
Cengage Learning
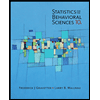
Statistics for The Behavioral Sciences (MindTap C…
Statistics
ISBN:
9781305504912
Author:
Frederick J Gravetter, Larry B. Wallnau
Publisher:
Cengage Learning
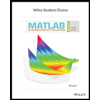
MATLAB: An Introduction with Applications
Statistics
ISBN:
9781119256830
Author:
Amos Gilat
Publisher:
John Wiley & Sons Inc
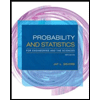
Probability and Statistics for Engineering and th…
Statistics
ISBN:
9781305251809
Author:
Jay L. Devore
Publisher:
Cengage Learning
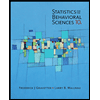
Statistics for The Behavioral Sciences (MindTap C…
Statistics
ISBN:
9781305504912
Author:
Frederick J Gravetter, Larry B. Wallnau
Publisher:
Cengage Learning
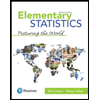
Elementary Statistics: Picturing the World (7th E…
Statistics
ISBN:
9780134683416
Author:
Ron Larson, Betsy Farber
Publisher:
PEARSON
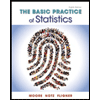
The Basic Practice of Statistics
Statistics
ISBN:
9781319042578
Author:
David S. Moore, William I. Notz, Michael A. Fligner
Publisher:
W. H. Freeman
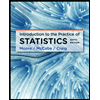
Introduction to the Practice of Statistics
Statistics
ISBN:
9781319013387
Author:
David S. Moore, George P. McCabe, Bruce A. Craig
Publisher:
W. H. Freeman